Effects of online service failure on customers’ intentions to complain online
Main Article Content
Dissatisfied customers are increasingly voicing complaints through social media following online service failures; therefore, it is important to clarify the motivational determinants of customers’ online complaint intentions (COCI). We investigated in 3 studies the influence and interaction effects of service failure types, attributions about these failures, scope of impact of the failures, and customer inoculation on both public and private online complaint intentions. Participants were 451 college students from Hainan Province, China. The results show that service failure types, service failure attributions, scope of impact of the service failure, and customer inoculation each had distinct effects on COCI and how customers complain online, and that these factors also had interactive effects on online complaint actions. Our finding that the form of COCI can predict service failure attributes offers implications for the implementation of enterprise service recovery from a consumer perspective.
The emergence of the Internet has given rise to a growing number of complaint websites where customers can share their experiences (Harrison-Walker, 2001). According to a report published by the E-Commerce Research Center (2018), the number of complaints received via social media rose by 66.93% during the first half of 2018. Research has shown that consumers’ complaint intentions regarding physical shopping can be influenced by their attitudes (Andreassen & Streukens, 2013), brand commitment (Weitzl & Hutzinger, 2019), perceived behavioral control (Chang & Chin, 2011), and online service failure type (Jian & Ke, 2017). However, online service failure incidents differ substantially from those occurring in-person. Few studies have investigated the factors that influence customers’ online complaint intentions (COCI). Therefore, exploring the factors that affect COCI and the internal mechanisms of this variable carries great theoretical and practical significance.
Literature Review and Development of Hypotheses
As suggested by Hsiao (2011), we classified customers’ online complaint behaviors into public and private action types. Public online complaint intentions (PUOC) refer to the process through which customers rebel against firms or third parties and express a desire to seek revenge online, whereas private online complaint intentions (PVOC) relate to sharing negative feedback about commodities with friends or relatives online (Hsiao, 2011). We focused in this study on the effects of service failure types, attributions about these failures, the scope of impact of service failure (individual vs. group), and customer inoculation on PUOC and PVOC.
Research has indicated that distinct types of service failure influence consumer satisfaction differently, with outcome failures in a service encounter relating to what customers actually receive from the service, whereas process failures are related to how customers receive the service, that is, the manner in which it is delivered (Smith et al., 1999). For instance, people tend to be more dissatisfied with process failures than outcome failures with respect to hotel services (Smith et al., 1999). In contrast, in the UK banking industry, only once a product or service has met a customer’s expectations do process factors influence consumer satisfaction (Johnston, 1995). Although studies of service marketing have not highlighted which types of service failure are most influential on consumers’ perceptions and satisfaction, scholars have found that the effects of service failure on customer behavior differ according to whether it is a process or outcome failure (Zhu et al., 2004). Although a few researchers have tested the impact of service failure types on customer behavior, the relationship between these two variables in the online domain has yet to be explored.
The concept of service failure attribution has garnered attention in customer complaint research, with Heider (1958) dividing this into internal and external attribution types: Internal attribution suggests that the reason for service failure lies with the corporation itself, whereas external attribution places responsibility outside the corporate environment. Iglesias (2009) examined a sample of 293 service encounters and noted that customers expressed less satisfaction when service failure was attributed to internal rather than external factors. Song and Wang (2005), Swanson and Kelley (2001), and Weitzl et al. (2018) also found that the more strongly customers attributed a service failure to internal factors, the more dissatisfied they tended to be, which increased consumers’ likelihood of spreading negative information. Therefore, in this study we tested the effects of service failure attributions on customer complaints online, and investigated whether an interactional influence exists between service failure types and attributions on COCI.
Researchers have also shown that the size of the group affected by service failure influences customer complaints. In this context, group service failure refers to more than two customers encountering the same service failure event simultaneously; individual service failure refers to one customer facing a failure within a service process (Du & Fan, 2011). Previous studies have revealed that individual members of a group can experience an emotional burden (i.e., feeling responsible for carrying an emotion in the name of the group) when their feelings are inconsistent with those of the collective (Chen & Zhang 2014; Goldenberg et al., 2014). Emotional transfer, which occurs when one’s negative feelings toward the in-group are transferred to the event itself, is also a potential outcome for individuals within a group (Chen & Zhang 2014; Goldenberg et al., 2014). Using the concept of emotional contagion and group dynamic theory, scholars have further shown that the effects of group service failure and individual service failure uniquely shape customers’ complaint emotions and behavior based on individual interactions (Du et al., 2014). However, the behavioral patterns and psychological mechanisms influencing individuals in groups are yet to be considered in online shopping situations. Therefore, we examined the scope of impact of a service failure in an online shopping context, under the assumption that group service failure and individual service failure would exert distinct influences on COCI.
As a preemptive service recovery strategy, customer inoculation occurs when customers are informed of a service failure before encountering it, thereby minimizing dissatisfaction (Papageorgis & McGuire, 1961). Inoculation theory suggests that with preexposure to negative information, customers’ subconscious minds prompt them to generate supporting arguments to resist counterarguments presented later (Mikolon et al., 2015; Papageorgis & McGuire, 1961). For instance, customers who are told they must wait roughly 30 minutes before dining should evaluate the service less negatively than do those who are not informed in advance of the estimated wait time. Being informed of a potential problem in advance enables customers to adapt to failure and avoid modifying their existing attitudes when confronted with actual negative information. Studies have indicated that customer inoculation causes individuals to be more resistant to subsequent attacks and sharp declines in satisfaction (Einwiller & Johar, 2013; Mikolon et al., 2015). Therefore, we anticipated that customer inoculation would influence customers’ emotions and COCI.
In summary, we performed three 2 × 2 mixed design experiments to explore the interaction effects of service failure type along with the influencing factors of service failure attribution, scope of impact, and customer inoculation on consumers’ PUOC and PVOC. These suppositions led to us forming the following hypotheses:
Hypothesis 1a: Service failure type (process vs. outcome) will influence customers’ public online complaint intentions.
Hypothesis 1b: Service failure type (process vs. outcome) will influence customers’ private online complaint intentions.
Hypothesis 2a: Service failure attributions (internal vs. external) will influence customers’ public online complaint intentions.
Hypothesis 2b: Service failure attributions (internal vs. external) will influence customers’ private online complaint intentions.
Hypothesis 3a: The scope of impact (individual vs. group) of a service failure will influence customers’ public online complaint intentions.
Hypothesis 3b: The scope of impact (individual vs. group) of a service failure will influence customers’ private online complaint intentions.
Hypothesis 4a: Customer inoculation will influence customers’ public online complaint intentions.
Hypothesis 4b: Customer inoculation will influence customers’ private online complaint intentions.
Study 1: Influences of Service Failure Types and Attributions on Customers’ Online Complaint Intentions
Method
Study 1 had a 2 (service failure type: process vs. outcome) × 2 (attribution type: internal vs. external) mixed design. Participants were randomly separated into four groups, each of which encountered a different scenario. All scenarios simulated a real episode from a consumer complaint website, with slight modifications made between groups. Participants were told to imagine they were purchasing a pair of shoes as a gift; service failure and attribution types were manipulated. Our goals were to test (a) whether different service failure types and attributions had unique effects on customers’ PUOC and PVOC, and (b) whether an interaction effect existed between service failure and attribution types on customers’ PUOC and PVOC.
Participants
We recruited 149 college students from a university in Hainan Province, China. After discarding cases with a large amount of missing data, 136 samples were deemed useable (valid response rate = 91.3%). There were 65 women and 71 men (Mage = 19.71 years, SD = 1.99, range = 17–24) All participants provided informed consent, took part in the study voluntarily, and received compensation of CNY 10 (approximately USD 1.45).
Measure
COCI was measured using six items adopted from Hsiao (2011), which were divided across two dimensions: “I will speak to my friends and relatives via the Internet about my bad experience” (PVOC), “I will convince my friends and relatives via the Internet not to use this product/service” (PVOC), “I will use the Internet to warn all the people I know against buying this product/service” (PVOC), “I will seek redress for the service failure directly from company’s website” (PUOC), “I will report this service failure to the consumer agency or other websites via the Internet” (PUOC), and “I will complain about the service failure to a private or government agency via the Internet” (PUOC). All items were measured on a 5-point Likert-type scale (1 = very unlikely, 5 = very likely). Cronbach’s alpha values for the PVOC and PUOC item subscales were .79 and .80, respectively.
Procedure
First, participants arrived at the laboratory and were divided randomly into four groups: outcome failure × internal attribution, outcome failure × external attribution, process failure × internal attribution, and process failure × external attribution. Then, each group viewed a different PowerPoint presentation designed to simulate a real shopping scenario. Next, each group completed the COCI measure. Study results were recorded and quantified after participants completed the questionnaire.
Results
Control and Manipulation Checks
As a control check, participants responded to the following three questions: “Have you ever had an online shopping experience?” (1 = very few, 5 = many), “Can you imagine yourself as a customer in this instance?” (1 = very hard to do so, 5 = very easy to do so), and “If this happened to you, how serious would you think it is?” (1 = not very serious, 5 = very serious). Findings indicate that the depicted scenarios were effectively controlled for: online shopping experience (M = 4.28, SD = 0.61), t(135) = 24.67, p < .001, perceived realism (M = 4.41, SD = 0.58), t(135) = 28.54, p < .001, and service failure severity (M = 4.39, SD = 0.57), t(135) = 28.28, p < .001.
Manipulation checks spanned two parts of the study. The first part, which comprised the question “If the service process of purchasing is divided into three stages: prepurchase, purchase, and postpurchase, which stage of service has failed according to you?”, was intended to verify whether the manipulation of service failure types was successful. Participants rated the degree to which each stage had failed based on a 5-point Likert-type scale (1 = do not agree at all, 5 = agree very much). The manipulation check results in Table 1 reveal that service failure types were manipulated effectively.
Table 1. Manipulation Check Results for Service Failure Type

The second part of the manipulation comprised the questions “Do you think the service failure occurred because of a fault in the online store itself?” and “Do you think the service failure occurred because of factors external to the online store itself?”, which were rated on a 5-point Likert-type scale (1 = not at all, 5 = absolutely). The results in Table 2 show that the manipulation of attribution types was successful.
Table 2. Manipulation Check Results for Service Failure Attribution

Effects of Service Failure and Attribution Types on Customers’ Public Online Complaint Intentions
We observed an interaction effect between service failure and attribution on customers’ PUOC on the basis of results of a multivariate analysis of variance (see Figure 1). Results indicate that the main effect of service failure type on customers’ PUOC was significant, F(1, 134) = 100.48, p < .001, η2p = .43, as was the main effect of attribution on customers’ PUOC, F(1, 134) = 359.26, p < .001, η2p = .73.
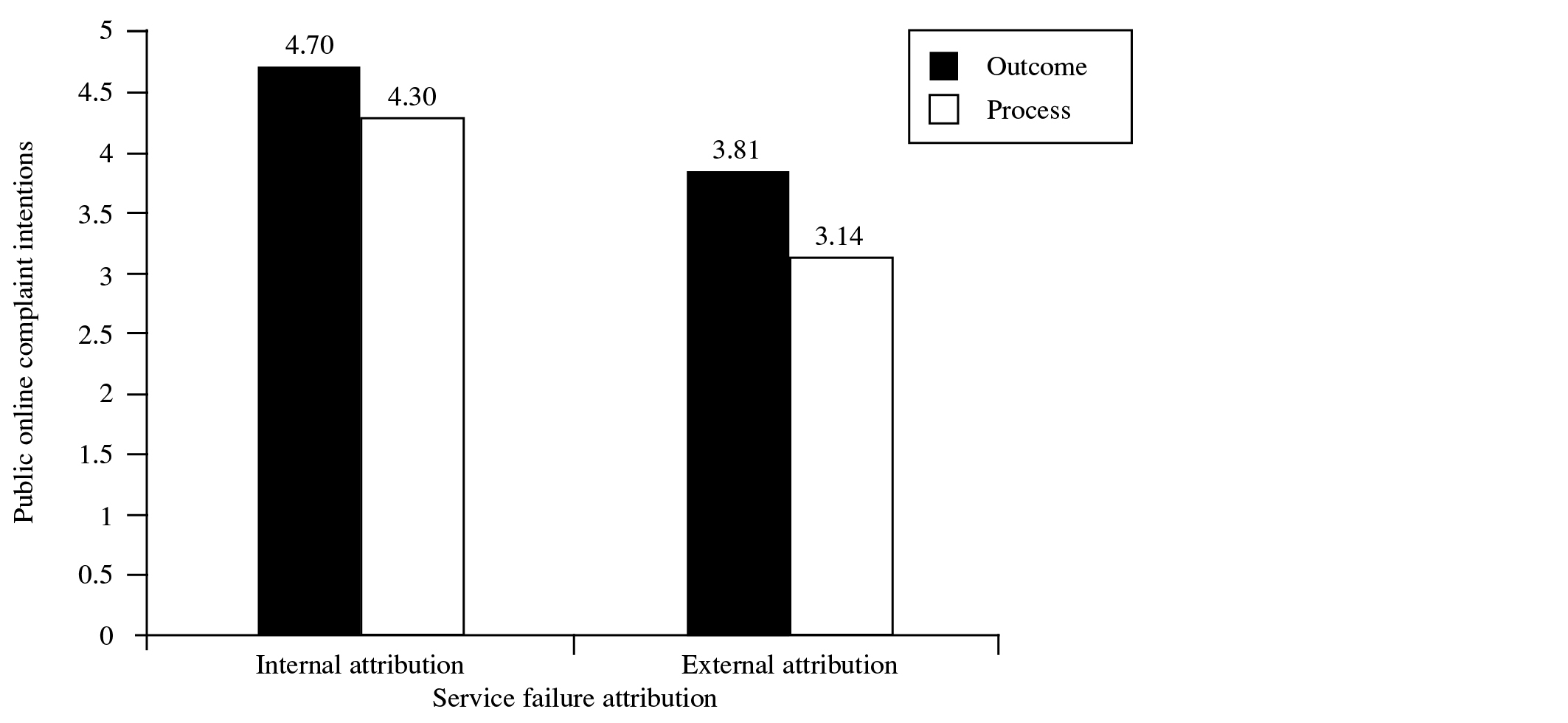
Figure 1. Interaction Effects Between Service Failure and Attribution Types on Customers’ Public Online Complaint Intentions (PUOC)
As shown in Table 3, the effect of outcome failure on customers’ PUOC was stronger than that of process failure, supporting Hypothesis 1a. The effect of internal attribution on customers’ PUOC was stronger than that of external attribution, supporting Hypothesis 2a. A repeated-measures analysis of variance (ANOVA) revealed significant interaction effects between service failure type and attribution type on customers’ PUOC, F(1, 134) = 6.51, p = .01, η2p = .05. Specifically, participants who attributed the service failure to internal factors and deemed it an outcome failure demonstrated stronger PUOC than did those who attributed the service failure to external factors and believed it to be a process failure.
Table 3. Comparison of Effects of Service Failure and Attribution Types on Customers’ Public Online Complaint Intentions

Effects of Service Failure and Attribution Types on Customers’ Private Online Complaint Intentions
Figure 2 illustrates that there was a significant main effect of service failure type on customers’ PVOC, F(1, 134) = 198.67, p < .001, η2p = .60. The main effect of attribution on customers’ PVOC was also significant, F(1, 134) = 479.06, p < .001, η2p = .78.
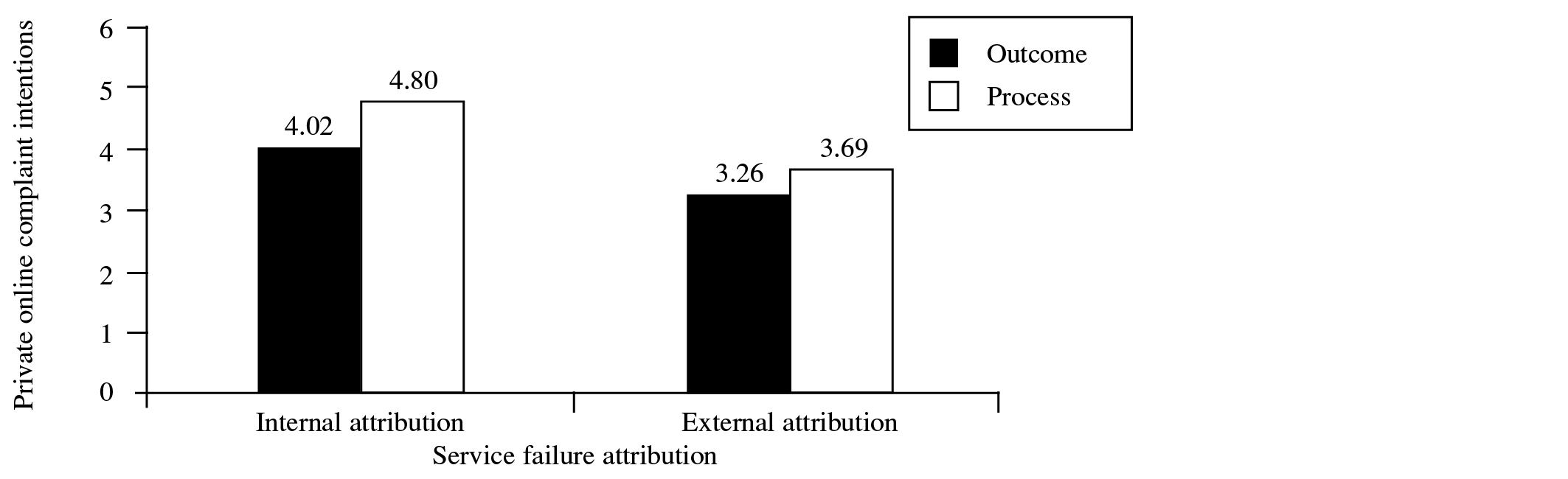
Figure 2. Interaction Effects Between Service Failure and Attribution Types on Customers’ Private Online Complaint Intentions (PVOC)
As indicated in Table 4, the effect of process failure on customers’ PVOC was more significant than that of outcome failure, supporting Hypothesis 1b. Internal attribution had a greater influence on customers’ PVOC than did external attribution, supporting Hypothesis 2b. In addition, the ANOVA results indicate that the interaction effects between service failure and attribution types on customers’ PVOC were significant, F(1, 132) = 16.09, p < .001, η2p = .11. More specifically, participants who attributed the service failure to internal factors and deemed it a process failure possessed higher PVOC than did those who attributed the service failure to external factors and believed it to be an outcome failure.
Table 4. Comparison of Effects of Service Failure and Attribution Types on Customers’ Private Online Complaint Intentions

Discussion
Our findings provide empirical evidence that outcome failure significantly predicted PUOC, whereas process failure significantly predicted PVOC. Prior studies simply tested the distinct influences of outcome and process failure types on the magnitude of customers’ complaint intentions. Extending this work, we divided customer complaints across two dimensions (i.e., PUOC and PVOC) and examined these dimensions in the online shopping context. We found that internal attributions led to greater customer dissatisfaction, a result that is in line with those obtained in prior studies (see, e.g., Song & Wang, 2005; Swanson & Kelley, 2001). However, we also conceptualized internal attribution as involving the service enterprise itself and external attribution as involving environmental factors. Our results then indicated that internal attribution inspired stronger PUOC, whereas external attribution inspired stronger PVOC.
Study 2: Influences of Service Failure Types and Scope of Impact of the Service Failure on Customers’ Online Complaint Intentions
Method
Study 2 had a 2 (service failure type: process vs. outcome) × 2 (scope of impact of the service failure: individual vs. group) mixed design. The background for this study was identical to Study 1, in that we manipulated service failure types and scope of impact of the service failure. However, Study 2 was an extension of the earlier study: Our purpose was to test the differential effect of the scope of impact of a service failure on customers’ PUOC and PVOC, and to investigate whether an interaction effect exists between service failure types and the scope of impact of service failure on customers’ PUOC and PVOC.
Participants
We recruited 148 undergraduate students from Hainan Province, China. After deleting cases with a large amount of missing data, the final useable sample consisted of 140 respondents (valid response rate = 94.6%). There were 63 women and 77 men (Mage = 20.96 years, SD = 1.33, range = 17–23 years) All participants provided informed consent, took part in the study voluntarily, and received compensation of CNY 10 (approximately USD 1.45).
Measure
The measure in this study was identical to that used in Study 1. Responses to all six items were summed as PUOC and PVOC scores, with higher scores indicating stronger online complaint intentions. Cronbach’s alpha values for the PVOC and PUOC item subscales in this study were .79 and .82, respectively.
Procedure
The procedure we followed was the same as in Study 1.
Results
Control and Manipulation Checks
Control check questions were the same as in Study 1. Results indicate that the depicted scenarios were effectively controlled for: online shopping experience (M = 4.39, SD = 0.52), t(139) = 31.71, p < .001, perceived realism (M = 4.39, SD = 0.51), t(139) = 31.66, p < .001, and severity of service failure (M = 4.31, SD = 0.51), t(139) = 30.49, p < .001. Regarding the two-part manipulation check, first, results in Table 5 show that the service failure types were manipulated effectively.
Table 5. Manipulation Check Results for Service Failure Type

Second, we verified whether the manipulation of scope of impact of the service failure in each scenario was successful. Participants responded to the question “When such a thing happens, do you think this is a one-off problem affecting a single customer or a more general one affecting multiple customers?” There were two response options, both rated on a 5-point Likert scale: (1) “It is a one-off problem” and (2) “It is a general problem” (1 = not at all, 5 = absolutely). The manipulation checks (see Table 6) indicate that scope of impact of the service failure was manipulated effectively.
Table 6. Manipulation Check Results for Scope of Impact of the Service Failure

Effects of Service Failure Types and Scope of Impact of Service Failure on Customers’ Public Online Complaint Intentions
Findings in Figure 3 reveal that service failure types influenced customers’ PUOC, F(1, 138) = 589.82, t(138) = 11.14, p < .001, η2p = .81. These results align with those in Study 1, supporting Hypothesis 1a. The scope of impact of the failure (individual vs. group) also influenced customers’ PUOC, F(1, 136) = 376.58, t(138) = −7.73, p < .001, η2p = .74, lending support to Hypothesis 3a.
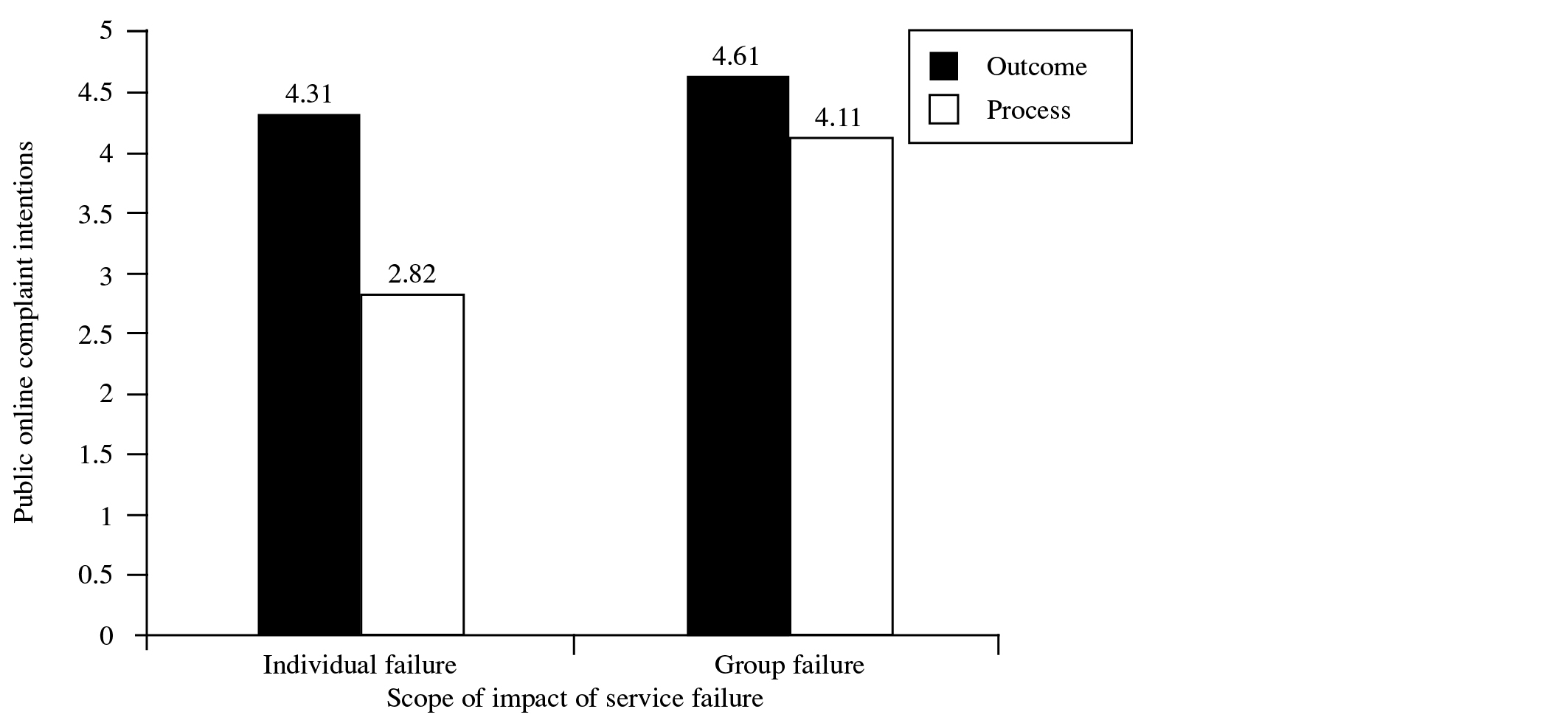
Figure 3. Interaction Effects Between Service Failure Type and Scope of Impact of Service Failure on Customers’ Public Online Complaint Intentions (PUOC)
Group service failure had a more significant effect on customers’ PUOC than did individual service failure (see Table 7). Results of a repeated-measures ANOVA indicate that there was a significant interaction effect between service failure type and scope of impact of service failure on customers’ PUOC, F(1, 136) = 143.25, p < .001, η2p = .51. Specifically, customers’ PUOC was strongest when they experienced a group- and outcome-based service failure.
Table 7. Comparison of the Effects of Service Failure Types and Scope of Impact of Service Failure on Customers’ Public Online Complaint Intentions

Effects of Service Failure Types and Scope of Impact on Customers’ Private Online Complaint Intentions
Our results in Figure 4 also support Hypothesis 1b, in that service failure type influenced customers’ PVOC, F(1, 138) = 374.49, t(138) = −10.98, p < .001, η2p = .73. This conclusion was again the same as in Study 1. The scope of impact of the service failure (group or individual) also significantly affected customers’ PVOC, F(1, 138) = 290.40, t(138) = 8.84, p < .001, η2p = .68, supporting Hypothesis 3b.
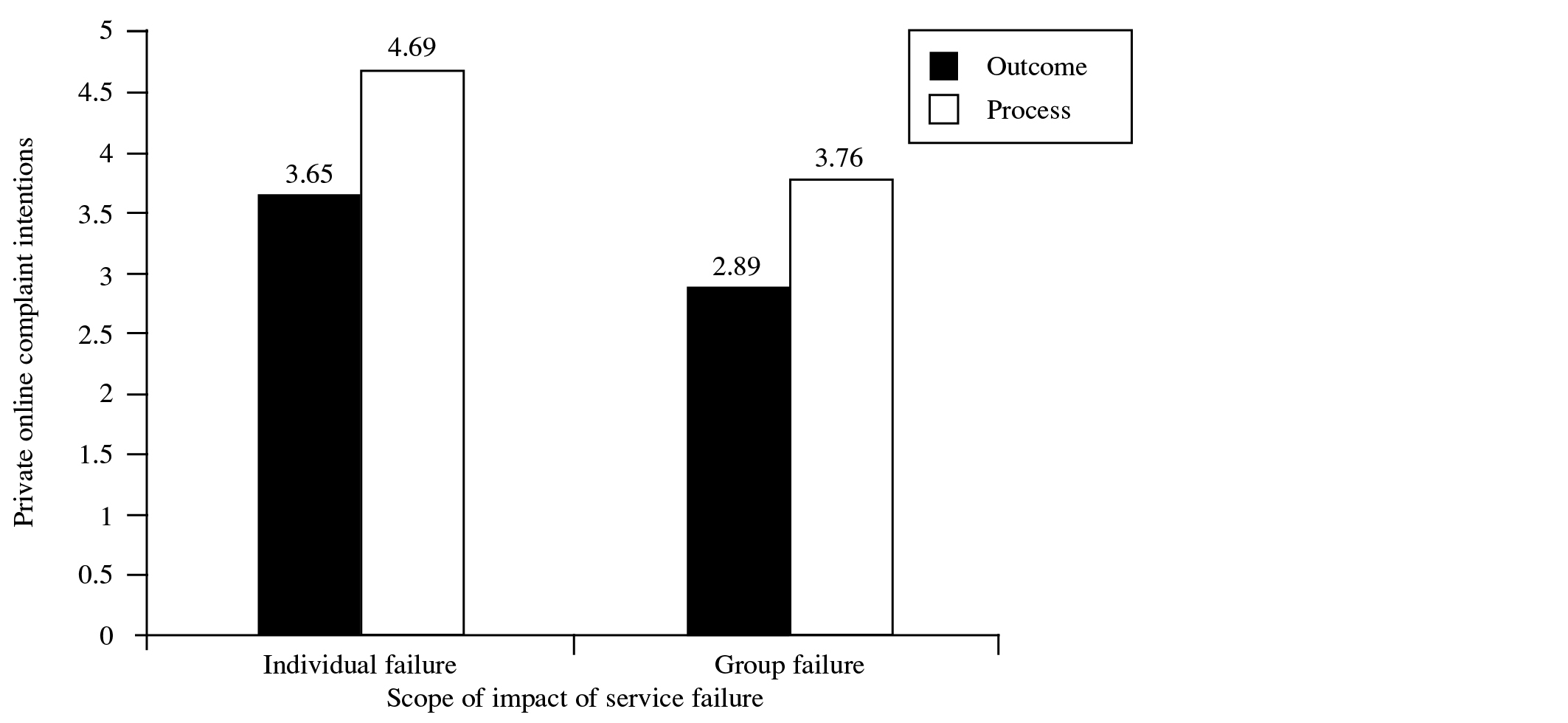
Figure 4. Interaction Effects Between Service Failure Type and Scope of Impact of Service Failure on Customers’ Private Online Complaint Intentions (PVOC)
Individual service failure had a more significant effect on customers’ PVOC than did group service failure (see Table 8). The ANOVA results indicate that there was a nonsignificant interaction effect between service failure type and the scope of impact of the service failure on customers’ PVOC, F(1, 136) = 2.68, p = .10, η2p = .02.
Table 8. Comparison of Effects of Service Failure Types and Scope of Impact of Service Failure on Customers’ Private Online Complaint Intentions

Discussion
In Study 2 we investigated the associations between service failure types, scope of impact of the failure, and COCI. Our findings substantiate Mackie and Smith’s (2018) argument that when customers share a similar experience, they are more likely to express greater dissatisfaction due to emotional contagion. We have also demonstrated that customers who experienced a group (vs. individual) service failure were more likely to complain publicly to receive compensation and warn other customers, as they believed that the service failure may not have been accidental and would be likely to happen again. Customers who experienced an individual service failure were more inclined to complain privately because they considered the service failure a one-time misstep.
Study 3: Influences of Service Failure Types and Customer Inoculation on Customers’ Online Complaint Intentions
Method
Study 3 had a 2 (service failure type: process vs. outcome) × 2 (customer inoculation: inoculation vs. no inoculation) mixed design. Service failure types and customer inoculation were manipulated to (a) examine the effect of customer inoculation on customer complaints online, and (b) explore whether an interaction effect exists between service failure type and customer inoculation on customers’ PUOC and PVOC.
Participants
We recruited 154 undergraduates from a university in Hainan Province, China; 148 provided useable data (valid response rate = 96.1%). There were 73 women and 75 men (Mage = 20.31 years, SD = 1.82, range = 18–24) All participants provided informed consent, took part in the study voluntarily, and received compensation of CNY 10 (approximately USD 1.45).
Measure
The measure was the same as in Study 1. Responses to all six items were again summed as PUOC and PVOC scores, with higher scores reflecting stronger online complaint intentions. Cronbach’s alpha values for the PVOC and PUOC subscales were .89 and .78, respectively.
Procedure
The procedure for Study 3 was the same as in Studies 1 and 2.
Results
Control and Manipulation Checks
For control checks, the questions were the same as those in Studies 1 and 2. Results reveal that the depicted scenarios were effectively controlled for: online shopping experience (M = 4.36, SD = 0.51), t(147) = 32.49, p < .001, perceived realism (M = 4.37, SD = 0.51), t(147) = 32.58, p < .001, and severity of service failure (M = 4.32, SD = 0.54), t(147) = 29.99, p < .001. In terms of manipulation checks, the first question was the same as in Study 1. Table 9 shows that the service failure types were effectively manipulated.
Table 9. Manipulation Check Results for Service Failure Type

The second part was designed to investigate whether the manipulation of customer inoculation was successful. Participants responded to the question “Imagining you are the customer, did the merchant inform you that this event might happen?” by rating the following response: “Online shop staff told me that such an event may occur” (1 = not at all, 5 = absolutely). Table 10 indicates that the manipulation of customer inoculation was successful.
Table 10. Manipulation Check Results for Customer Inoculation

Effects of Service Failure Types and Customer Inoculation on Customers’ Public Online Complaint Intentions
The results of Study 3 (see Figure 5) support Hypothesis 1a, F(1, 144) = 661.50, t(146) = 18.86, p < .001, η2p = .82. The effect of service failure type on customers’ PUOC was the same as in Study 1. Customer inoculation was found to influence customers’ PUOC, F(1, 144) = 127.44, t(146) = −4.81, p < .001, η2p = .47, lending support to Hypothesis 4a.
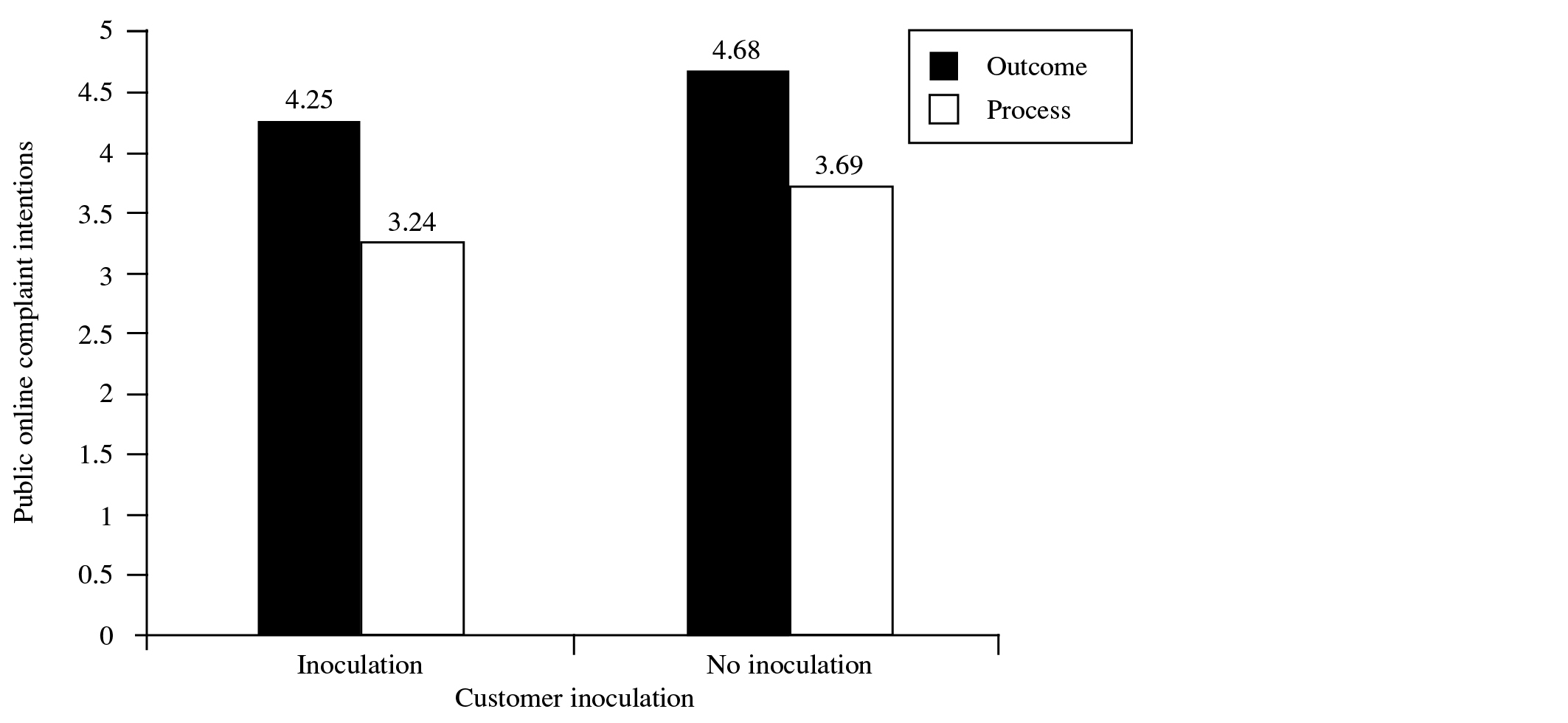
Figure 5. Interaction Effects Between Service Failure Types and Customer Inoculation on Customers’ Public Online Complaint Intentions (PUOC)
Customers who were not inoculated expressed greater intentions to complain publicly than inoculated customers did, in the event of both outcome and process failures (see Table 11). Results of a repeated-measures ANOVA indicate that the interaction effect between service failure type and customer inoculation on customers’ PUOC was nonsignificant, F(1, 144) = .12, p = .727, η2p = .00.
Table 11. Comparison of Effects of Service Failure Type and Customer Inoculation on Customers’ Public Online Complaint Intentions

Effects of Service Failure Types and Customer Inoculation on Customers’ Private Online Complaints Intentions
The results in Figure 6 support Hypothesis 1b, F(1, 144) = 1252.63, t(146) = −30.71, p < .001, η2p = .90.
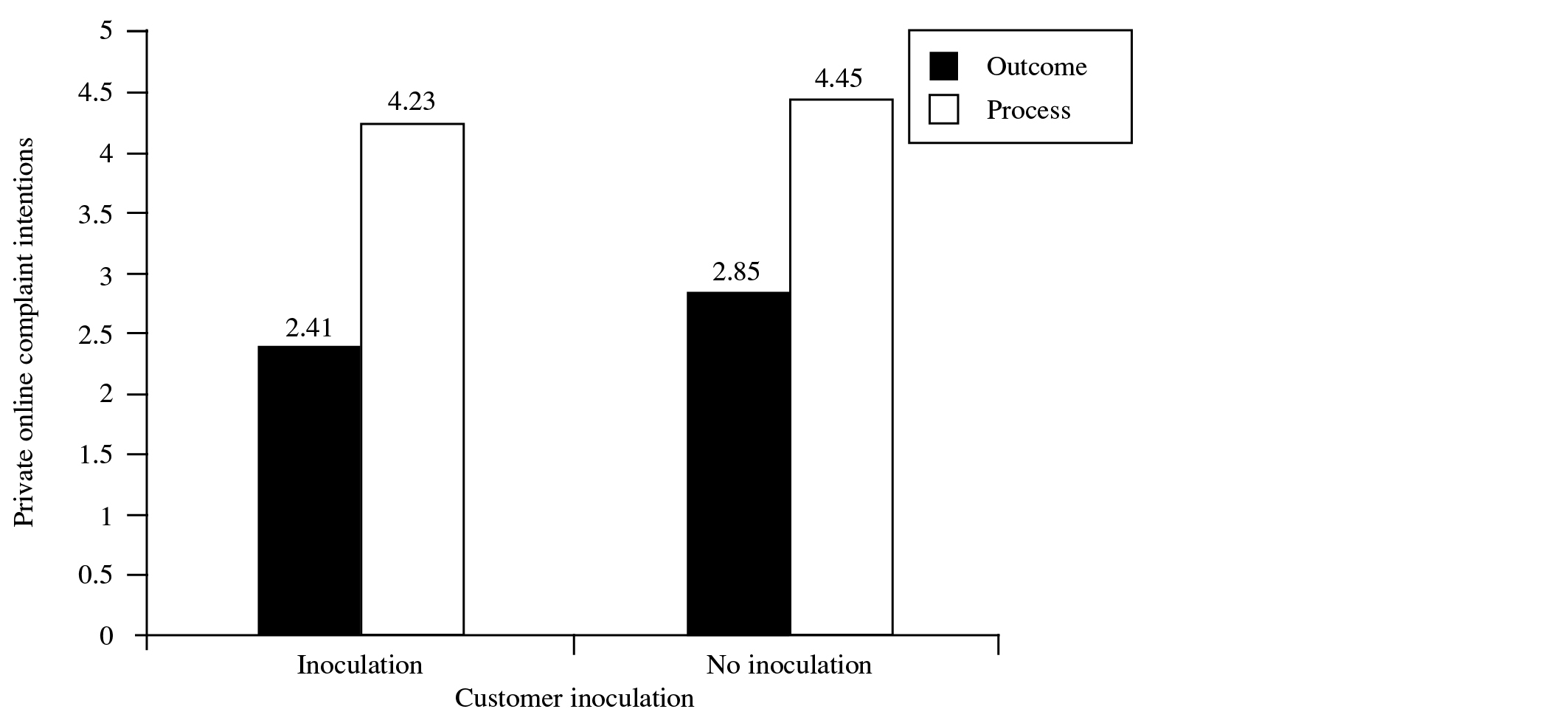
Figure 6. Interaction Effects Between Service Failure Types and Customer Inoculation on Customers’ Private Online Complaint Intentions (PVOC)
The effect of a process failure on customers’ PVOC was more significant than that of an outcome failure (see Table 12). Customer inoculation also influenced customers’ PVOC, F(1, 144) = 374.49, t(138) = −10.98, p < .001, η2p = .24, supporting Hypothesis 4b. Customers who were not inoculated were more inclined to complain privately than were inoculated customers (see Table 12). ANOVA results reveal that the interaction effect between service failure type and customer inoculation on customer PVOC was significant, F(1, 144) = 5.00, p = .03, η2p = .03. The process failure and no-inoculation scenarios resulted in the highest levels of customers’ PVOC.
Table 12. Comparison of Effects of Service Failure Type and Customer Inoculation on Customers’ Private Online Complaint Intentions

Discussion
Our findings show that the interaction between service failure type and customer inoculation was significantly associated with customers’ PVOC, particularly in the context of a process failure when customers had not been inoculated. Service failure type and customer inoculation also each had a separate significant influence on PUOC; however, the interaction term of service failure type × customer inoculation did not have a significant influence on customers’ PUOC. This demonstrates that customer inoculation can decrease customers’ dissatisfaction after a service failure. In other words, when customers have been informed of an upcoming service failure, they may become immune to the failure and express less dissatisfaction than do those who were not inoculated, which is consistent with the findings of Papageorgis and McGuire (1961).
General Discussion
This research makes several contributions to the customer behavior literature by clarifying how service failure types, attributions, scope of impact of service failure, and customer inoculation can influence COCI. First, we are the first to conduct empirical tests within the customer complaint domain to investigate the interaction effect between service failure and these factors on COCI. Second, we divided COCI into two dimensions (PUOC and PVOC) to further investigate the different effects of the above factors. Our findings enhance understanding of customer complaint behavior in online shopping settings. Thus, our work provides a new perspective for further research on factors influencing COCI.
Our study also has practical implications for enterprises. Service businesses should monitor online reviews, especially those by customers who are complaining online publicly. To reduce the negative influence of these online complaints, businesses should respond publicly and in a timely manner, so that these customers perceive they are being taken seriously. Additionally, online business managers should formulate training manuals to help employees recognize potential impending service failures and inform customers in advance of these, which may mitigate patrons’ negative reactions to some extent.
One limitation in this study is that all participants were college students, which may have influenced the external validity of our findings. Future research could include more diverse samples (e.g., office workers, civil servants, farmers) to ensure greater internal and external validity. Second, all three experiments were conducted in a laboratory setting. Subsequent studies could replicate our experimental scenarios in reality (e.g., in the setting of a hospitality service failure) for enhanced authenticity.
References
Andreassen, T. W., & Streukens, S. (2013). Online complaining: Understanding the adoption process and the role of individual and situational characteristics. Managing Service Quality: An International Journal, 23(1), 4–24.
https://doi.org/10.1108/09604521311287632
Chang, C. C., & Chin, Y. C. (2011). Comparing consumer complaint responses to online and offline environment. Internet Research, 21(2), 124–137.
https://doi.org/10.1108/10662241111123720
Chen, K., & Zhang, J. (2014). Are customers more likely to complain in a group? An exploratory study on customer complaints when encountering a group service problem [In Chinese]. China Soft Science, 10, 97–108.
https://doi.org/10.3969/j.issn.1002-9753.2014.10.010
Du, J., & Fan, X. (2011). Research on group consumers’ psychological interaction processes in service failure contexts [In Chinese]. Journal of Management Science, 14(12), 60–70. https://bit.ly/31wqiRz
Du, J., Fan, X., & Feng, T. (2014). Group emotional contagion and complaint intentions in group service failure: The role of group size and group familiarity. Journal of Service Research, 17(3), 326–338.
https://doi.org/10.1177/1094670513519290
E-Commerce Research Center. (2018, August 8). Electronic commerce research center: China’s e-commerce user experience and complaint monitoring report in 2018 [In Chinese]. Author. https://bit.ly/2YUdEu3
Einwiller, S. A., & Johar, G. V. (2013). Countering accusations with inoculation: The moderating role of consumer-company identification. Public Relations Review, 39(3), 198–206.
https://doi.org/10.1016/j.pubrev.2013.03.002
Goldenberg, A., Saguy, T., & Halperin, E. (2014). How group-based emotions are shaped by collective emotions: Evidence for emotional transfer and emotional burden. Journal of Personality and Social Psychology, 107(4), 581–596.
https://doi.org/10.1037/a0037462
Harrison-Walker, L. J. (2001). E-complaining: A content analysis of an internet complaint forum. Journal of Services Marketing, 15(5), 397–412.
https://doi.org/10.1108/EUM0000000005657
Heider, F. (1958). The psychology of interpersonal relations. John Wiley & Sons.
https://doi.org/10.1037/10628-000
Hsiao, C.-H. (2011). Predicting online consumer complaints in northern Taiwan. African Journal of Business Management, 5(13), 5281–5291.
Iglesias, V. (2009). The attribution of service failures: Effects on consumer satisfaction. The Service Industries Journal, 29(2), 127–141.
https://doi.org/10.1080/02642060802293088
Jian, Z., & Ke, Y. (2017). The impacts of service failure and service recovery on customer satisfaction and loyalty in the context of online shopping [In Chinese]. Management Review, 29(1), 175–186.
https://doi.org/10.14120/j.cnki.cn11-5057/f.2017.01.018
Johnston, R. (1995). The determinants of service quality: Satisfiers and dissatisfiers. International Journal of Service Industry Management, 6(5), 53–71.
https://doi.org/10.1108/09564239510101536
Mackie, D. M., & Smith, E. R. (2018). Intergroup emotions theory: Production, regulation, and modification of group-based emotions. Advances in Experimental Social Psychology, 58, 1–69.
https://doi.org/10.1016/bs.aesp.2018.03.001
Mikolon, S., Quaiser, B., & Wieseke, J. (2015). Don’t try harder: Using customer inoculation to build resistance against service failures. Journal of the Academy of Marketing Science, 43(4), 512–527.
https://doi.org/10.1007/s11747-014-0398-1
Papageorgis, D., & McGuire, W. J. (1961). The generality of immunity to persuasion produced by pre-exposure to weakened counterarguments. The Journal of Abnormal and Social Psychology, 62(3), 475–481.
https://doi.org/10.1037/h0048430
Smith, A. K., Bolton, R. N., & Wagner, B. J. (1999). A model of customer satisfaction with service encounters involving failure and recovery. Journal of Marketing Research, 36(3), 356–372.
https://doi.org/10.1177/002224379903600305
Song, Y., & Wang, X. (2005). The impact of service failure attributions on service recovery effects [In Chinese]. Nankai Business Review, 8(4), 12–17.
https://doi.org/10.3969/j.issn.1008-3448.2005.04.003
Swanson, S. R., & Kelley, S. W. (2001). Attributions and outcomes of the service recovery process. Journal of Marketing Theory and Practice, 9(4), 50–65.
https://doi.org/10.1080/10696679.2001.11501903
Weitzl, W. J., & Hutzinger, C. (2019). Rise and fall of complainants’ desires: The role of pre-failure brand commitment and online service recovery satisfaction. Computers in Human Behavior, 97, 116–129.
https://doi.org/10.1016/j.chb.2019.02.029
Weitzl, W., Hutzinger, C., & Einwiller, S. (2018). An empirical study on how webcare mitigates complainants’ failure attributions and negative word-of-mouth. Computers in Human Behavior, 89, 316–327.
https://doi.org/10.1016/j.chb.2018.07.012
Zhu, Z., Sivakumar, K., & Parasuraman, A. (2004). A mathematical model of service failure and recovery strategies. Decision Sciences, 35(3), 493–525.
https://doi.org/10.1111/j.0011-7315.2004.02597.x
Andreassen, T. W., & Streukens, S. (2013). Online complaining: Understanding the adoption process and the role of individual and situational characteristics. Managing Service Quality: An International Journal, 23(1), 4–24.
https://doi.org/10.1108/09604521311287632
Chang, C. C., & Chin, Y. C. (2011). Comparing consumer complaint responses to online and offline environment. Internet Research, 21(2), 124–137.
https://doi.org/10.1108/10662241111123720
Chen, K., & Zhang, J. (2014). Are customers more likely to complain in a group? An exploratory study on customer complaints when encountering a group service problem [In Chinese]. China Soft Science, 10, 97–108.
https://doi.org/10.3969/j.issn.1002-9753.2014.10.010
Du, J., & Fan, X. (2011). Research on group consumers’ psychological interaction processes in service failure contexts [In Chinese]. Journal of Management Science, 14(12), 60–70. https://bit.ly/31wqiRz
Du, J., Fan, X., & Feng, T. (2014). Group emotional contagion and complaint intentions in group service failure: The role of group size and group familiarity. Journal of Service Research, 17(3), 326–338.
https://doi.org/10.1177/1094670513519290
E-Commerce Research Center. (2018, August 8). Electronic commerce research center: China’s e-commerce user experience and complaint monitoring report in 2018 [In Chinese]. Author. https://bit.ly/2YUdEu3
Einwiller, S. A., & Johar, G. V. (2013). Countering accusations with inoculation: The moderating role of consumer-company identification. Public Relations Review, 39(3), 198–206.
https://doi.org/10.1016/j.pubrev.2013.03.002
Goldenberg, A., Saguy, T., & Halperin, E. (2014). How group-based emotions are shaped by collective emotions: Evidence for emotional transfer and emotional burden. Journal of Personality and Social Psychology, 107(4), 581–596.
https://doi.org/10.1037/a0037462
Harrison-Walker, L. J. (2001). E-complaining: A content analysis of an internet complaint forum. Journal of Services Marketing, 15(5), 397–412.
https://doi.org/10.1108/EUM0000000005657
Heider, F. (1958). The psychology of interpersonal relations. John Wiley & Sons.
https://doi.org/10.1037/10628-000
Hsiao, C.-H. (2011). Predicting online consumer complaints in northern Taiwan. African Journal of Business Management, 5(13), 5281–5291.
Iglesias, V. (2009). The attribution of service failures: Effects on consumer satisfaction. The Service Industries Journal, 29(2), 127–141.
https://doi.org/10.1080/02642060802293088
Jian, Z., & Ke, Y. (2017). The impacts of service failure and service recovery on customer satisfaction and loyalty in the context of online shopping [In Chinese]. Management Review, 29(1), 175–186.
https://doi.org/10.14120/j.cnki.cn11-5057/f.2017.01.018
Johnston, R. (1995). The determinants of service quality: Satisfiers and dissatisfiers. International Journal of Service Industry Management, 6(5), 53–71.
https://doi.org/10.1108/09564239510101536
Mackie, D. M., & Smith, E. R. (2018). Intergroup emotions theory: Production, regulation, and modification of group-based emotions. Advances in Experimental Social Psychology, 58, 1–69.
https://doi.org/10.1016/bs.aesp.2018.03.001
Mikolon, S., Quaiser, B., & Wieseke, J. (2015). Don’t try harder: Using customer inoculation to build resistance against service failures. Journal of the Academy of Marketing Science, 43(4), 512–527.
https://doi.org/10.1007/s11747-014-0398-1
Papageorgis, D., & McGuire, W. J. (1961). The generality of immunity to persuasion produced by pre-exposure to weakened counterarguments. The Journal of Abnormal and Social Psychology, 62(3), 475–481.
https://doi.org/10.1037/h0048430
Smith, A. K., Bolton, R. N., & Wagner, B. J. (1999). A model of customer satisfaction with service encounters involving failure and recovery. Journal of Marketing Research, 36(3), 356–372.
https://doi.org/10.1177/002224379903600305
Song, Y., & Wang, X. (2005). The impact of service failure attributions on service recovery effects [In Chinese]. Nankai Business Review, 8(4), 12–17.
https://doi.org/10.3969/j.issn.1008-3448.2005.04.003
Swanson, S. R., & Kelley, S. W. (2001). Attributions and outcomes of the service recovery process. Journal of Marketing Theory and Practice, 9(4), 50–65.
https://doi.org/10.1080/10696679.2001.11501903
Weitzl, W. J., & Hutzinger, C. (2019). Rise and fall of complainants’ desires: The role of pre-failure brand commitment and online service recovery satisfaction. Computers in Human Behavior, 97, 116–129.
https://doi.org/10.1016/j.chb.2019.02.029
Weitzl, W., Hutzinger, C., & Einwiller, S. (2018). An empirical study on how webcare mitigates complainants’ failure attributions and negative word-of-mouth. Computers in Human Behavior, 89, 316–327.
https://doi.org/10.1016/j.chb.2018.07.012
Zhu, Z., Sivakumar, K., & Parasuraman, A. (2004). A mathematical model of service failure and recovery strategies. Decision Sciences, 35(3), 493–525.
https://doi.org/10.1111/j.0011-7315.2004.02597.x
Table 1. Manipulation Check Results for Service Failure Type

Table 2. Manipulation Check Results for Service Failure Attribution

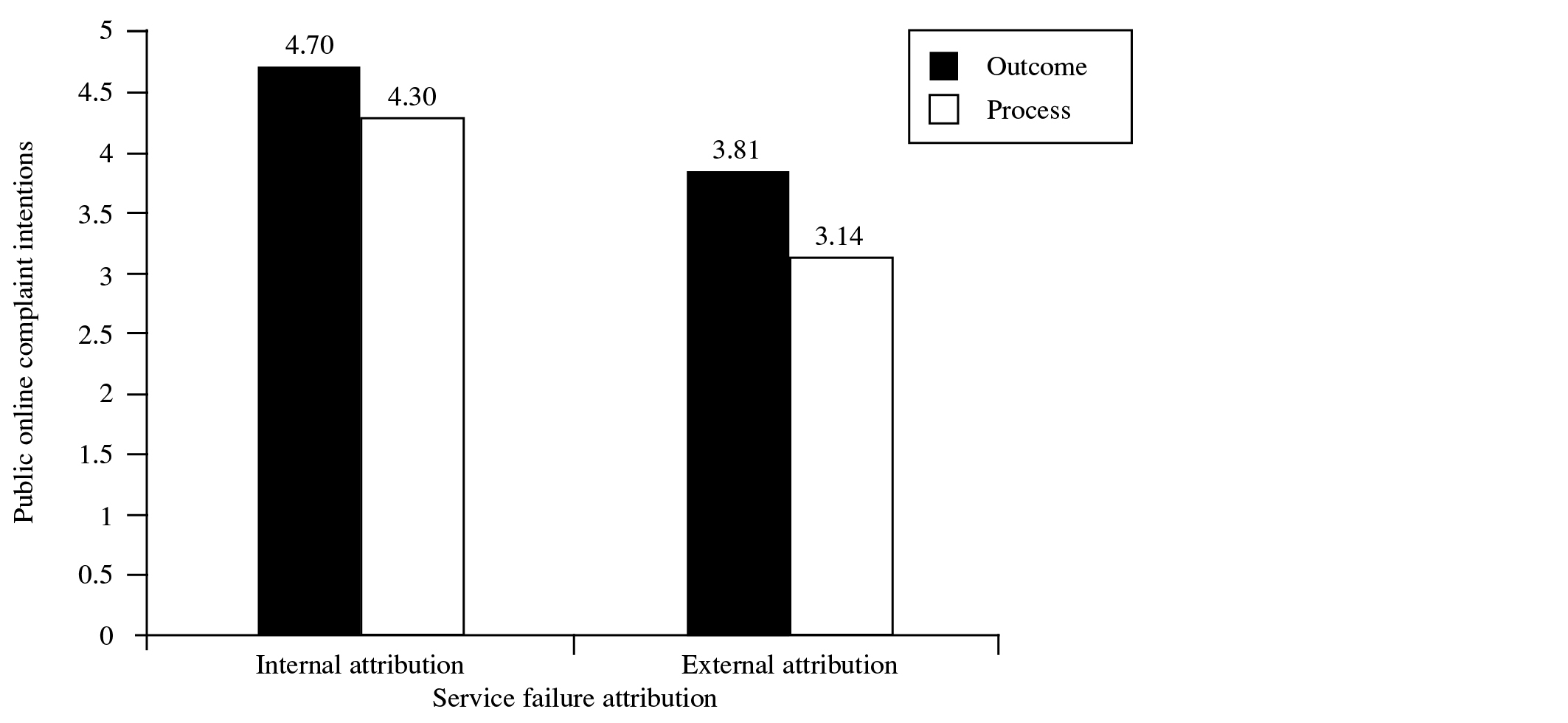
Figure 1. Interaction Effects Between Service Failure and Attribution Types on Customers’ Public Online Complaint Intentions (PUOC)
Table 3. Comparison of Effects of Service Failure and Attribution Types on Customers’ Public Online Complaint Intentions

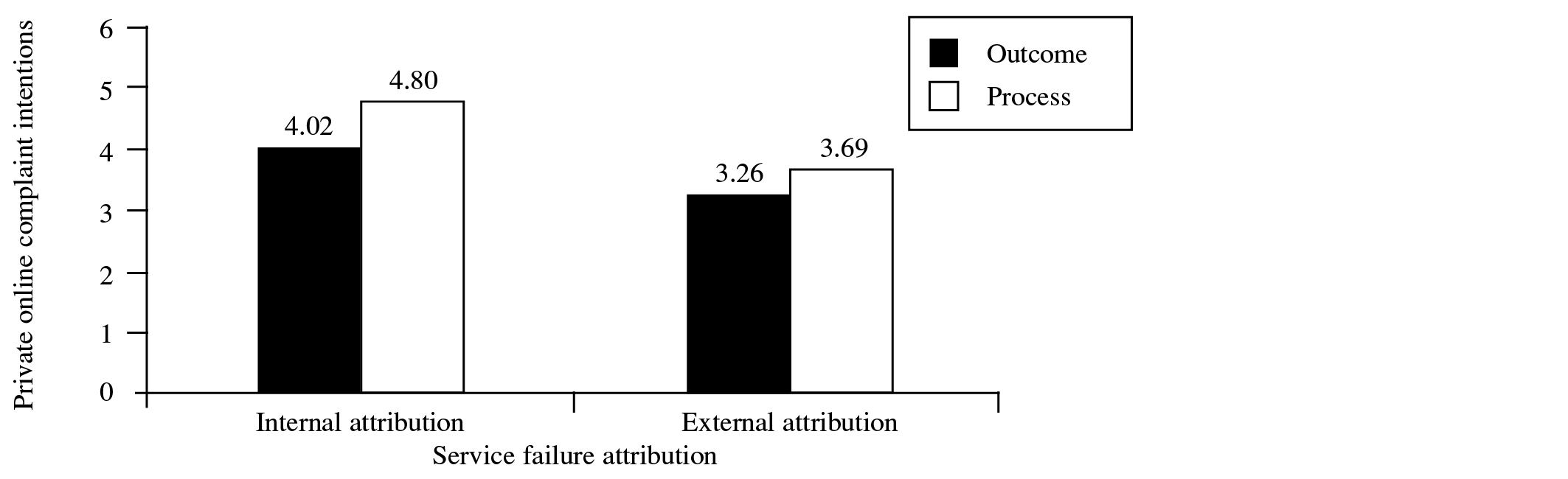
Figure 2. Interaction Effects Between Service Failure and Attribution Types on Customers’ Private Online Complaint Intentions (PVOC)
Table 4. Comparison of Effects of Service Failure and Attribution Types on Customers’ Private Online Complaint Intentions

Table 5. Manipulation Check Results for Service Failure Type

Table 6. Manipulation Check Results for Scope of Impact of the Service Failure

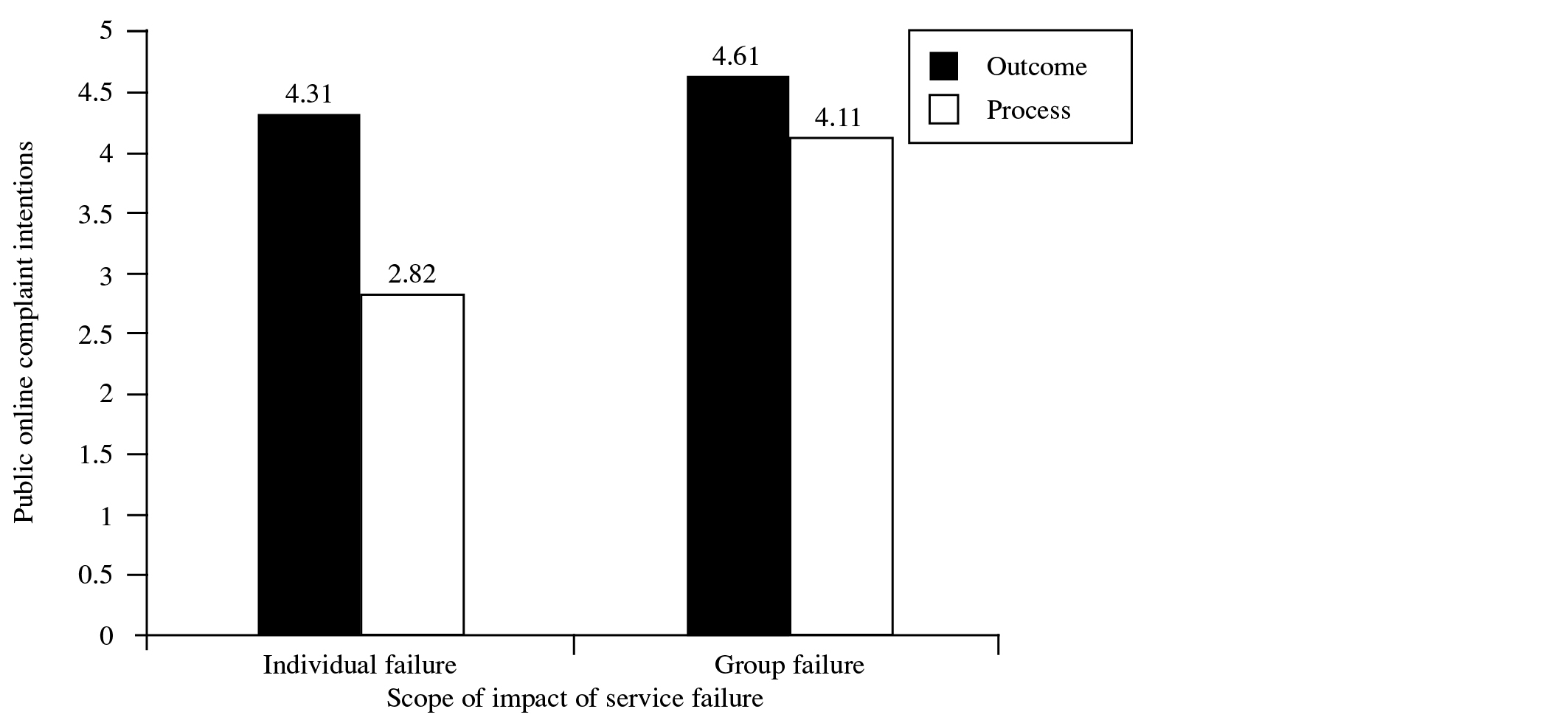
Figure 3. Interaction Effects Between Service Failure Type and Scope of Impact of Service Failure on Customers’ Public Online Complaint Intentions (PUOC)
Table 7. Comparison of the Effects of Service Failure Types and Scope of Impact of Service Failure on Customers’ Public Online Complaint Intentions

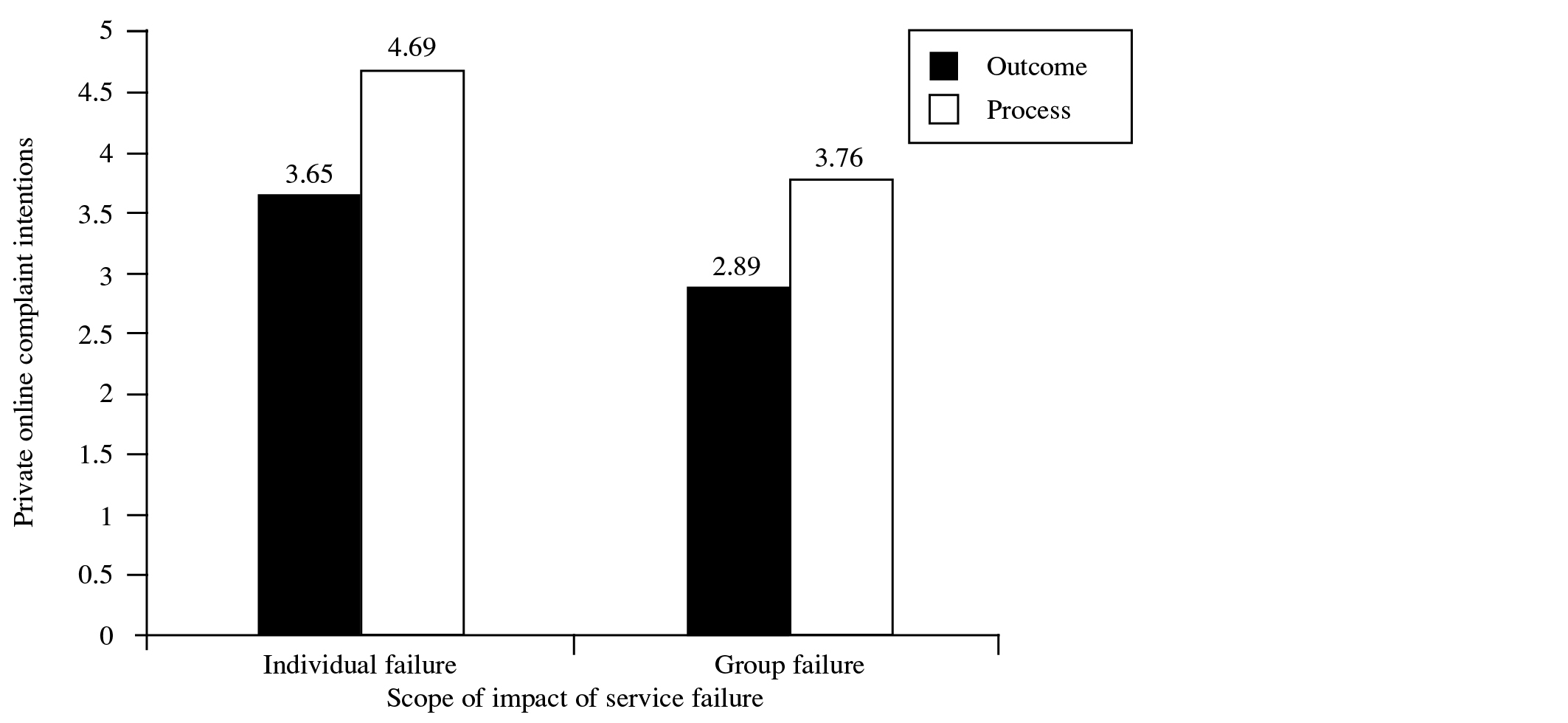
Figure 4. Interaction Effects Between Service Failure Type and Scope of Impact of Service Failure on Customers’ Private Online Complaint Intentions (PVOC)
Table 8. Comparison of Effects of Service Failure Types and Scope of Impact of Service Failure on Customers’ Private Online Complaint Intentions

Table 9. Manipulation Check Results for Service Failure Type

Table 10. Manipulation Check Results for Customer Inoculation

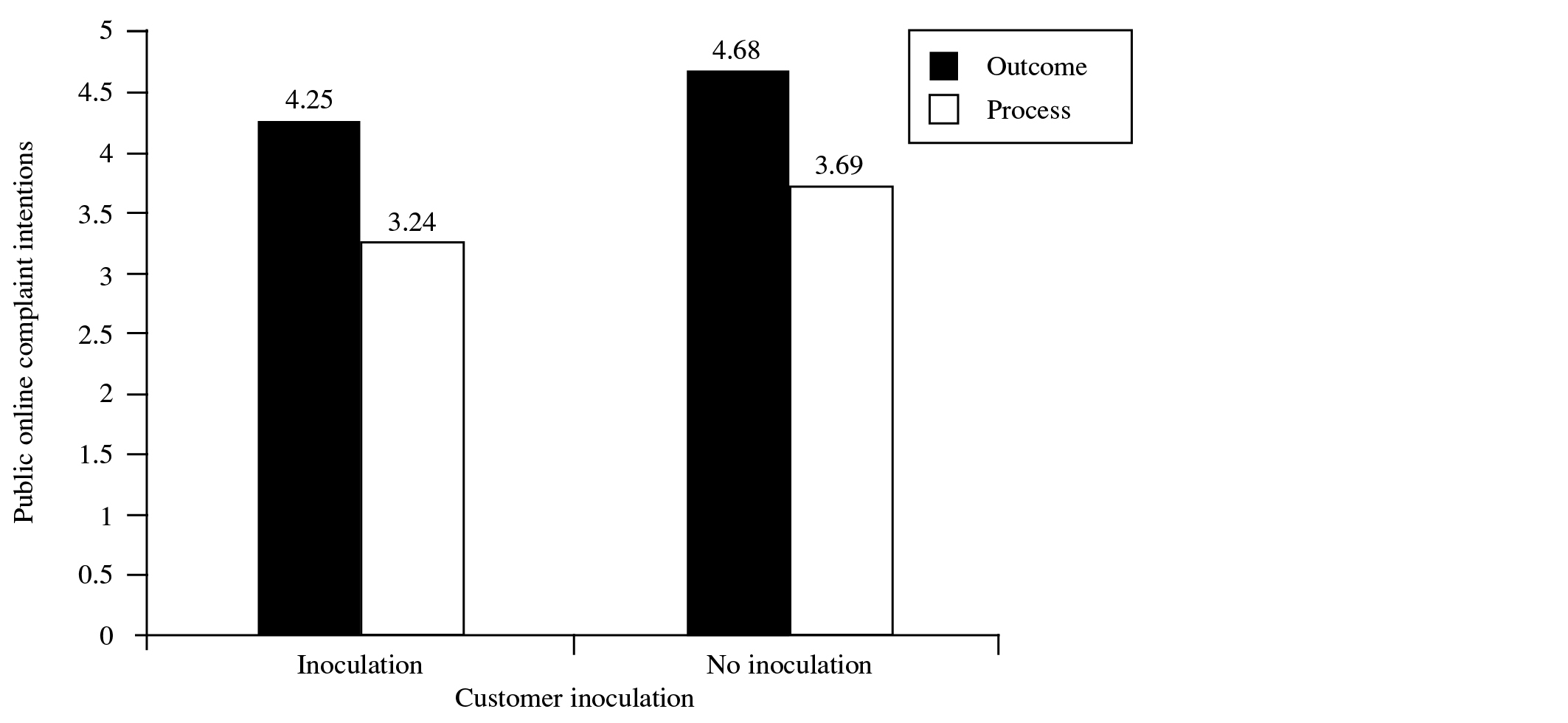
Figure 5. Interaction Effects Between Service Failure Types and Customer Inoculation on Customers’ Public Online Complaint Intentions (PUOC)
Table 11. Comparison of Effects of Service Failure Type and Customer Inoculation on Customers’ Public Online Complaint Intentions

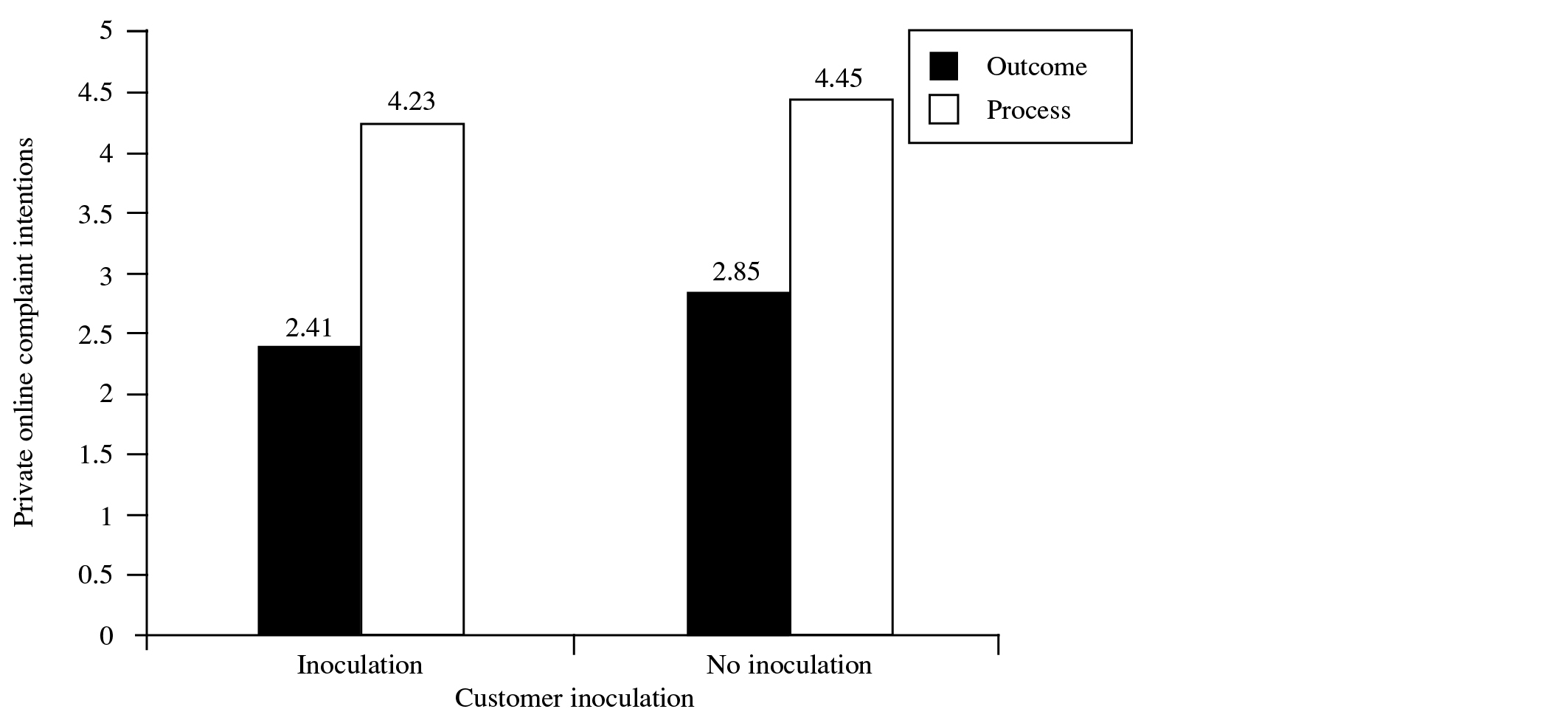
Figure 6. Interaction Effects Between Service Failure Types and Customer Inoculation on Customers’ Private Online Complaint Intentions (PVOC)
Table 12. Comparison of Effects of Service Failure Type and Customer Inoculation on Customers’ Private Online Complaint Intentions

This work was supported by the National Natural Science Foundation of China (71962006
71602104
71602102) and the Hainan Provincial Social Science Foundation (HNSKJD19-11
HNSKJD19-15).
A full description of the research manipulations used in this paper can be obtained by writing to the authors.
Lilei Wang, Tourism College, Hainan University, No. 58 People Road, Meilan District, Haikou 570228, People’s Republic of China. Email: [email protected]