Teacher online feedback and learning motivation: Learning engagement as a mediator
Main Article Content
Teacher online feedback (TOF) through online course delivery has been identified as key to promoting and validating knowledge in online learning environments. We examined the relationships between TOF, learning motivation, and learning engagement, and the mediating role of learning engagement in the relationship between TOF and learning motivation, using structural equation modeling and bootstrap analysis. Participants comprised 312 university students of College English courses in China. The results indicated that TOF was positively related to both learning motivation and learning engagement. Further, the positive effect of TOF on learning motivation was partially mediated by learning engagement. Theoretical implications and practical implications for language teachers’ implementation of TOF are discussed.
Teacher feedback has been widely studied owing to its enormous potential to support students’ learning, especially in relation to classroom settings (Hattie & Timperley, 2007; Price, Handley, Millar, & O’Donovan, 2010). With the development of network technology, online course delivery has for many years been widely used by higher education instructors implementing blended online course formats. There is growing interest in the study of feedback in online learning environments, particularly in higher education, as feedback has been identified as key to promoting and validating knowledge in the online setting (Coll, Rochera, & de Gispert, 2014). Researchers have demonstrated that participation and interaction in online learning environments require teacher intervention through feedback or other means (Sorensen & Takle, 2005; Zhu, 2006), during which the teacher role is as a moderator or facilitator (Salmon, 2000).
Regarding the content of teacher online feedback (TOF), researchers (e.g., Coll et al., 2014; Narciss, 2013; Narciss & Huth, 2006) have proposed that productive feedback should include two kinds of information: Verification refers to information about outcomes or the correctness of an answer, whereas elaboration involves more complex information about knowledge of concepts, working rules, task requirements, errors, procedures, and metacognition. However, although much attention has been paid to the effectiveness of TOF (Coll et al., 2014; Yang, 2016), how TOF affects learning motivation is not fully understood. Thus, we examined if learning engagement mediates the relationship between TOF and learning motivation.
Literature Review and Hypothesis Development
Teacher Online Feedback and Learning Motivation
Online learning environments constructed through the advancement of educational technologies necessitate the use of TOF, which is both informational for instructing students’ learning, and motivational for stimulating students’ efforts (Shute, 2008). TOF refers to the guidance of, and feedback about, students’ learning that teachers provide using network technology. Use of the network platform means that TOF is, to some extent, not limited by time and place, which is helpful in guiding students’ personalized learning and promoting autonomous learning (Ryan, Henderson, & Phillips, 2019). The benefit of TOF over traditional face-to-face or paper-based feedback is that teachers can present and submit responses in a more structured way (Ozkara & Cakir, 2018).
Recent researchers have empirically examined the relationship between TOF and learning motivation (Bailey, Hendricks, & Applewhite, 2015; Hibbert, 2017). Their results have demonstrated that TOF settings allow the implementation of dynamic feedback processes that steer students toward a desirable pattern of interaction (Noroozi, Biemans, & Mulder, 2016), motivate students to take active responsibility for their learning, and elevate instructors’ ability to serve as the facilitator, strategist, and coordinator of learning activities (Liu & Yu, 2019). Researchers have also explored the teacher’s role in online learning environments, in particular, teaching presence (Garrison & Anderson, 2003). Teaching presence is defined as “the design, facilitation, and direction of cognitive and social processes for the purpose of realizing personally meaningful and educationally worthwhile learning outcomes” (Anderson, Rourke, Garrison, & Archer, 2001, p. 5). Jenson, Kummer, and Godoy (2015) suggested that active TOF results in students having a more positive attitude when using an active constructive approach to learning.
Teacher Online Feedback and Learning Engagement
Learning engagement refers to the continuous positive emotions that students may have during the learning process, and has the three characteristics of vitality, dedication, and focus (Schaufeli, Martínez, Pinto, Salanova, & Bakker, 2002). Many researchers have found that the higher the perceived level of teacher support is, the more time and energy students invest in learning (e.g., Hew, 2016; Jung & Lee, 2018). Kearsley and Shneiderman (1998) proposed engagement theory as a model of technology-based environment learning, and stated that learning engagement can be accomplished through an emphasis on collaborative efforts, project-based assignments, and a nonacademic focus. O’Brien and Toms (2008) sought to critically deconstruct students’ engagement experiences with technology and found a common trajectory, as their technology-based learning engagement partly resulted from collaborative efforts. Further, Cunningham (2010) indicated that TOF amplifies learning engagement by promoting students’ learning attitude when they interact and make connections in their immediate online interactive context. The creative, meaningful, and authentic nature of these online teacher–student interactions increases the required intrinsic motivation for learning engagement for student learner success.
Learning Engagement as a Mediator of the Relationship Between Teacher Online Feedback and Learning Motivation
Psychologists have pointed out that, in addition to the direct impact of independent variables on dependent variables, intermediary mechanisms should be taken into account (Baron & Kenny, 1986; Wood, Froh, & Geraghty, 2010). Thus, given the apparent strong relationship between TOF and learning motivation, it is important to consider the link mechanism. According to the positive affect hypothesis of learning engagement (Çakiroğlu & Erdemir, 2019), TOF causes students to experience a sense of satisfaction and sometimes delight from teacher support, so that they persist with learning and accept online environments (Larmuseau, Desmet, & Depaepe, 2019). Therefore, we query if there is a mediator effect in the TOF process that affects students’ learning motivation. In addition, TOF spans both explicit and implicit characteristics in its support of student learning, because it not only provides direct pedagogical instructions but also emotional support. Therefore, we proposed the following hypotheses:
Hypothesis 1: Teacher online feedback will be positively correlated with students’ learning motivation.
Hypothesis 2: Teacher online feedback will be positively correlated with students’ learning engagement.
Hypothesis 3: The relationship between teacher online feedback and learning motivation will be mediated by learning engagement.
Method
Participants and Procedure
Participants were 318 undergraduate students enrolled in a College English course at Zhejiang Normal University. Participants completed a face-to-face survey in the classroom at class intervals, with 312 (185 men, 127 women) providing valid responses. The survey was distributed on the spot and collected immediately after completion. In China, College English involves the exclusive use of English as a second language as the medium for instruction and learning, and it is a compulsory course for undergraduate students for a minimum of 2 years. At Zhejiang Normal University the College English teaching and research group combines online teaching with traditional classroom teaching and through a network platform, where teachers and students interact, and teachers give feedback and evaluate students’ learning.
Most previous researchers have examined teacher support for students’ learning through students’ self-reports, that is, how teacher support is perceived by students (Li & Bai, 2018). Therefore, we adopted a survey to investigate students’ self-reported perception of TOF and its relationship with learning motivation. As all students participated in the network platform learning and online interaction, their participation in the survey was cooperative and voluntary. To avoid potential bias from our involvement on this platform, participants were restricted to students from our colleagues’ teaching classes, and we anonymized the research data to protect participants’ privacy.
Measures
Students’ perceptions of teacher online feedback. We assessed students’ perceptions of TOF with the feedback constructs developed by Babad (1990), Hattie and Timperley (2007), Nicol and Macfarlane-Dick (2006), and Price et al. (2010). Three dimensions comprised 16 items: developmental feedback (six items, Cronbach’s α = .90; e.g., “My teacher provided some information on how to make progress in learning”), activity-based feedback (five items, Cronbach’s α = .87; e.g., “My teacher conversed with me about how to develop my writing ability”), and evaluative feedback (five items, Cronbach’s α = .91; e.g., “My teacher graded my homework”). Responses were made on a 6-point Likert scale ranging from 1 = strongly disagree to 6 = strongly agree, such that a higher score indicated greater perceived TOF. Confirmatory factor analysis (CFA) results showed that the measurement model had a good fit to the data: chi square (χ2)/degrees of freedom (df) = 1.87, comparative fit index (CFI) = .96, Tucker–Lewis index = .96, root mean square error of approximation (RMSEA) = .078, standardized root mean square error residual (SRMR) = .036, as well as good reliability.
Learning engagement. We revised Schaufeli et al.’s (2002) eight-item Learning Engagement Scale to fit the study context. A sample item is “I was willing to spend time learning English on the network platform.” Participants rated the degree of conformity with their actual learning situation on a 6-point Likert scale ranging from 1 = very inconsistent to 6 = very consistent. Higher scores indicate higher engagement in learning. As Cronbach’s α was .89, the scale had good reliability.
Learning motivation. We revised 18 motivation items from Guilloteaux and Dörnyei’s (2008) and Kormos and Csizér’s (2014) studies to fit the study context. The initial CFA revealed that factor loadings of two items (“I was ready to work hard at English” and “I really enjoyed learning English”) were low. After removal of these two items, CFA showed that the remaining 16 items in the measurement model had a satisfactory fit to the data: χ2/df = 1.80, CFI = .94, TLI = .95, RMSEA = .056, SRMR = .061. The items were divided into three dimensions: confidence and effort (seven items), interest in English language learning (four items), and attitude toward learning motivation (five items). Participants rated the degree of conformity with their actual learning situation on a 6-point Likert scale ranging from 1 = very inconsistent to 6 = very consistent, such that higher scores indicated higher learning motivation. Cronbach’s α was .90, indicating good reliability.
Data Analysis
We used SPSS 21.0 and Amos 21.0 for data analysis. First, descriptive statistics were used to describe TOF (i.e., developmental, activity-based, and evaluative feedback), learning engagement, and learning motivation. Second, Pearson correlation analysis was used to explore the relationships between TOF, learning engagement, and learning motivation. Third, we used structural equation modeling for path analysis to verify the hypotheses.
Results
Descriptive Statistics and Correlation Analysis
Means and standard deviations of the TOF components, learning engagement, and learning motivation are shown in Table 1.
Table 1. Means and Standard Deviations of Scores for Study Variables

Note. N = 312.
Activity-based feedback was rated the highest by students, and developmental feedback and evaluative feedback also received high ratings. TOF was communicated to students as a component of guiding and scaffolding their learning, and students preferred to strengthen communication and interaction with their teachers through the network platform, expected teachers to give guidance on strategic learning methods, and had a relatively weak response to such evaluative feedback as “well done.” As learning engagement was rated slightly above average, this suggested that students identified their effort investment in learning via the network platform as insufficient. Finally, students rated their learning motivation as high, suggesting they had strong motivation for learning through the network platform.
Table 2 shows that all bivariate correlations were significant and positive. The three components of TOF (i.e., developmental, activity-based, and evaluative feedback) were significantly positively correlated with learning engagement and learning motivation. Learning engagement was significantly correlated with learning motivation. As the correlations of the three TOF, learning engagement, and learning motivation variables were all below .70, this excluded the issue of collinearity, and demonstrated their suitability for structural equation modeling.
Table 2. Bivariate Correlations and Internal Consistency Reliability for Study Variables

Note. Cronbach’s α are shown on the diagonal in parentheses.
** p < .01.
Structural Equation Modeling and Bootstrap Analysis
Three kinds of path analysis were used to verify both the correlations of the three groups of variables and if learning engagement was an intermediary to regulate TOF and learning motivation. We used path analysis to test three models: In Model A, TOF predicted learning engagement and learning motivation (direct model); In Model B, learning engagement mediated the relationship between TOF and learning motivation (intermediary model); and in Model C, which is similar to Model B, there was no direct path from TOF to learning motivation. The fit indices of RMSEA, SRMR, CFI, TLI, and GFI for each model are shown in Table 3.
Table 3. Fit Indices of the Three Models

Note. N = 312. Model A = direct effect model, Model B = mediated model, Model C = mediated model with the direct path from TOF to learning motivation omitted. χ2 = chi square, df = degrees of freedom, TLI = Tucker–Lewis index, CFI = comparative fit index, RMSEA = root mean square error of approximation, SRMR = standardized root mean square residual.
We used several indices to evaluate the fit of the three models. Because the χ2 test is highly sensitive to sample size, we calculated the χ2/df ratio. We applied the common guidelines for acceptable model fit: χ2/df ≤ 3.00 (Carmines & McIver, 1981), RMSEA ≤ .08, CFI ≥ .95, TLI ≥ .95, and SRMR ≤ .10 (Marsh, Hau, & Grayson, 2005). Table 3 shows that the fit indices of Model B (intermediary model) were the most satisfactory.
As noted in Model A (direct model; see Figure 1), TOF was significantly correlated with learning engagement and learning motivation. Hypotheses 1 and 2 were thus supported. In Model B (intermediary model) TOF was significantly related to learning engagement, and the path coefficient from TOF to learning motivation was lower than that of Model A, but still significant. Results of a Sobel test showed that the addition of learning engagement to the model reduced the effect of TOF on learning motivation (z = 5.36, p < .001). Hypothesis 3 was therefore supported. Finally, the fit indices of Model C (figure omitted), which had no direct path between TOF and learning motivation, were poor (see Table 3), indicating that Model B was more consistent with data than was Model C. According to Baron and Kenny’s (1986) criteria, these results showed that learning engagement partially mediated the relationship between TOF and learning motivation.
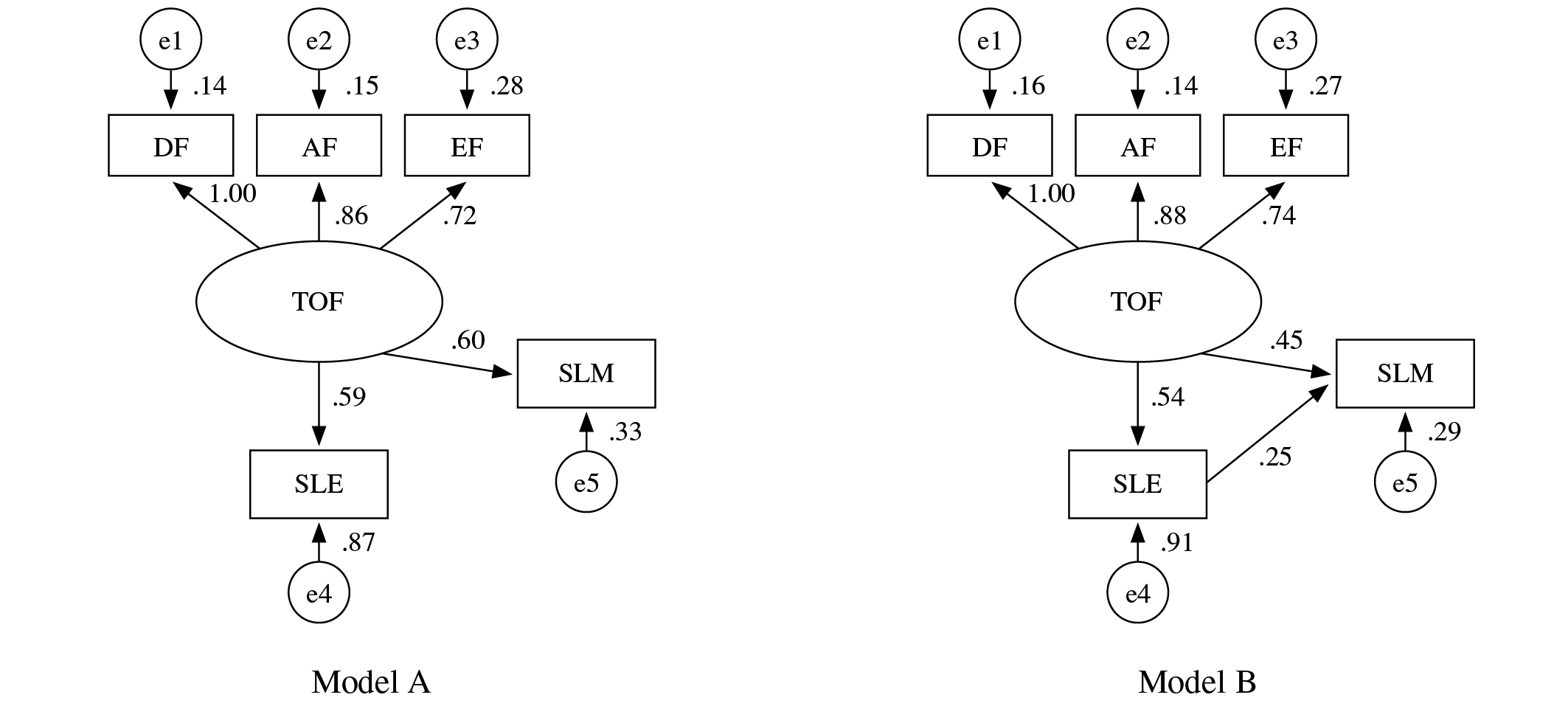
Figure 1. The direct relationship between students’ perceptions of teachers’ online feedback (TOF) and students’ learning motivation (SLM; Model A), and the relationship as mediated by students’ learning engagement (SLE; Model B). DF = developmental feedback, AF = activity-based feedback, EF = evaluative feedback.
All regression weights were significant at p < .001.
Discussion
We explored the correlations between TOF, learning motivation, and learning engagement, and found that TOF was significantly positively correlated with learning motivation and learning engagement. The results also showed that TOF had a significant effect on learning motivation, and that learning engagement partially mediated this relationship. This indicated the value and significance of TOF in improving students’ learning motivation and behavior in an online learning environment (Alvarez, Espasa, & Guasch, 2012; Çakiroğlu & Erdemir, 2019).
Further, we found that students’ perception of TOF was enhanced by their learning engagement, which, in turn, promoted their learning motivation. For example, as the students considered TOF a source of support for their learning, this encouraged them to increase learning engagement. The formation of this close teacher–student relationship accelerated students’ positive cognitive assessment of their learning state (Fredrickson, 1998). In other words, students’ learning was based on not just their engagement, but also positive learning motivation triggered by teachers’ feedback support and social interactive environments. Therefore, this mechanism can contribute to the increase of individual learning motivation.
In addition, the results showed that students’ perception of TOF not only indirectly affected their learning motivation through active learning engagement, but also directly influenced their learning motivation. Students with a high (vs. low) perception of TOF demonstrated more learning engagement, which directly affected their learning motivation. This result is consistent with previous findings that students’ perceived social support is related to their academic performance (Guo & Bian, 2013; Jayarathna, 2014). Therefore, we suggest that future researchers seek to understand the cognitive mechanisms that affect students’ perception of TOF (e.g., learning pressure, students’ emotion, learning motivation), to further understand the whole interactive relationship.
On a practical level, we have three suggestions for strengthening TOF: First, TOF should be timely and sufficient. Teachers should offer online interactive feedback as much as possible, so that language learning students feel that they are receiving support from teachers. Second, teachers should vary the focus and type of feedback by responding to language teaching content, learning tasks, and students’ needs. We found that as students preferred activity-based and developmental feedback, this indicated that they desired online teacher–student interaction, and expected teachers to give developmental feedback and guidance in relation to language-learning strategies. This finding corroborates those of previous researchers who have concluded that feedback should not only include verification of what has been learned but should also aim to promote students’ learning (Alvarez et al., 2012; van der Kleij, Eggen, Timmers, & Veldkamp, 2012). This also illustrates the complex role that the teacher is required to fulfill in online settings (Coll et al., 2014). Finally, language teachers should provide positive feedback as much as possible, enabling students to perceive emotional support from teachers and to improve their online learning engagement and participation enthusiasm. This is consistent with Hattie and Yates’ (2014) recommendation that teachers’ negative feedback may embarrass their students and create an unfavorable learning environment.
There are several limitations in this study. First, although the questionnaire had high reliability and validity, we relied on self-reported data, which may have affected the accuracy of our results. Future researchers can combine classroom observations, interviews, and other research methods to verify our results. Second, as we used a cross-sectional design, this may have led to bias causality results. We recommend that future researchers use a longitudinal design to address possible causal relationships. Future researchers can also replicate this study with a larger sample size in the questionnaire. Third, we focused on the subject matter of English language learning. The key teacher factors that affect students’ learning engagement and learning motivation may differ across different subject matters and grades. Thus, we recommend caution when applying our results to subjects other than English language learning.
References
Alvarez, I., Espasa, A., & Guasch, T. (2012). The value of feedback in improving collaborative writing assignments in an online learning environment. Studies in Higher Education, 37, 387–400.
https://doi.org/10.1080/03075079.2010.510182
Anderson, T., Rourke, L., Garrison, D. R., & Archer, W. (2001). Assessing teaching presence in a computer conferencing context. Journal of Asynchronous Learning Networks, 5(2).
https://doi.org/10.24059/olj.v5i2.1875
Babad, E. (1990). Measuring and changing teachers’ differential behavior as perceived by students and teachers. Journal of Educational Psychology, 82, 683–690.
https://doi.org/10.1037/0022-0663.82.4.683
Bailey, S., Hendricks, S., & Applewhite, S. (2015). Student perspectives of assessment strategies in online courses. Journal of Interactive Online Learning, 13, 112–125. Retrieved from https://bit.ly/2VdM6OI
Baron, R. M., & Kenny, D. A. (1986). The moderator-mediator variable distinction in social psychological research: Conceptual, strategic, and statistical considerations. Journal of Personality and Social Psychology, 51, 1173–1182.
https://doi.org/10.1037/0022-3514.51.6.1173
Çakiroğlu, Ü., & Erdemir, T. (2019). Online project based learning via cloud computing: Exploring roles of instructor and students. Interactive Learning Environments, 27, 547–566.
https://doi.org/10.1080/10494820.2018.1489855
Carmines, E. G., & McIver, J. P. (1981). Analyzing models with unobserved variables: Analysis of covariance structures. In G. W. Bohrnstedt & E. F. Borgatta (Eds.), Social measurement: Current issues (pp. 65–115). Beverly Hills, CA: Sage.
Coll, C., Rochera, M. J., & de Gispert, I. (2014). Supporting online collaborative learning in small groups: Teacher feedback on learning content, academic task and social participation. Computers & Education, 75, 53–64.
https://doi.org/10.1016/j.compedu.2014.01.015
Cunningham, J. (2010). Self-direction: A critical tool in distance learning. Common Ground Journal, 7, 89–100. Retrieved from https://bit.ly/3349tMK
Fredrickson, B. L. (1998). What good are positive emotions? Review of General Psychology, 2, 300–319.
https://doi.org/10.1037/1089-2680.2.3.300
Garrison, D. R., & Anderson, T. (2003). E-learning in the 21st century: A framework for research and practice. London, UK: RoutledgeFalmer.
Guilloteaux, M. J., & Dörnyei, Z. (2008). Motivating language learners: A classroom-oriented investigation of the effects of motivational strategies on student motivation. TESOL Quarterly, 42, 55–77.
https://doi.org/10.1002/j.1545-7249.2008.tb00207.x
Guo, W., & Bian, Y. (2013) Eighth graders’ perceived social support and academic achievement: The mediating effect of academic self-concept [In Chinese]. Journal of Psychological Science, 36, 627–631.
Hattie, J., & Timperley, H. (2007). The power of feedback. Review of Educational Research, 77, 81–112.
https://doi.org/10.3102/003465430298487
Hattie, J. A., & Yates, G. C. (2014). Using feedback to promote learning. In V. A. Benassi, C. E. Overson, & C. M. Hakala (Eds.), Applying science of learning in education: Infusing psychological science into the curriculum (pp. 45–58). Washington, DC: American Psychological Association.
Hew, K. F. (2016). Promoting engagement in online courses: What strategies can we learn from three highly rated MOOCS. British Journal of Educational Technology, 47, 320–341.
https://doi.org/10.1111/bjet.12235
Hibbert, M. (2017). Blurred experiences: The undefined contours of student learning in online environments. Journal of Interactive Online Learning, 15, 92–106. Retrieved from https://bit.ly/2xBrw1G
Jayarathna, L. C. H. (2014). Perceived social support and academic engagement. Kelaniya Journal of Management, 3, 85–92.
https://doi.org/10.4038/kjm.v3i2.7483
Jensen, J. L., Kummer, T. A., & Godoy, P. D. d. M. (2015). Improvements from a flipped classroom may simply be the fruits of active learning. CBE—Life Sciences Education, 14(1), article 5.
https://doi.org/10.1187/cbe.14-08-0129
Jung, Y., & Lee, J. (2018). Learning engagement and persistence in massive open online courses (MOOCS). Computers & Education, 122, 9–22.
https://doi.org/10.1016/j.compedu.2018.02.013
Kearsley, G., & Shneiderman, B. (1998). Engagement theory: A framework for technology-based teaching and learning. Educational Technology, 38, 20–23. Retrieved from https://bit.ly/336cnk5
Kormos, J., & Csizér, K. (2014). The interaction of motivation, self-regulatory strategies, and autonomous learning behavior in different learner groups. TESOL Quarterly, 48, 275–299.
https://doi.org/10.1002/tesq.129
Larmuseau, C., Desmet, P., & Depaepe, F. (2019). Perceptions of instructional quality: Impact on acceptance and use of an online learning environment. Interactive Learning Environments, 27, 953–964.
https://doi.org/10.1080/10494820.2018.1509874
Li, W., & Bai, Y. (2018). How does perceived teacher support for second year junior students affect their academic achievement? Multiple mediating effects analysis based on academic self-efficacy and learning engagement [In Chinese]. Education and Economy, 6, 86–92. Retrieved from https://bit.ly/3bhSQkc
Liu, Y.-H., & Yu, F.-Y. (2019). Supporting active learning and formative evaluation via teaching-by-questioning in classrooms: Design, development, and preliminary evaluation of an online learning system. Interactive Learning Environments, 27, 841–855.
https://doi.org/10.1080/10494820.2018.1489858
Marsh, H. W., Hau, K.-T., & Grayson, D. (2005). Goodness of fit evaluation in structural equation modeling. In A. Maydeu-Olivares & J. J. McArdle (Eds.), Contemporary psychometrics: A festschrift for Roderick P. McDonald (pp. 275–340). Mahwah, NJ: Erlbaum.
Narciss, S. (2013). Designing and evaluating tutorial feedback strategies for digital learning environments on the basis of the interactive tutoring feedback model. Digital Education Review, 23, 7–26. Retrieved from https://bit.ly/39DLGFV
Narciss, S., & Huth, K. (2006). Fostering achievement and motivation with bug-related tutoring feedback in a computer-based training on written subtraction. Learning and Instruction, 16, 310–322.
https://doi.org/10.1016/j.learninstruc.2006.07.003
Nicol, D. J., & Macfarlane-Dick, D. (2006). Formative assessment and self-regulated learning: A model and seven principles of good feedback practice. Studies in Higher Education, 31, 99–218.
https://doi.org/10.1080/03075070600572090
Noroozi, O., Biemans, H., & Mulder, M. (2016). Relations between scripted online peer feedback processes and quality of written argumentative essay. The Internet and Higher Education, 31, 20–31.
https://doi.org/10.1016/j.iheduc.2016.05.002
O’Brien, H. L., & Toms, E. G. (2008). What is user engagement? A conceptual framework for defining user engagement with technology. Journal of the American Society for Information Science and Technology, 59, 938–955.
https://doi.org/10.1002/asi.20801
Ozkara, B. O., & Cakir, H. (2018). Participation in online courses from the students’ perspective. Interactive Learning Environments, 26, 924–942.
https://doi.org/10.1080/10494820.2017.1421562
Price, M., Handley, K., Millar, J., & O’Donovan, B. (2010). Feedback: All that effort, but what is the effect? Assessment & Evaluation in Higher Education, 35, 277–289.
https://doi.org/10.1080/02602930903541007
Ryan, T., Henderson, M., & Phillips, M. (2019). Feedback modes matter: Comparing student perceptions of digital and non-digital feedback modes in higher education. British Journal of Educational Technology, 50, 1507–1523.
https://doi.org/10.1111/bjet.12749
Salmon, G. (2000). E-moderating: The key to teaching and learning online. London, UK: Kogan Page.
Schaufeli, W. B., Martínez, I. M., Pinto, A. M., Salanova, M., & Bakker, A. B. (2002). Burnout and engagement in university students: A cross-national study. Journal of Cross-Cultural Psychology, 33, 464–481.
https://doi.org/10.1177/0022022102033005003
Shute, V. J. (2008). Focus on formative feedback. Review of Educational Research, 78, 153–189.
https://doi.org/10.3102/0034654307313795
Sorensen, E. K., & Takle, E. S. (2005). Investigating knowledge building dialogues in networked communities of practice: A collaborative learning endeavor across cultures. Interactive Educational Multimedia, 10, 50–60. Retrieved from https://bit.ly/3aGHTYA
van der Kleij, F. M., Eggen, T. J. H. M., Timmers, C. F., & Veldkamp, B. P. (2012). Effects of feedback in a computer-based assessment for learning. Computers & Education, 58, 263–272.
https://doi.org/10.1016/j.compedu.2011.07.020
Wood, A. M., Froh, J. J., & Geraghty, A. W. A. (2010). Gratitude and well-being: A review and theoretical integration. Clinical Psychology Review, 30, 890–905.
https://doi.org/10.1016/j.cpr.2010.03.005
Yang, S.-H. (2016). Conceptualizing effective feedback practice through an online community of inquiry. Computers & Education, 94, 162–177.
https://doi.org/10.1016/j.compedu.2015.10.023
Zhu, E. (2006). Interaction and cognitive engagement: An analysis of four asynchronous online discussions. Instructional Science, 34, 451–480.
https://doi.org/10.1007/s11251-006-0004-0
Alvarez, I., Espasa, A., & Guasch, T. (2012). The value of feedback in improving collaborative writing assignments in an online learning environment. Studies in Higher Education, 37, 387–400.
https://doi.org/10.1080/03075079.2010.510182
Anderson, T., Rourke, L., Garrison, D. R., & Archer, W. (2001). Assessing teaching presence in a computer conferencing context. Journal of Asynchronous Learning Networks, 5(2).
https://doi.org/10.24059/olj.v5i2.1875
Babad, E. (1990). Measuring and changing teachers’ differential behavior as perceived by students and teachers. Journal of Educational Psychology, 82, 683–690.
https://doi.org/10.1037/0022-0663.82.4.683
Bailey, S., Hendricks, S., & Applewhite, S. (2015). Student perspectives of assessment strategies in online courses. Journal of Interactive Online Learning, 13, 112–125. Retrieved from https://bit.ly/2VdM6OI
Baron, R. M., & Kenny, D. A. (1986). The moderator-mediator variable distinction in social psychological research: Conceptual, strategic, and statistical considerations. Journal of Personality and Social Psychology, 51, 1173–1182.
https://doi.org/10.1037/0022-3514.51.6.1173
Çakiroğlu, Ü., & Erdemir, T. (2019). Online project based learning via cloud computing: Exploring roles of instructor and students. Interactive Learning Environments, 27, 547–566.
https://doi.org/10.1080/10494820.2018.1489855
Carmines, E. G., & McIver, J. P. (1981). Analyzing models with unobserved variables: Analysis of covariance structures. In G. W. Bohrnstedt & E. F. Borgatta (Eds.), Social measurement: Current issues (pp. 65–115). Beverly Hills, CA: Sage.
Coll, C., Rochera, M. J., & de Gispert, I. (2014). Supporting online collaborative learning in small groups: Teacher feedback on learning content, academic task and social participation. Computers & Education, 75, 53–64.
https://doi.org/10.1016/j.compedu.2014.01.015
Cunningham, J. (2010). Self-direction: A critical tool in distance learning. Common Ground Journal, 7, 89–100. Retrieved from https://bit.ly/3349tMK
Fredrickson, B. L. (1998). What good are positive emotions? Review of General Psychology, 2, 300–319.
https://doi.org/10.1037/1089-2680.2.3.300
Garrison, D. R., & Anderson, T. (2003). E-learning in the 21st century: A framework for research and practice. London, UK: RoutledgeFalmer.
Guilloteaux, M. J., & Dörnyei, Z. (2008). Motivating language learners: A classroom-oriented investigation of the effects of motivational strategies on student motivation. TESOL Quarterly, 42, 55–77.
https://doi.org/10.1002/j.1545-7249.2008.tb00207.x
Guo, W., & Bian, Y. (2013) Eighth graders’ perceived social support and academic achievement: The mediating effect of academic self-concept [In Chinese]. Journal of Psychological Science, 36, 627–631.
Hattie, J., & Timperley, H. (2007). The power of feedback. Review of Educational Research, 77, 81–112.
https://doi.org/10.3102/003465430298487
Hattie, J. A., & Yates, G. C. (2014). Using feedback to promote learning. In V. A. Benassi, C. E. Overson, & C. M. Hakala (Eds.), Applying science of learning in education: Infusing psychological science into the curriculum (pp. 45–58). Washington, DC: American Psychological Association.
Hew, K. F. (2016). Promoting engagement in online courses: What strategies can we learn from three highly rated MOOCS. British Journal of Educational Technology, 47, 320–341.
https://doi.org/10.1111/bjet.12235
Hibbert, M. (2017). Blurred experiences: The undefined contours of student learning in online environments. Journal of Interactive Online Learning, 15, 92–106. Retrieved from https://bit.ly/2xBrw1G
Jayarathna, L. C. H. (2014). Perceived social support and academic engagement. Kelaniya Journal of Management, 3, 85–92.
https://doi.org/10.4038/kjm.v3i2.7483
Jensen, J. L., Kummer, T. A., & Godoy, P. D. d. M. (2015). Improvements from a flipped classroom may simply be the fruits of active learning. CBE—Life Sciences Education, 14(1), article 5.
https://doi.org/10.1187/cbe.14-08-0129
Jung, Y., & Lee, J. (2018). Learning engagement and persistence in massive open online courses (MOOCS). Computers & Education, 122, 9–22.
https://doi.org/10.1016/j.compedu.2018.02.013
Kearsley, G., & Shneiderman, B. (1998). Engagement theory: A framework for technology-based teaching and learning. Educational Technology, 38, 20–23. Retrieved from https://bit.ly/336cnk5
Kormos, J., & Csizér, K. (2014). The interaction of motivation, self-regulatory strategies, and autonomous learning behavior in different learner groups. TESOL Quarterly, 48, 275–299.
https://doi.org/10.1002/tesq.129
Larmuseau, C., Desmet, P., & Depaepe, F. (2019). Perceptions of instructional quality: Impact on acceptance and use of an online learning environment. Interactive Learning Environments, 27, 953–964.
https://doi.org/10.1080/10494820.2018.1509874
Li, W., & Bai, Y. (2018). How does perceived teacher support for second year junior students affect their academic achievement? Multiple mediating effects analysis based on academic self-efficacy and learning engagement [In Chinese]. Education and Economy, 6, 86–92. Retrieved from https://bit.ly/3bhSQkc
Liu, Y.-H., & Yu, F.-Y. (2019). Supporting active learning and formative evaluation via teaching-by-questioning in classrooms: Design, development, and preliminary evaluation of an online learning system. Interactive Learning Environments, 27, 841–855.
https://doi.org/10.1080/10494820.2018.1489858
Marsh, H. W., Hau, K.-T., & Grayson, D. (2005). Goodness of fit evaluation in structural equation modeling. In A. Maydeu-Olivares & J. J. McArdle (Eds.), Contemporary psychometrics: A festschrift for Roderick P. McDonald (pp. 275–340). Mahwah, NJ: Erlbaum.
Narciss, S. (2013). Designing and evaluating tutorial feedback strategies for digital learning environments on the basis of the interactive tutoring feedback model. Digital Education Review, 23, 7–26. Retrieved from https://bit.ly/39DLGFV
Narciss, S., & Huth, K. (2006). Fostering achievement and motivation with bug-related tutoring feedback in a computer-based training on written subtraction. Learning and Instruction, 16, 310–322.
https://doi.org/10.1016/j.learninstruc.2006.07.003
Nicol, D. J., & Macfarlane-Dick, D. (2006). Formative assessment and self-regulated learning: A model and seven principles of good feedback practice. Studies in Higher Education, 31, 99–218.
https://doi.org/10.1080/03075070600572090
Noroozi, O., Biemans, H., & Mulder, M. (2016). Relations between scripted online peer feedback processes and quality of written argumentative essay. The Internet and Higher Education, 31, 20–31.
https://doi.org/10.1016/j.iheduc.2016.05.002
O’Brien, H. L., & Toms, E. G. (2008). What is user engagement? A conceptual framework for defining user engagement with technology. Journal of the American Society for Information Science and Technology, 59, 938–955.
https://doi.org/10.1002/asi.20801
Ozkara, B. O., & Cakir, H. (2018). Participation in online courses from the students’ perspective. Interactive Learning Environments, 26, 924–942.
https://doi.org/10.1080/10494820.2017.1421562
Price, M., Handley, K., Millar, J., & O’Donovan, B. (2010). Feedback: All that effort, but what is the effect? Assessment & Evaluation in Higher Education, 35, 277–289.
https://doi.org/10.1080/02602930903541007
Ryan, T., Henderson, M., & Phillips, M. (2019). Feedback modes matter: Comparing student perceptions of digital and non-digital feedback modes in higher education. British Journal of Educational Technology, 50, 1507–1523.
https://doi.org/10.1111/bjet.12749
Salmon, G. (2000). E-moderating: The key to teaching and learning online. London, UK: Kogan Page.
Schaufeli, W. B., Martínez, I. M., Pinto, A. M., Salanova, M., & Bakker, A. B. (2002). Burnout and engagement in university students: A cross-national study. Journal of Cross-Cultural Psychology, 33, 464–481.
https://doi.org/10.1177/0022022102033005003
Shute, V. J. (2008). Focus on formative feedback. Review of Educational Research, 78, 153–189.
https://doi.org/10.3102/0034654307313795
Sorensen, E. K., & Takle, E. S. (2005). Investigating knowledge building dialogues in networked communities of practice: A collaborative learning endeavor across cultures. Interactive Educational Multimedia, 10, 50–60. Retrieved from https://bit.ly/3aGHTYA
van der Kleij, F. M., Eggen, T. J. H. M., Timmers, C. F., & Veldkamp, B. P. (2012). Effects of feedback in a computer-based assessment for learning. Computers & Education, 58, 263–272.
https://doi.org/10.1016/j.compedu.2011.07.020
Wood, A. M., Froh, J. J., & Geraghty, A. W. A. (2010). Gratitude and well-being: A review and theoretical integration. Clinical Psychology Review, 30, 890–905.
https://doi.org/10.1016/j.cpr.2010.03.005
Yang, S.-H. (2016). Conceptualizing effective feedback practice through an online community of inquiry. Computers & Education, 94, 162–177.
https://doi.org/10.1016/j.compedu.2015.10.023
Zhu, E. (2006). Interaction and cognitive engagement: An analysis of four asynchronous online discussions. Instructional Science, 34, 451–480.
https://doi.org/10.1007/s11251-006-0004-0
Table 1. Means and Standard Deviations of Scores for Study Variables

Note. N = 312.
Table 2. Bivariate Correlations and Internal Consistency Reliability for Study Variables

Note. Cronbach’s α are shown on the diagonal in parentheses.
** p < .01.
Table 3. Fit Indices of the Three Models

Note. N = 312. Model A = direct effect model, Model B = mediated model, Model C = mediated model with the direct path from TOF to learning motivation omitted. χ2 = chi square, df = degrees of freedom, TLI = Tucker–Lewis index, CFI = comparative fit index, RMSEA = root mean square error of approximation, SRMR = standardized root mean square residual.
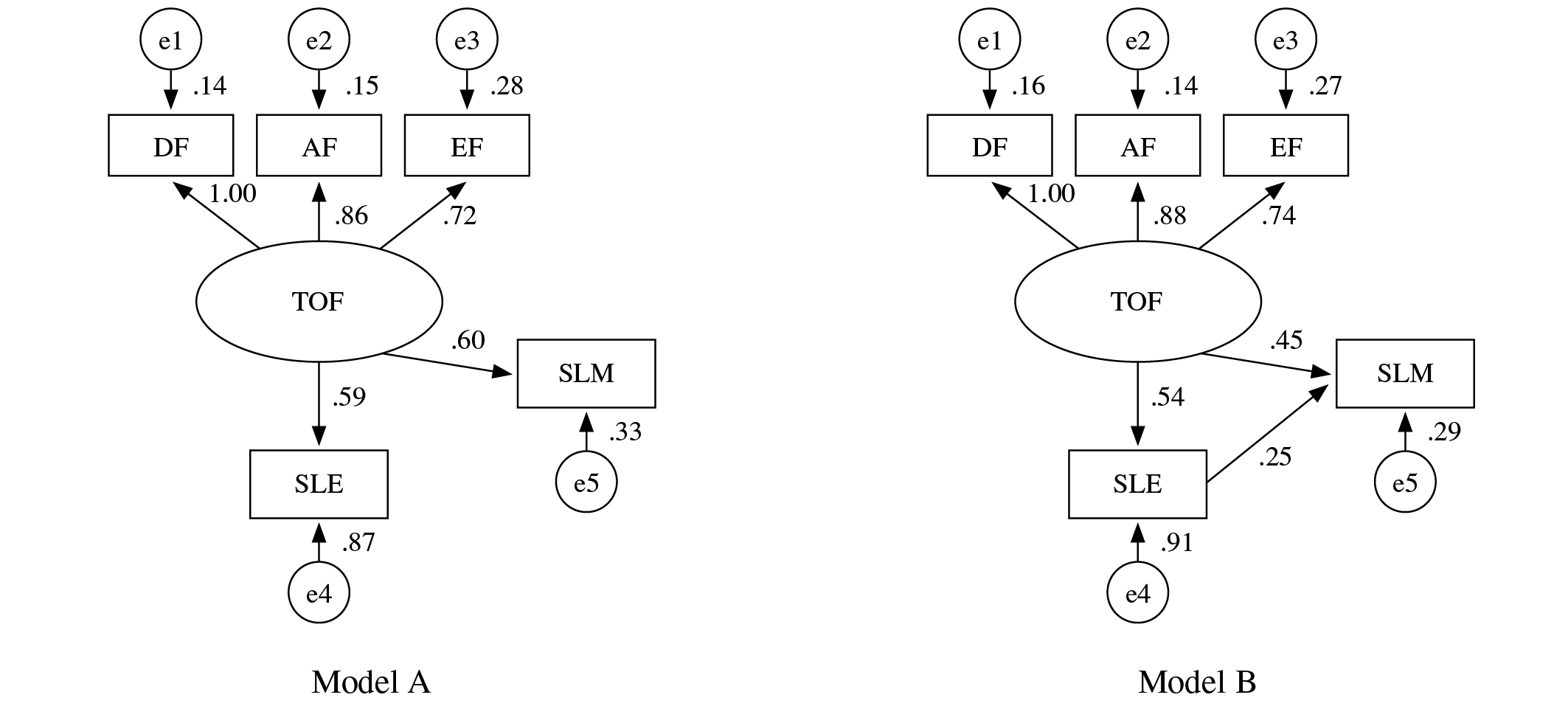
Figure 1. The direct relationship between students’ perceptions of teachers’ online feedback (TOF) and students’ learning motivation (SLM; Model A), and the relationship as mediated by students’ learning engagement (SLE; Model B). DF = developmental feedback, AF = activity-based feedback, EF = evaluative feedback.
All regression weights were significant at p < .001.
This work was supported by a grant from the Department of Education of Zhejiang Province (Y201840805).
Xiaoquan Pan, Xingzhi College, Zhejiang Normal University, 688 Yingbin Road, Jinhua, Zhejiang 321004, People’s Republic of China. Email: [email protected]