Using logistic regression for persona segmentation in tourism: A case study
Main Article Content
I introduced a method for persona segmentation in the tourism industry to identify representative subgroups with different motivations or goals. Data from 496 key opinion leaders of groups representing 7,965 travel service users were analyzed with a logistic regression model of user characteristics and tourism motivation. I found that logistic regression is an integrated method of persona segmentation that balances precision and accuracy, and yields replicable and valid results. Three subgroups for persona segmentation based on logistic regression models are proposed.
The information-intensive nature of tourism and rising demand for information from increasingly sophisticated consumers have brought unprecedented pressure on tourism enterprises. This has required tourism companies to provide high-quality information services to meet current and future tourism industry development needs (Hou & Li, 2011). Online tourism is a new type of tourism business undertaken by the application of technology, such as the Internet and mobile e-commerce, to the tourism industry. Online tourism has improved the quality of tourism services, reduced the cost for tourism enterprises, improved the efficiency of their business operations, and allowed them to maintain their competitiveness. This has had a profound impact on the tourism industry, gaining the interest of researchers (Hou & Li, 2011).
Persona segmentation is the first critical step in persona creation, whereby archetypal users can be determined. Persona creation as a user experience design tool dates back to initial application in information technology systems development (Cooper, 1999), and is useful for service design (Idoughi, Seffah, & Kolski, 2012). However, researchers and practitioners have paid little attention to the persona-based approach in online tourism services because tourists, tourism products, and tourism service types are highly diverse; further, because of professional requirements, the current methods of persona creation are time-consuming, costly, and difficult to use. In addition, travel services are based on stakeholders’ and practitioners’ ideas, and resource accessibility. As online businesses can obtain rich user information on a previously unimaginable scale, persona segmentation has emerged as a challenge to stakeholders and practitioners. Therefore, I aimed to establish a persona segmentation method in the context of online tourism.
Literature Review
Persona Creation
A persona represents an archetypal or representative user of a product, system, or service, and this includes behavioral specifications that embody the characteristics of that user (Calde, Goodwin, & Reimann, 2002; Cooper, Reimann, & Cronin, 2007; Faily & Flechais, 2011). Thus, a persona is a description of a fictional character constructed from questionnaires, in-depth interviews, observations, focus groups, and internal discussions based on existing individuals, and can be used to identify user information needs and characteristics to develop design and marketing strategies.
In general, persona creation comprises two steps: persona segmentation and persona writing. Persona segmentation involves the identification of representative subgroups with different motivations or goals through qualitative and quantitative methods (Laporte, Slegers, & De Grooff, 2012; Tu, He, et al., 2010). Persona writing is the actual illustration of a persona by the use of the segmentation data. Qualitative methods are usually used to obtain precise information.
Precision and accuracy are important dimensions of persona creation. Precision is the ability to obtain detailed information about representative users. Accuracy is the representative users’ needs or motivation, and the relationship between user characteristics and needs. Qualitative methods, however, lack accuracy because they are subjective, and data are obtained from small-sized samples, and quantitative methods lack precision because the user’s goals or requirements are merely categorized. Therefore, researchers have proposed using a combined qualitative and quantitative approach, such as cluster or factor analysis and interviews (McGinn & Kotamraju, 2008). However, the focus in the combined approach currently used is more on the use of quantitative methods to identify representative users’ needs or goals, with reliance on qualitative methods to account for the relationship between needs and characteristics. The resulting accuracy of personas is still insufficient.
Personas in tourism. Compared to traditional tourism marketing, in which marketers rely on the product’s characteristics in their promotions, the basis in current tourism marketing is users’ characteristics and needs. Park, Tussyadiah, Mazanec, and Fesenmaier (2010) used network analysis to examine the personas of American travelers based on their trip portfolio. The analysis generated four personas with significant differences in age, income, primary trip activities, and travel intention. Liu, Wu, Yi, and Fan (2018) conducted an importance–performance analysis to investigate personas of Chinese tourists traveling with their children, and found that the core participants were women aged approximately 30 years. These two studies are examples of the few I identified in my literature review regarding the concept of the persona in tourism, not to mention studies in which a quantitative approach designed to yield an accurate relationship between user characteristics and needs, was used.
Persona creation methods. In general, qualitative and quantitative methods can be employed to gather information for persona development and refinement. Common qualitative methods include direct observation, focus groups, semi-structured interviews, and informal discussion (LeRouge, Ma, Sneha, & Tolle, 2013), case studies (Switzky, 2012), and workshops (Blomquist & Arvola, 2002). Researchers typically identify users’ common needs by coding obtained data and abstracting common demographic characteristics to establish potential personas. However, the qualitative approach has been criticized for its use of small-sized samples, and its difficulty and complexity for researchers, as it relies on their practical experience and professional knowledge of persona establishment, which is not easily disseminated to the designer or practitioner in user-centered design. In addition, qualitative methods are time-consuming and costly in terms of project realization (Pruitt & Adlin, 2006).
To overcome the qualitative approach limitations, researchers have begun using surveys to obtain more user data while employing, for example, factor analysis (McGinn & Kotamraju, 2008), principal components and cluster analysis (Tu, Dong, Rau, & Zhang, 2010), and correspondence analysis (Laporte et al., 2012). To refine user needs, researchers use individual difference statistics based on survey or market research data (Faily & Flechais, 2011; Goodwin & Santilli, 2009). Although these approaches (vs. purely qualitative methods) are faster and more effective, an accurate assessment of the relationship between user characteristics and needs cannot be established. To balance precision and accuracy, my goal was, thus, to provide a quantitative method to enable researchers to obtain more accurate information than was possible with previous methods, regarding the characteristics and needs of a persona in an information-rich domain, such as tourism.
Tourism Motivation: Events and Special Interests
Events and special interests are individuals’ two main motivations for traveling. Events have become a core element of the destination system (Getz, 2008), for example, the annual Melissa’s Road Race held in Banff National Park and the Franco-Albertan Festival held in Jasper National Park (Halpenny, 2008). Planned events play an important role in the development of tourism destinations, increasing their competitiveness and attractiveness (Getz & Page, 2016a). Special interest tourism (SIT) is qualitatively different from other types of tourism because it is designed to meet tourists’ need to engage in behavior that enhances their sense of self (Trauer, 2006). SIT occurs when specific activities or destinations determine tourist motivation and decision making (Weiler & Hall, 1992). Special interest tourists are motivated by an existing or new interest (Swarbrooke & Horner, 1999), such as “recreational travel undertaken to remote or exotic destinations for the purpose of exploration or engaging in a variety of rugged activities” (Drita & Albana, 2011, p. 80). Popular SIT activities include adventure tourism, rural tourism, cultural tourism, religious tourism, culinary tourism, wildlife tourism, heritage tourism, and ecotourism. Although event tourism (Getz, 2008) and SIT (Trauer, 2006) were both developed more than a decade ago, tourism researchers have not yet examined the relationship between tourists’ characteristics and these two types of tourism. In general, the characteristics of event tourism and SIT have been examined separately.
Characteristics of event tourists. Event tourism researchers have focused on several important topics and trends: (a) planning, management, and impact of single events (Tkaczynski, 2013); (b) in regard to how planned events can change a destination’s image, event tourism is attracting attention from researchers and theorists (Ziakas, 2013); (c) interdisciplinary studies on event tourism are becoming prevalent (Weed, 2012); and (d) enhancement of tourism destination image and cobranding roles for events have become valued (Karadakis, Kaplanidou, & Karlis, 2010) because the motivator for event tourism users is mainly destination attractiveness, eclipsing seasonality.
To the best of my knowledge, few researchers have explored tourist characteristics in the event tourism context. The exceptions are a case study of two events by Halpenny (2008), in which the tourist characteristics of age, gender, annual household income, education level, and frequency of travel were described, and the tourists’ travel plans and recommendations, travel patterns, and event management preferences were analyzed, and a qualitative study by Chen (2010), in which gender differences in sport event tourism were investigated according to user loyalty, socialization, self-actualization, volunteering, and equality through sport. Chen’s results indicate that women (vs. men) score higher on these attributes.
Characteristics of special interest tourists. Current foci in SIT research are the exploration of specific types of SIT, the development of SIT in a specific area or country, the characteristics of special interest tourists, and the influence of organizations or governments on SIT. Specifically, (a) religious tourism (Gil & de Esteban Curiel, 2008), adventure tourism (Trauer, 2006), and wine tourism (Carmichael, 2005) have attracted academic attention; (b) many researchers have focused on the development of SIT in specific geographic areas, including Spain (Gil & de Esteban Curiel, 2008), Macao (Wang & Meng, 2016), Canada (Carmichael, 2005), and Australia (Moscardo, McCarthy, Murphy, & Pearce, 2009); (c) several researchers have also considered the characteristics of special interest tourists. For example, Sheng and Chen (2012) investigated the experience expectations of museum visitors, and Sheng, Shen, and Chen (2008) explored the demographic features of special interest tourists; and (d) researchers have also paid attention to organizational influences, such as government public marketing and the customer satisfaction relationship with SIT (Wang & Meng, 2016).
Trauer (2006) proposed two dimensions for conceptualizing the SIT user experience: enduring involvement and frequency of SIT product purchase. In addition, four types of special interest tourists have been identified according to their characteristics. Sheng et al. (2008) used a questionnaire to explore the relationship between special interest tour preference and tourists’ demographic characteristics. Tour preference was found to have a significant relationship with participants’ age, gender, marital status, and other demographic variables.
In summary, the demographic variables of age, gender, annual household income, education level, marital status, and frequency of travel are the most relevant user characteristics for researchers who are examining event tourism and SIT. Accordingly, I selected and encoded these variables as related to user characteristics.
Event tourism and SIT have not been addressed empirically in the same framework, and few researchers have explored the persona concept in the tourism context. In China, group tours are an important mode of travel. Decisions about travel themes, destinations, and average price per person, therefore, often depend on one or two people, who may also influence their friends, neighbors, coworkers, or even experts, celebrities, and other personalities. These decision makers and influencers are known as key opinion leaders (KOLs). In marketing, the concept of opinion leadership originated from the diffusion of innovations theory, which describes how individuals indirectly alter others’ attitudes and behavior through social influence (Rogers, 1995). I have used this concept to describe people in group tourism who have the power to make the final decision on travel plans, including core travel feature components.
Traditional or general persona methodologies lack accuracy regarding the relationship between users’ demographics and their needs. The use of logistic regression allows for describing the data and explaining the relationship between one dependent binary variable and one or more nominal, ordinal, interval, or ratio-level independent variables, and determining the relative weights of the independent variables in terms of their effect on the dependent variable. Therefore, I queried if the use of logistic regression (vs. traditional or general methodologies) can provide more accurate and integrated user information for persona segmentation. My second research objective was to explore the differences in the characteristics of users of events and SIT services based on KOL user data.
Method
Logistic Regression Persona Segmentation Approach
I proposed the following six steps for logistic regression persona segmentation.
Step 1: Identify goals or motivations and characteristics of the users of a target service, using a literature review. User motivation is often defined as a dependent variable, and user characteristics are usually independent variables.
Step 2: Determine the data source in the analysis: Where will the data come from? Will the data include lead users, ordinary users, and/or potential users?
Step 3: Encode the categorical independent variables in terms of previous studies or actual requirements, to test the differences between group means for each variable when the regression model is being used.
Step 4: Transform each type of user motivation into a binary dependent variable. The value is labeled as 1 if the user has the corresponding motivation and zero, otherwise.
Step 5: Analyze the transformed data through a logistic regression model, compare the resulting models for each motivation, and determine the final models to obtain the respective subgroups.
Step 6: Interpret the final models based on Wald test results, and describe the subgroups.
Data Collection
The data that I used are part of an overseas tourism dataset compiled by a Chinese company providing e-tourism services and customizing trips according to Chinese user needs. The data included information about tourism projects and demographic information. When travelers intend to use the online tourism service, they fill in their own demographic information and relevant travel experience. The online tourism company staff supplement this with relevant information as part of the subsequent online service.
I combined stratified and random sampling methods to select data from July 2017 to April 2018. Tourism information included travel time, travel destination, and travel type. Travel time included typical months in the tourism off-season and busy season, and the proportion of busy season travel time was 47.1%. Travel destinations included America (42.9%), Europe (22.8%), Oceania (17.7%), Asia (10.3%), Africa (4.0%), Antarctica (1.8%), and Arctica (0.4%). To analyze the travelers’ motivation, I focused on two travel types: event tourism (30.4%) and SIT (69.6%). The activity types for event tourism were education (including educational experiences, 26.5% and parent–child study, 9.9%), business (including business visit/business, 23.8% and participation in activities/business, 6.6%), participation in activities/fashion (6.6%) or activities/sport (2.0%), medical care (21.9%), and honeymoon (2.6%). The activity types for SIT were family leisure and natural scenery (50.1%), culture (26.7%), and special interest experience (23.2%).
Participants
I selected data for 496 KOLs who represented 7,965 users. The size of the groups represented by each KOL ranged from 1 to 100 (M = 16.10, SD = 15.96). The travel cost per person ranged from US$904.71 to US$178,571.43 (M = 18,291.36, SD = 22,498.00). Ages ranged from 16 to 54 years (M = 35.90, SD = 10.45). There were 316 men (63.7%) and 180 women (36.3%). The highest educational level was a bachelor’s degree (53.6% of the dataset), with undergraduates accounting for 16.7%, and below undergraduate level accounting for 15.7%. There were 218 (44%) business owners, 233 (47%) senior executives, 33 (6.7%) students, and a small group of civil servants and retirees (2.4%). In terms of marital status and children, 34.5% were married, 6.5% were single, 1.2% were divorced (overall, 57.9% of values were missing for this variable), and 71.8% of users had at least one child. In terms of annual income, 36.9% reported over US$1.43 million, 31.0% over US$0.14 million but less than US$1.43 million, and 9.9% less than US$0.14 million (22.2% did not report their income). In terms of home area, 241 (48.6%) users lived in South China and 255 (51.4%) lived in North China. Finally, 98.0% of users had previously traveled abroad, and 53.6% had not previously been to their proposed destination.
Data Analysis
I used SPSS 22.0 to compute the descriptive statistics of the following demographics of KOLs for event tourism and SIT: gender, age, educational level, occupation, annual income, hometown, marital status, parental status, group size, and average cost per person. The relationship between the KOL demographic characteristics and event tourism/SIT was analyzed with an analysis of variance/chi-square test and logistic regression.
Orthogonal coding of the independent variables representing user characteristics in the logistic regression is presented in Table 1. For example, age was categorized into four groups, representing “under 23 years,” “23–28 years,” “29–35 years,” and “over 35 years.” These can be transformed into three new variables, that is, age Vector 1 (V1), age Vector 2 (V2), and age Vector 3 (V3). Age V1 (3, -1, -1, -1) represents the comparison between the group aged under 23 years and the average of the other groups, age V2 (2, 0, -1, -1) represents the comparison between the group aged under 23 years, the average of the groups aged between 29–35 years and over 35 years, and age V3 (0, 0, 1, -1) represents the comparison between the groups aged between 29–35 years and over 35 years. The number of comparisons will be the number of categories minus 1. Researchers can define any comparisons of interest, provided they verify that the comparison or contrasts are orthogonal to one another (von Eye & Schuster, 1998). If the dot product of each pair of vectors is zero, the comparisons or contrasts are orthogonal.
Table 1. Orthogonal Coding of the Independent Variables

Results
Test for Differences in Travel Motivation According to Tourists’ Characteristics
There were 151 KOLs for event tourism and 345 for SIT. Because of the age, group size, and traveling costs per person, standard deviations were relatively large. Thus, I divided the variables into groups to analyze the relationship between user characteristics and motivation. For instance, the correlation between the raw data on group size and tourism motivation was .058 (p > .05), but it rose to .131 (p < .01) after the group size variable was divided into two groups. For a more representative and precise persona segmentation, I divided age, group size, and traveling costs per person into nominal variables for further analysis. I computed the descriptive statistics for tourist characteristics in event tourism and SIT in addition to their correlations with tourism motivation using either a chi-square test or analysis of variance (see Table 2).
Table 2. Characteristics of Tourists in Event and Special Interest Tourism
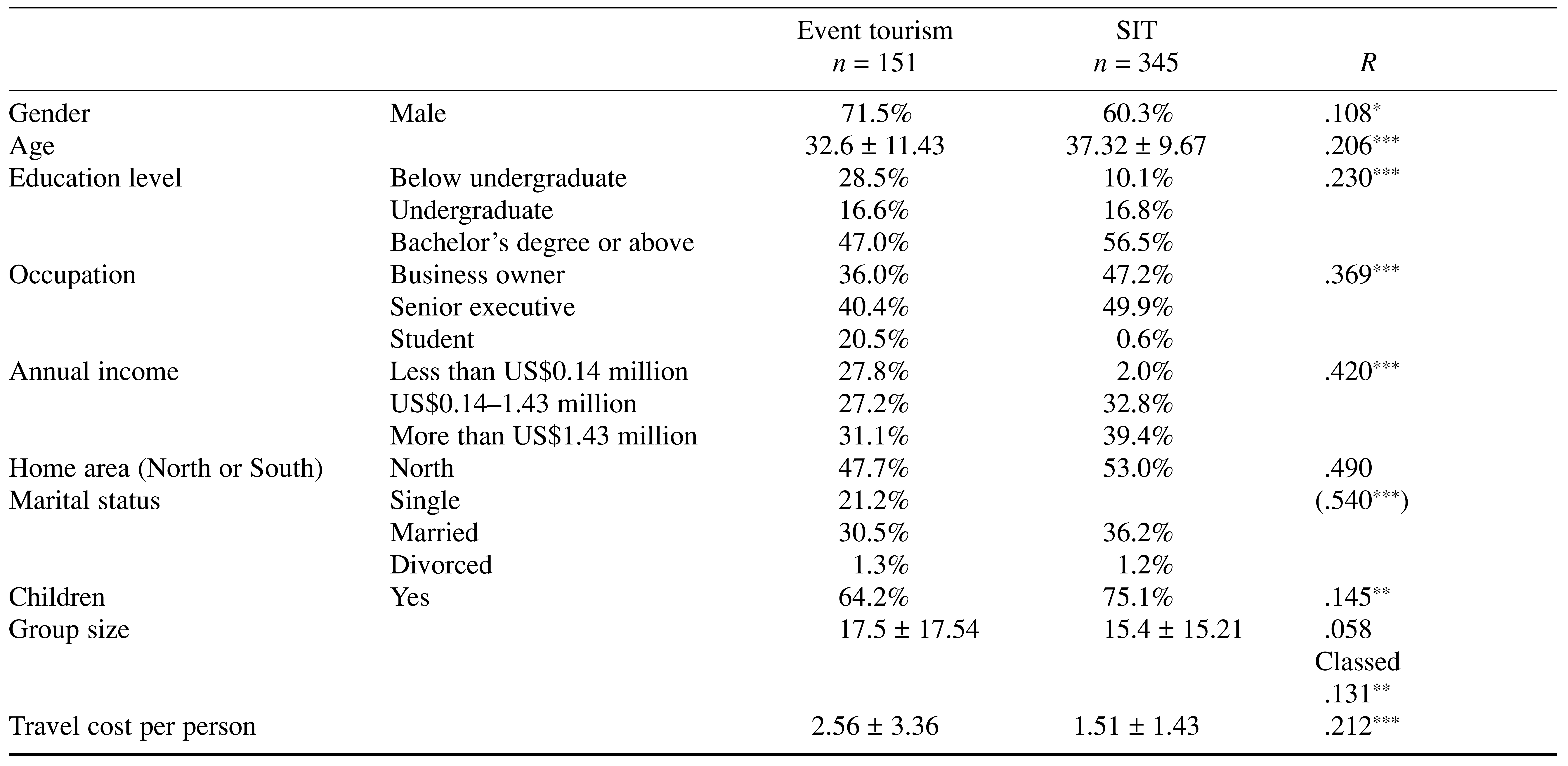
Note. SIT = special interest tourism. Age was measured in years and the unit of the average travel cost per person was US$10,000.
* p < .05, ** p < .01, *** p < .001.
I first explored the correlations between user characteristics and travel motivation. Except for marital status, the descriptive statistics for the chi-square test on motivation and other user characteristics show that the expected frequency was above 5, indicating that it was appropriate to use this test. Marital status was not suitable for the chi-square test because of missing values. Descriptive statistics for marital status show that differences in motivation existed for the single group, but not for the married and divorced groups. The characteristics of the single group are reflected in the model with occupation and parental status variables, meaning that marriage could be deleted from the difference test and logistic regression model.
According to the chi-square test results, gender was significantly related to tourism motivation (χ2 = 5.732, p < .05). Thus, tourism motivation differed by gender with a relatively small correlation coefficient (Cramer’s V = .108). Further pairwise comparisons show that men were more likely than women were to prefer event tourism (34.2% vs. 23.9%, p < .05), and women were more likely than men were to prefer SIT (65.8% vs. 76.1%, p < .05).
Age and travel motivation were also correlated with each other (χ2 = 39.391, p < .001). Age differences emerged in travel motivation with a moderate correlation coefficient (Cramer’s V = .282). The results of further pairwise comparisons and adjusted residual errors show that users aged under 23 years (vs. the other age groups) were more likely to prefer event tourism. The absolute value of the adjusted residual error was 6.2 > 3, 62.3% (aged under 23 years) versus 24.6% (23–28 years), 29.9% (29–35 years), 24.2% (aged over 35 years; p < .05). The group aged under 23 years was also less likely to choose SIT. There were no statistically significant differences between the other three age groups. However, the adjusted standard residual errors indicate that, relatively, those aged over 35 years preferred SIT (3.5 > 3).
Educational level and travel motivation were correlated with each other (χ2 = 22.491, p < .001). Users had different travel motivations according to educational level, with a moderate correlation coefficient (Cramer’s V = .230). The results of further pairwise comparison and adjusted residual errors show that users with an education below the undergraduate level were more likely to choose event tourism than were those with an undergraduate education or higher educational qualification (55.1% vs. 30.1% and 26.7%, p < .05). There were no significant differences for travel motivation between undergraduates and those with a bachelor’s degree or higher level of education. However, the adjusted standard residual errors indicate that users with a bachelor’s degree or higher level of education (vs. undergraduates) were more likely to choose SIT (3.3 > 3).
Occupation and travel motivation were correlated with each other (χ2 = 67.677, p < .001). Travel motivation differed according to occupation, with a moderate correlation coefficient (Cramer’s V = .369). The results of further pairwise comparisons and adjusted residual errors show that student users were more likely to choose event tourism than were users with other occupations, 93.9% (students) versus 25.2% (business owners), 26.2% (senior executives), 33.3% (civil servants and retirees; p < .05). Although there were no differences between the other three groups, the adjusted standard residual errors indicate that business owners preferred SIT to event tourism (2.2 > 1.96).
Income was related to travel motivation (χ2 = 68.070, p < .001). There were motivational differences between user groups according to income level, with a moderate correlation coefficient (Cramer’s V = .420). Further pairwise comparisons and adjusted residual errors show that users who earned less than US$0.14 million annually were more likely to choose event tourism than were those who earned more than US$0.14 million, 85.7% (less than US$0.14 million) versus 26.6% (over US$0.14 million but less than US$1.43 million), 25.7% (over US$1.43 million; p < .05). There was no significant difference between the other two income groups (over US$0.14 million but less than US$1.43 million, and over US$1.43 million). The adjusted standard residual errors indicate that these two groups were more likely to choose SIT (2.4, 3.2 > 1.96).
Having at least one child was related to travel motivation (χ2 = 9.067, p < .001). Travel motivation differed according to whether users had children, with a weak correlation coefficient (Cramer’s V = .145). The results of further pairwise comparison and adjusted residual errors show that users with (vs. without) children were more likely to choose SIT (72.8% vs. 55.3%, p < .05), and users without children preferred event tourism to SIT (44.7% vs. 27.2%, p < .05).
The KOLs’ group size was related to travel motivation (χ2 = 8.530, p < .001), with a weak correlation coefficient (Cramer’s V = .131). The results of further pairwise comparison and adjusted residual errors show that 72.5% of KOLs in smaller groups and 27.5% in larger groups preferred SIT, whereas 57% in smaller groups and 43% in larger groups preferred event tourism (p < .05).
The travel cost per person was related to travel motivation (χ2 = 18.449, p < .001), with a weak correlation coefficient (Cramer’s V = .193). The results of further pairwise comparison and adjusted residual errors show that users whose travel expenses were below US$14,286 had different travel motivations from those whose travel expenses were in the range of US$14,286–28,571, or more than US$28,571 (event tourism motivation: below US$14,286 group = 30.5%; US$14,286–US$28,571 group = 18.5%; more than US$28,571 group = 49.3%; p < .05). The adjusted standard residual errors indicate that users whose travel expenses were below US$14,286 did not have an obvious preference between event tourism and SIT, but the other two groups showed significant differences (-3.0, 3.6 > 3). The results of further pairwise comparison and adjusted residual errors show that 49.3% of users whose expenses were in the range of US$14,286–28,571 preferred event tourism, whereas 18.5% preferred SIT, and 50.7% of users who spent more than US$28,571 preferred event tourism, whereas 81.5% preferred SIT.
There was no significant correlation between home area (Southern or Northern China) and travel motivation.
Relationship Between User Characteristics and Travel Motivation Based on Logistic Regression Modeling
Event tourism was labeled “1” in my model. The correlational matrix of independent variables was computed to select the variables for the logistic regression (see Table 3). High correlations between two independent variables mean that they can replace each other in the equation.
Table 3. Correlation Matrix of the Independent Variables
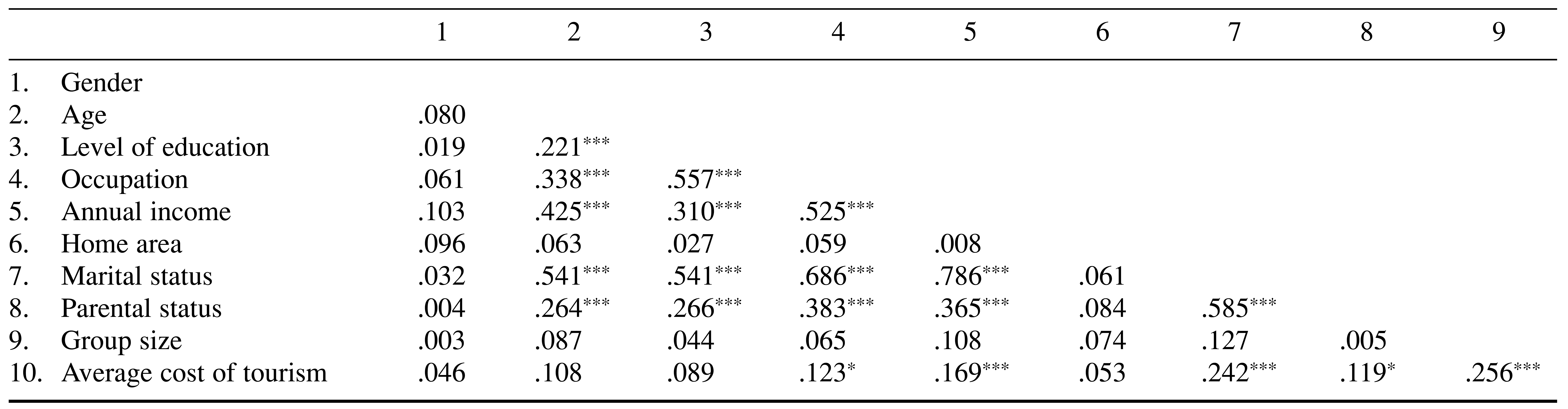
Note. * p < .05, *** p < .001.
In Model 1 I selected all independent variables to explore which ones influenced user motivation. The results show that the model fit was good (χ2 = 82.247, p < .001); these factors influenced the choice of type of travel motivation. As the Hosmer–Lemeshow goodness-of-fit test result shows that χ2 was 3.687 (p > .05), the data information was fully extracted. The prediction accuracy of the model was 75.70% (R2 = .245). The Wald test result shows that the effects of gender, annual income, group size, and average cost of tourism on event tourism were significant after all variables, except marital status, had been introduced into the regression. Men with a low income, traveling in large groups, and with a higher budget were twice as likely as women with a high income, traveling in small groups, and with a lower budget to choose event tourism. Women with an income above US$0.14 million, traveling in small groups, and with a medium budget were more likely to choose SIT.
Table 4. Results of Model 1
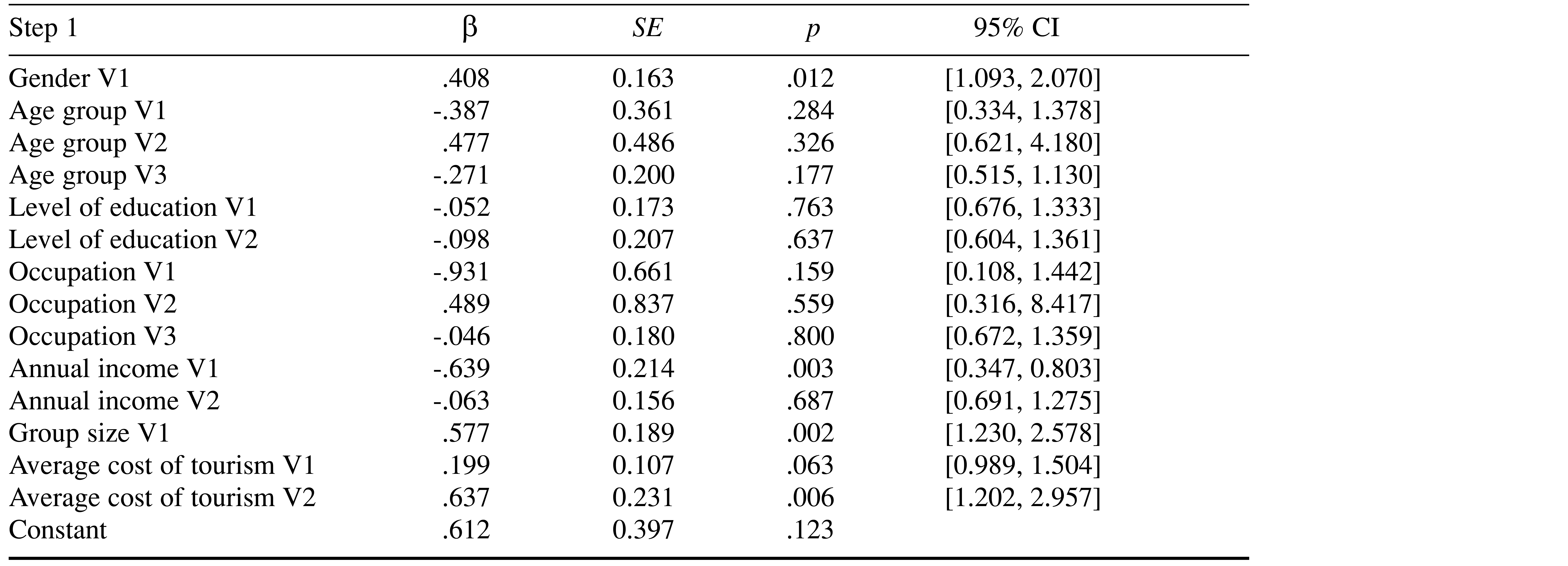
Note. V1 = Vector 1, V2 = Vector 2, V3 = Vector 3, CI = confidence interval.
To obtain a simplified and interpretable model, I formulated several models based on the correlation matrix and difference test. I therefore proposed Models 2, 3, and 4 (see below). The annual income variable in Model 2 was removed, and age, occupation, and educational level were included because annual income was highly correlated with all of these variables. The parental status and home area variables showed no significant differences and were removed. In Model 2 the χ2 was 102.307 (p < .001), indicating that these factors influenced the type of travel motivation. The Hosmer–Lemeshow test result shows that χ2 was 3.144 (p > .05). The data information was, thus, fully extracted. The prediction accuracy was 74.90% (R2 = .297). The Wald test result shows that the effects of age, gender, group size, and average cost of tourism per person on event tourism were significant. The preference for event tourism was higher for men, especially those aged 29 to 35 years, traveling with a larger group, and with a budget per person that exceeded US$28,571.
Table 5. Results of Model 2
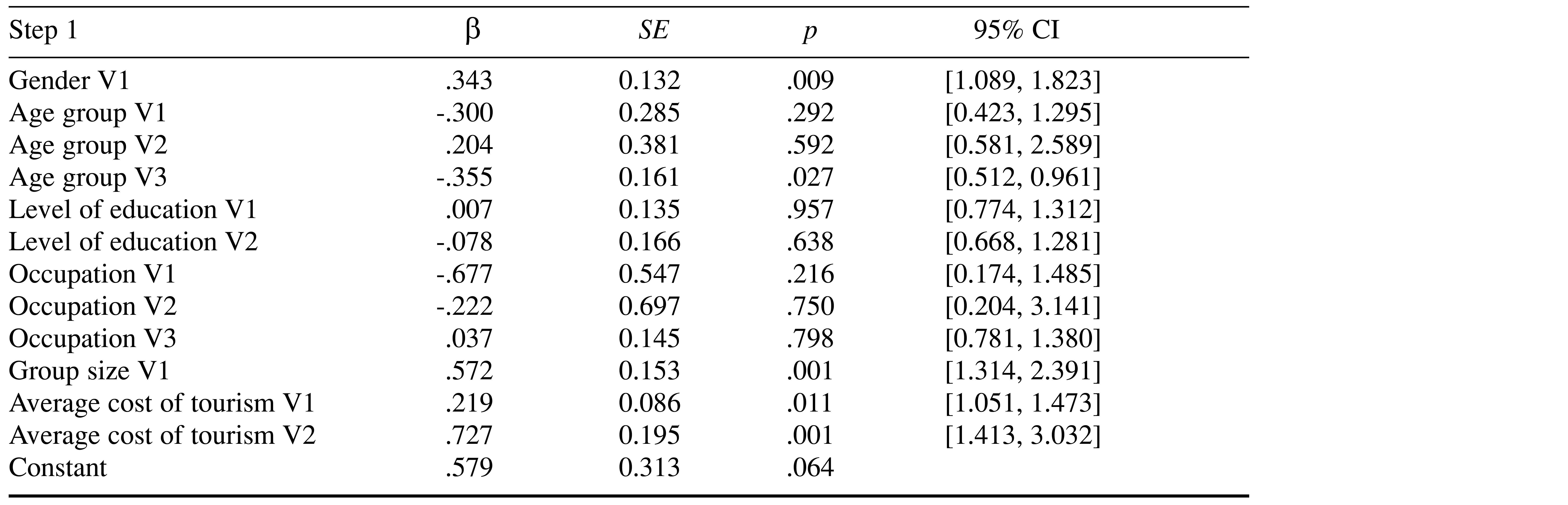
Note. V1 = Vector 1, V2 = Vector 2, V3 = Vector 3, CI = confidence interval.
Table 6. Results of Model 3
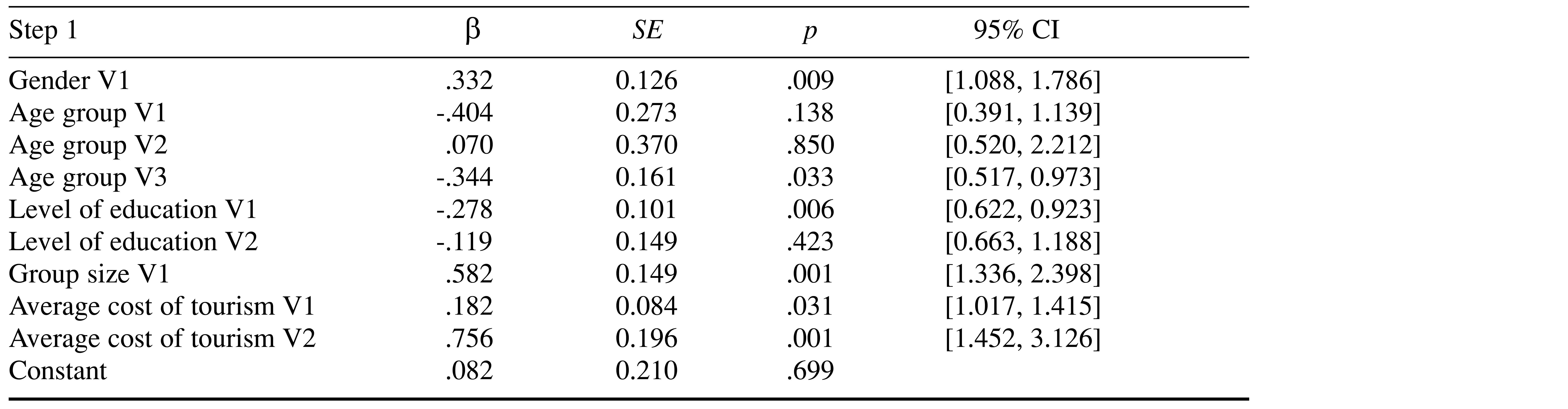
Note. V1 = Vector 1, V2 = Vector 2, V3 = Vector 3, CI = confidence interval.
In Model 3 I removed the occupation variable because the Model 2 results show it was related to age and educational level. The model fit results show that χ2 was 80.738 (p < .001). These factors influenced the type of travel motivation. As the Hosmer–Lemeshow test result shows that χ2 was 7.119 (p > .05), the data information was fully extracted. The prediction accuracy was 74.90% (R2 = .240). The Wald test result shows that the effects of age, gender, educational level, group size, and travel cost per person on event tourism were significant. The preference for event tourism was higher for men aged 35 years and over, with an educational level below undergraduate, traveling with a larger group, and with a high travel budget per person that was above US$28,571.
I removed annual income and included occupation in Model 4, on the basis of the Model 1 results. Parental status and home area were also removed because they showed no significant differences in the choice of event tourism. The results for the model fit show that χ2 was 101.458 (p < .001). These factors influenced the type of travel motivation. As the Hosmer–Lemeshow test result shows that χ2 was 6.082 (p > .05), the data information was fully extracted. The prediction accuracy was 74.60% (R2 = .261). The Wald test result shows that gender, group size, and travel cost per person had significant effects on the choice of event tourism, but occupation did not. Model 4 did not improve upon Model 1 because the annual income variable, which comprises data that are private and difficult to collect, was removed in Model 4. In comparison with Model 1 with all the variables, Model 4 was more concise, but the prediction accuracy did not improve, and the occupational variable was nonsignificant. The preference for event tourism was higher for men, users traveling with a larger group, and those with a travel cost per person that was above US$28,571.
Table 7. Results of Model 4

Note. V1 = Vector 1, V2 = Vector 2, CI = confidence interval.
By comparing the R2 values and considering predictive accuracy, parsimony, and theoretical feasibility, Model 2 is the most appropriate for the tourism services sector. Model 3 does not contain personal privacy information, such as occupation; thus, it is more suitable for conducting long-term tourism customer management. However, more targeted services can be provided by Model 2, by considering the user’s occupation.
Description of Subgroups for Event and Special Interest Tourism
To balance precision and accuracy, I chose Model 2, which extends Model 1, for persona segmentation. The final persona segmentation, based on Models 1 and 2 and company information, therefore describes three subgroups of high-end tourists:
a) KOL event-driven, midlevel male manager, with a moderate income of more than US$0.14 million; aged over 35 years; representing a group of more than 20 people in booking event-based travel; and with a travel cost per person of more than US$28,571. This KOL may work in a large company and be responsible for arranging business travel.
b) KOL SIT-driven, high-income woman: income of more than US$0.14 million; aged 29–35 years; representing a small group of fewer than 20 people; booking SIT and accepting a cost per person of less than US$14,286. This KOL may be an executive in a large company who travels to learn about history and culture, relax, and enjoy hobbies with friends/family.
c) KOL event/SIT-driven: This may be a student booking educational event-based travel or cultural, special interest-based travel. These KOLs may be women with one or more children.
Discussion
I introduced a method for persona segmentation in the tourism industry and found that a KOL’s annual income may be related to travel motivation in high-end tourism groups, and the weight of income may be greater than that of age and gender. Although a user’s income is private information, occupational information can provide an indication of the individual’s income level. Further, in contrast to the researcher-constructed data used in traditional qualitative and quantitative methods of persona segmentation, I used real-world objective data for logistic regression. The results are easily communicated to stakeholders, designers, and marketers of tourism products and services.
Differences in Tourist Characteristics for Event and Special Interest Tourism
I found statistically significant differences according to gender, age, educational level, occupation, annual income, parental status, group size, and average tourism cost per person regarding preference for event tourism and SIT. Of these variables, age, occupation, and annual income showed the largest differences regarding preference for type of tourism. The factors relate to the notion of persona in tourism, that is, gender, age, and income were the same as those identified in a study on event tourism based on a qualitative method (Halpenny, 2008) and in a study on SIT based on a quantitative method (Sheng et al., 2008). However, these previous researchers focused less on general tourism motivation than on specific preferences, such as types of interest tourism, parent–child travel, and sport event tourism. Park et al. (2010) examined general personas in tourism but did not consider user needs and their relationship with demographic characteristics. I used a more general framework in which I integrated tourist needs. I found that men were more likely to choose event tourism and women were more likely to choose SIT. Single (vs. married) users and those with less than an undergraduate level of education (vs. those with a higher level of education) were also more likely to choose event tourism. Event tourism (vs. SIT) was more likely to be undertaken by a larger group. Students were also more likely to choose event tourism than they were to choose SIT. Users with a lower (vs. higher) income were more likely to choose event tourism.
The logistic regression results also indicate that gender, age, income, group size, and travel cost per person together could quite possibly predict the likelihood of a user choosing event tourism over SIT. A male KOL, aged over 35 years, in a larger group, and with a higher travel cost per person would be more likely to choose event tourism over SIT. I have described two typical subgroups in the Results section: a KOL who is an event-driven, male, middle manager and a KOL who is an SIT-driven woman with a high income. These findings are consistent with those of previous studies in which the relationship between event tourism and career path has been emphasized (see, e.g., Getz & Page, 2016b). These results indicate that the average travel cost of event tourism would be more than that of SIT. Group size and age were a second predictable factor. The results show that a KOL may be a male middle manager. A female KOL aged 29–35 years, with a small group size and medium travel cost per person, would likely choose SIT. This may be because it involves relaxation travel and parent–child travel (Liu et al., 2018).
The effect of education level that I found is different from Sheng et al.'s (2008) findings related to SIT in their study conducted using a quantitative method. This can be attributed to the motivation range because, whereas Sheng et al. considered only SIT, I considered a broad motivation framework.
Advantages of Persona Segmentation Based on the Logistic Regression Model
Like other quantitative methods, such as factor analysis (McGinn & Kotamraju, 2008), cluster analysis (Tu, Dong, et al., 2010), and correspondence analysis (Laporte et al., 2012), the logistic regression model can handle a large sample of users (using machine learning with logistic regression) and select a representative user, because the statistical relationships between multiple user characteristics and motivation can be managed simultaneously. This means that not only are categories constructed, but predictions are also developed. Therefore, precision and accuracy can be balanced during persona segmentation with the method that I used.
Because the data collection is not based on surveys, interviews, or other material gathered by professionals, it is less costly and time-consuming than traditional methods. Besides its ease of application, the logistic regression model for persona segmentation is replicable and has good ecological validity because real-world objective data are used, based on established statistical relationships and a simplified model that is essential for business innovation. The model variables are determined based on the literature and on objective real characteristics, as well as the quantitative motivation category. This setup makes the model more reliable than traditional methods.
The companies collecting data in this study were online travel companies providing mid- and high-end travel services. However, a limitation in this study is that the sample data did not include the white collar occupation, which is also one of the main branches of middle- and high-end tourism services. Future researchers could include data from this group to improve the model. In addition, as I investigated only one trip per KOL, future researchers could investigate multiple trips per KOL.
In summary, compared with traditional methods, logistic regression is a more robust method of persona segmentation compared to statistical difference tests because it can provide more specific persona information on user characteristics.
Conclusion
The two main contributions of this study are as follows: First, logistic regression can be effectively used as an integrated method for persona segmentation that balances precision and accuracy because it includes the relationship between user characteristics and needs. This relationship cannot be established by traditional methods. Moreover, the logistic regression framework provides results that are replicable and have good ecological validity owing to the use of objective real data. Second, I have proposed three subgroups for persona segmentation based on logistic regression models and, with the aid of these subgroups, provided a thorough characterization of the most common tourist profiles in the Chinese travel market.
Online travel companies can offer different travel planning schemes to existing or potential customers, according to different types of travel motivation that correspond to customer persona characteristics. In this way, companies can more accurately recommend and tailor customers’ personalized travel plans according to their needs. This should not only improve user experience and user stickiness, but also increase customers’ buying behavior, which is a win–win situation for both customers and online travel companies.
References
Blomquist, Å., & Arvola, M. (2002, October). Personas in action: Ethnography in an interaction design team. Paper presented at the Second Nordic Conference on Human-Computer Interaction, Aarhus, Denmark.
https://doi.org/10.1145/572020.572044
Calde, S., Goodwin, K., & Reimann, R. (2002, April). SHS Orcas: The first integrated information system for long-term healthcare facility management. Paper presented at Case Studies of the CHI2002/AIGA Experience Design FORUM, Minneapolis, MN.
https://doi.org/10.1145/507752.507753
Carmichael, B. (2005). Understanding the wine tourism experience for winery visitors in the Niagara Region, ONT, Canada. Tourism Geographies, 7, 185–204.
https://doi.org/10.1080/14616680500072414
Chen, P.-J. (2010). Differences between male and female sport event tourists: A qualitative study. International Journal of Hospitality Management, 29, 277–290.
https://doi.org/10.1016/j.ijhm.2009.10.007
Cooper, A. (1999). The inmates are running the asylum: Why high tech products drive us crazy and how to restore the sanity. Indianapolis, IN: Macmillan.
Cooper, A., Reimann, R., & Cronin, D. (2007). About Face 3: The essentials of interaction design (3rd ed.). Indianapolis, IN: Wiley.
Drita, K., & Albana, G. (2011). The special interest tourism development and the small regions. Turizam, 15, 77–89.
https://doi.org/10.5937/Turizam1102077K
Faily, S., & Flechais, I. (2011, May). Persona cases: A technique for grounding personas. Paper presented at the SIGCHI Conference on Human Factors in Computing Systems, Vancouver, BC, Canada.
https://doi.org/10.1145/1978942.1979274
Getz, D. (2008). Event tourism: Definition, evolution, and research. Tourism Management, 29, 403–428.
https://doi.org/10.1016/j.tourman.2007.07.017
Getz, D., & Page, S. (2016a). Event studies: Theory, research and policy for planned events (3rd ed.). London, UK: Routledge.
Getz, D., & Page, S. (2016b). Progress and prospects for event tourism research. Tourism Management, 52, 593–631.
https://doi.org/10.1016/j.tourman.2015.03.007
Gil, A. R., & de Esteban Curiel, J. (2008). Religious events as special interest tourism. A Spanish experience. PASOS: Revista de Turismo y Patrimonio Cultural, 6, 419–433. Retrieved from https://bit.ly/387iRkm
Goodwin, H., & Santilli, R. (2009). Community-based tourism: A success? ICRT Occasional Paper 11. Retrieved from https://bit.ly/38QGDkc
Halpenny, E. A. (2008, May). Events tourism in mountain parks: Two case studies of visitor characteristics and outcomes related to special events held in Jasper and Banff National Parks, 2007. Paper presented at the Canadian Parks for Tomorrow: 40th Anniversary Conference, University of Calgary, Calgary, AB, Canada.
Hou, J.-N., & Li, X.-D. (2011). Research progress and online tourism [In Chinese]. World Regional Studies, 20, 151–158. Retrieved from https://bit.ly/2uFmPCr
Idoughi, D., Seffah, A., & Kolski, C. (2012). Adding user experience into the interactive service design loop: A persona-based approach. Behaviour & Information Technology, 31, 287–303.
https://doi.org/10.1080/0144929X.2011.563799
Karadakis, K., Kaplanidou, K., & Karlis, G. (2010). Event leveraging of mega sport events: A SWOT analysis approach. International Journal of Event and Festival Management, 1, 170–185.
https://doi.org/10.1108/17852951011077998
Laporte, L., Slegers, K., & De Grooff, D. (2012, October). Using correspondence analysis to monitor the persona segmentation process. Paper presented at the 7th Nordic Conference on Human-Computer Interaction: Making Sense Through Design, Copenhagen, Denmark.
https://doi.org/10.1145/2399016.2399058
LeRouge, C., Ma, J., Sneha, S., & Tolle, K. (2013). User profiles and personas in the design and development of consumer health technologies. International Journal of Medical Informatics, 82, e251–e268.
https://doi.org/10.1016/j.ijmedinf.2011.03.006
Liu, J., Wu, M., Yi, S., & Fan, Y. (2018). User profile of Internet parent-child travel based on IPA analysis [In Chinese]. Journal of Hunan Institute of Science and Technology (Natural Sciences), 31, 67–75.
https://doi.org/10.16740/j.cnki.cn43-1421/n.2018.01.014
McGinn, J., & Kotamraju, N. (2008, April). Data-driven persona development. Paper presented at the SIGHI Conference on Human Factors in Computing Systems, Florence, Italy.
https://doi.org/10.1145/1357054.1357292
Moscardo, G., McCarthy, B., Murphy, L., & Pearce, P. (2009). The importance of networks in special interest tourism: Case studies of music tourism in Australia. International Journal of Tourism Policy, 2, 5–23.
https://doi.org/10.1504/IJTP.2009.02327
Park, S., Tussyadiah, I. P., Mazanec, J. A., & Fesenmaier, D. R. (2010). Travel personae of American pleasure travelers: A network analysis. Journal of Travel & Tourism Marketing, 27, 797–811.
https://doi.org/10.1080/10548408.2010.527246
Pruitt, J., & Adlin, T. (2006). The persona lifecycle: Keeping people in mind throughout product design. San Francisco, CA: Morgan Kaufmann.
Rogers E. M. (1995). Diffusion of innovations (4th ed.). New York, NY: Free Press.
Sheng, C.-W., & Chen, M.-C. (2012). A study of experience expectations of museum visitors. Tourism Management, 33, 53–60.
https://doi.org/10.1016/j.tourman.2011.01.023
Sheng, C.-W., Shen, M.-J., & Chen, M.-C. (2008). An exploratory study of types of special interest tour preferences and preference demographic variables analysis. International Journal of Culture, Tourism and Hospitality Research, 2, 271–284.
https://doi.org/10.1108/17506180810891627
Swarbrooke, J., & Horner, S., (1999). Consumer behaviour in tourism. Burlington, MA: Butterworth-Heinemann.
Switzky, A. (2012, May). Incorporating UCD into the software development lifecycle: A case study. Paper presented at Extended Abstracts on Human Factors in Computing Systems, Austin, TX.
https://doi.org/10.1145/2212776.2212823
Tkaczynski, A. (2013). A stakeholder approach to attendee segmentation: A case study of an Australian Christian music festival. Event Management, 17, 283–298.
https://doi.org/10.3727/152599513X13708863377999
Trauer, B. (2006). Conceptualizing special interest tourism–Frameworks for analysis. Tourism Management, 27, 183–200.
https://doi.org/10.1016/j.tourman.2004.10.004
Tu, N., Dong, X., Rau, P.-L. P., & Zhang, T. (2010, October). Using cluster analysis in persona development. Paper presented at the 8th International Conference on Supply Chain Management and Information Systems, Hong Kong, China.
Tu, N., He, Q., Zhang, T., Zhang, H., Li, Y., Xu, H., & Xiang, Y. (2010, November). Combine qualitative and quantitative methods to create persona. Paper presented at the 3rd International Conference on Information Management, Innovation Management and Industrial Engineering, Kunming, China.
https://doi.org/10.1109/ICIII.2010.463
von Eye, A., & Schuster, C. (1998). Regression analysis for social sciences. Ann Arbor, MI: Elsevier.
Wang, X., & Meng, T. (2016). The research of customers satisfaction and public policy & marketing design in special interest tourism–Macao culinary tourism. International Journal of Business and Management, 11, 124–135.
https://doi.org/10.5539/ijbm.v11n1p124
Weed, M. (2012). Towards an interdisciplinary events research agenda across sport, tourism, leisure and health. In S. J. Page & J. Connell (Eds.), The Routledge handbook of events (pp. 57–72). Abingdon, UK: Routledge.
https://doi.org/10.4324/9780203803936
Weiler, B., & Hall, C. M. (Eds.). (1992). Special interest tourism. London, UK: Belhaven Press.
Ziakas, V. (2013). Event portfolio planning and management: A holistic approach. London, UK: Routledge.
https://doi.org/10.4324/9780203119396
Blomquist, Å., & Arvola, M. (2002, October). Personas in action: Ethnography in an interaction design team. Paper presented at the Second Nordic Conference on Human-Computer Interaction, Aarhus, Denmark.
https://doi.org/10.1145/572020.572044
Calde, S., Goodwin, K., & Reimann, R. (2002, April). SHS Orcas: The first integrated information system for long-term healthcare facility management. Paper presented at Case Studies of the CHI2002/AIGA Experience Design FORUM, Minneapolis, MN.
https://doi.org/10.1145/507752.507753
Carmichael, B. (2005). Understanding the wine tourism experience for winery visitors in the Niagara Region, ONT, Canada. Tourism Geographies, 7, 185–204.
https://doi.org/10.1080/14616680500072414
Chen, P.-J. (2010). Differences between male and female sport event tourists: A qualitative study. International Journal of Hospitality Management, 29, 277–290.
https://doi.org/10.1016/j.ijhm.2009.10.007
Cooper, A. (1999). The inmates are running the asylum: Why high tech products drive us crazy and how to restore the sanity. Indianapolis, IN: Macmillan.
Cooper, A., Reimann, R., & Cronin, D. (2007). About Face 3: The essentials of interaction design (3rd ed.). Indianapolis, IN: Wiley.
Drita, K., & Albana, G. (2011). The special interest tourism development and the small regions. Turizam, 15, 77–89.
https://doi.org/10.5937/Turizam1102077K
Faily, S., & Flechais, I. (2011, May). Persona cases: A technique for grounding personas. Paper presented at the SIGCHI Conference on Human Factors in Computing Systems, Vancouver, BC, Canada.
https://doi.org/10.1145/1978942.1979274
Getz, D. (2008). Event tourism: Definition, evolution, and research. Tourism Management, 29, 403–428.
https://doi.org/10.1016/j.tourman.2007.07.017
Getz, D., & Page, S. (2016a). Event studies: Theory, research and policy for planned events (3rd ed.). London, UK: Routledge.
Getz, D., & Page, S. (2016b). Progress and prospects for event tourism research. Tourism Management, 52, 593–631.
https://doi.org/10.1016/j.tourman.2015.03.007
Gil, A. R., & de Esteban Curiel, J. (2008). Religious events as special interest tourism. A Spanish experience. PASOS: Revista de Turismo y Patrimonio Cultural, 6, 419–433. Retrieved from https://bit.ly/387iRkm
Goodwin, H., & Santilli, R. (2009). Community-based tourism: A success? ICRT Occasional Paper 11. Retrieved from https://bit.ly/38QGDkc
Halpenny, E. A. (2008, May). Events tourism in mountain parks: Two case studies of visitor characteristics and outcomes related to special events held in Jasper and Banff National Parks, 2007. Paper presented at the Canadian Parks for Tomorrow: 40th Anniversary Conference, University of Calgary, Calgary, AB, Canada.
Hou, J.-N., & Li, X.-D. (2011). Research progress and online tourism [In Chinese]. World Regional Studies, 20, 151–158. Retrieved from https://bit.ly/2uFmPCr
Idoughi, D., Seffah, A., & Kolski, C. (2012). Adding user experience into the interactive service design loop: A persona-based approach. Behaviour & Information Technology, 31, 287–303.
https://doi.org/10.1080/0144929X.2011.563799
Karadakis, K., Kaplanidou, K., & Karlis, G. (2010). Event leveraging of mega sport events: A SWOT analysis approach. International Journal of Event and Festival Management, 1, 170–185.
https://doi.org/10.1108/17852951011077998
Laporte, L., Slegers, K., & De Grooff, D. (2012, October). Using correspondence analysis to monitor the persona segmentation process. Paper presented at the 7th Nordic Conference on Human-Computer Interaction: Making Sense Through Design, Copenhagen, Denmark.
https://doi.org/10.1145/2399016.2399058
LeRouge, C., Ma, J., Sneha, S., & Tolle, K. (2013). User profiles and personas in the design and development of consumer health technologies. International Journal of Medical Informatics, 82, e251–e268.
https://doi.org/10.1016/j.ijmedinf.2011.03.006
Liu, J., Wu, M., Yi, S., & Fan, Y. (2018). User profile of Internet parent-child travel based on IPA analysis [In Chinese]. Journal of Hunan Institute of Science and Technology (Natural Sciences), 31, 67–75.
https://doi.org/10.16740/j.cnki.cn43-1421/n.2018.01.014
McGinn, J., & Kotamraju, N. (2008, April). Data-driven persona development. Paper presented at the SIGHI Conference on Human Factors in Computing Systems, Florence, Italy.
https://doi.org/10.1145/1357054.1357292
Moscardo, G., McCarthy, B., Murphy, L., & Pearce, P. (2009). The importance of networks in special interest tourism: Case studies of music tourism in Australia. International Journal of Tourism Policy, 2, 5–23.
https://doi.org/10.1504/IJTP.2009.02327
Park, S., Tussyadiah, I. P., Mazanec, J. A., & Fesenmaier, D. R. (2010). Travel personae of American pleasure travelers: A network analysis. Journal of Travel & Tourism Marketing, 27, 797–811.
https://doi.org/10.1080/10548408.2010.527246
Pruitt, J., & Adlin, T. (2006). The persona lifecycle: Keeping people in mind throughout product design. San Francisco, CA: Morgan Kaufmann.
Rogers E. M. (1995). Diffusion of innovations (4th ed.). New York, NY: Free Press.
Sheng, C.-W., & Chen, M.-C. (2012). A study of experience expectations of museum visitors. Tourism Management, 33, 53–60.
https://doi.org/10.1016/j.tourman.2011.01.023
Sheng, C.-W., Shen, M.-J., & Chen, M.-C. (2008). An exploratory study of types of special interest tour preferences and preference demographic variables analysis. International Journal of Culture, Tourism and Hospitality Research, 2, 271–284.
https://doi.org/10.1108/17506180810891627
Swarbrooke, J., & Horner, S., (1999). Consumer behaviour in tourism. Burlington, MA: Butterworth-Heinemann.
Switzky, A. (2012, May). Incorporating UCD into the software development lifecycle: A case study. Paper presented at Extended Abstracts on Human Factors in Computing Systems, Austin, TX.
https://doi.org/10.1145/2212776.2212823
Tkaczynski, A. (2013). A stakeholder approach to attendee segmentation: A case study of an Australian Christian music festival. Event Management, 17, 283–298.
https://doi.org/10.3727/152599513X13708863377999
Trauer, B. (2006). Conceptualizing special interest tourism–Frameworks for analysis. Tourism Management, 27, 183–200.
https://doi.org/10.1016/j.tourman.2004.10.004
Tu, N., Dong, X., Rau, P.-L. P., & Zhang, T. (2010, October). Using cluster analysis in persona development. Paper presented at the 8th International Conference on Supply Chain Management and Information Systems, Hong Kong, China.
Tu, N., He, Q., Zhang, T., Zhang, H., Li, Y., Xu, H., & Xiang, Y. (2010, November). Combine qualitative and quantitative methods to create persona. Paper presented at the 3rd International Conference on Information Management, Innovation Management and Industrial Engineering, Kunming, China.
https://doi.org/10.1109/ICIII.2010.463
von Eye, A., & Schuster, C. (1998). Regression analysis for social sciences. Ann Arbor, MI: Elsevier.
Wang, X., & Meng, T. (2016). The research of customers satisfaction and public policy & marketing design in special interest tourism–Macao culinary tourism. International Journal of Business and Management, 11, 124–135.
https://doi.org/10.5539/ijbm.v11n1p124
Weed, M. (2012). Towards an interdisciplinary events research agenda across sport, tourism, leisure and health. In S. J. Page & J. Connell (Eds.), The Routledge handbook of events (pp. 57–72). Abingdon, UK: Routledge.
https://doi.org/10.4324/9780203803936
Weiler, B., & Hall, C. M. (Eds.). (1992). Special interest tourism. London, UK: Belhaven Press.
Ziakas, V. (2013). Event portfolio planning and management: A holistic approach. London, UK: Routledge.
https://doi.org/10.4324/9780203119396
Table 1. Orthogonal Coding of the Independent Variables

Table 2. Characteristics of Tourists in Event and Special Interest Tourism
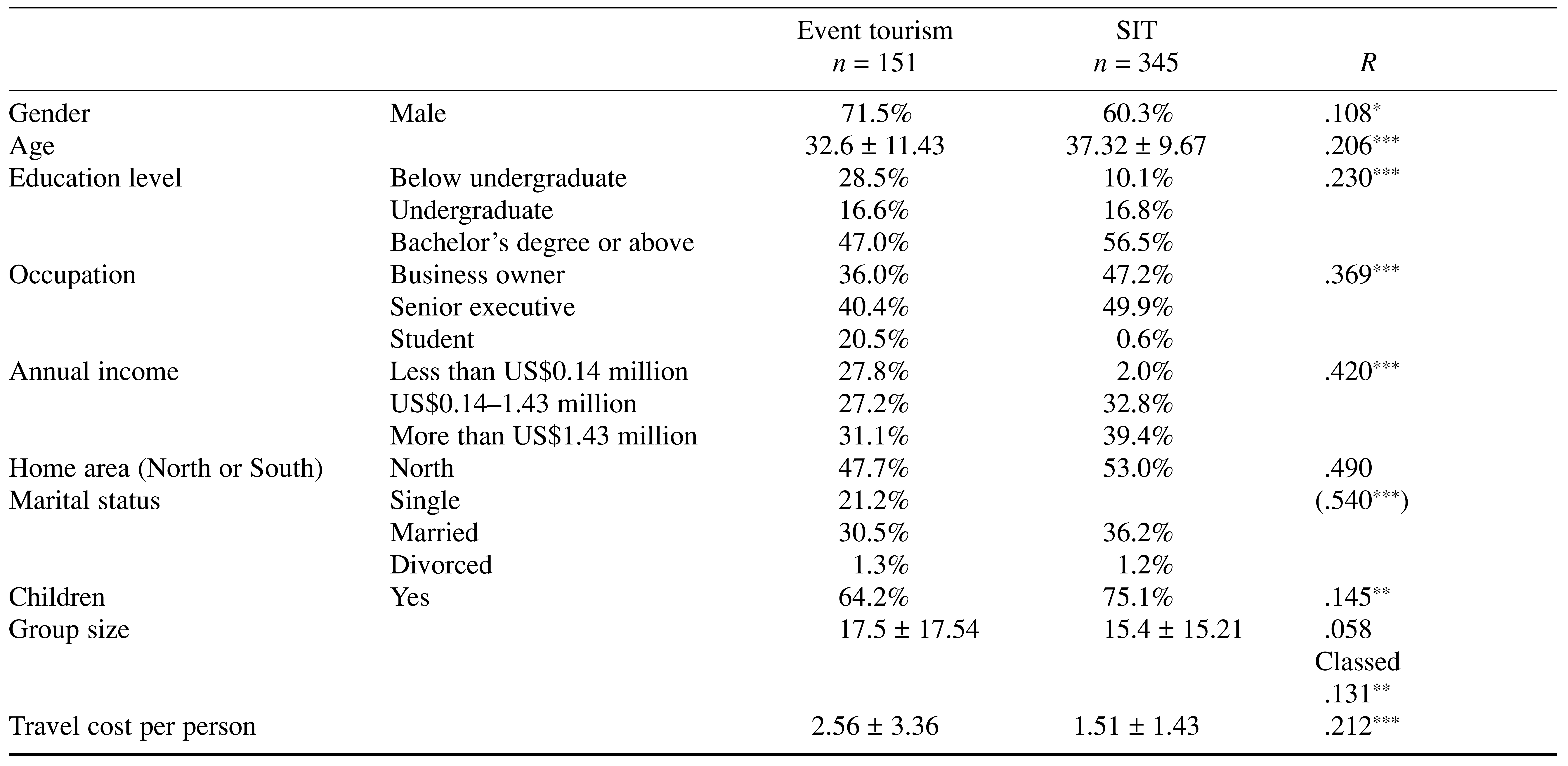
Note. SIT = special interest tourism. Age was measured in years and the unit of the average travel cost per person was US$10,000.
* p < .05, ** p < .01, *** p < .001.
Table 3. Correlation Matrix of the Independent Variables
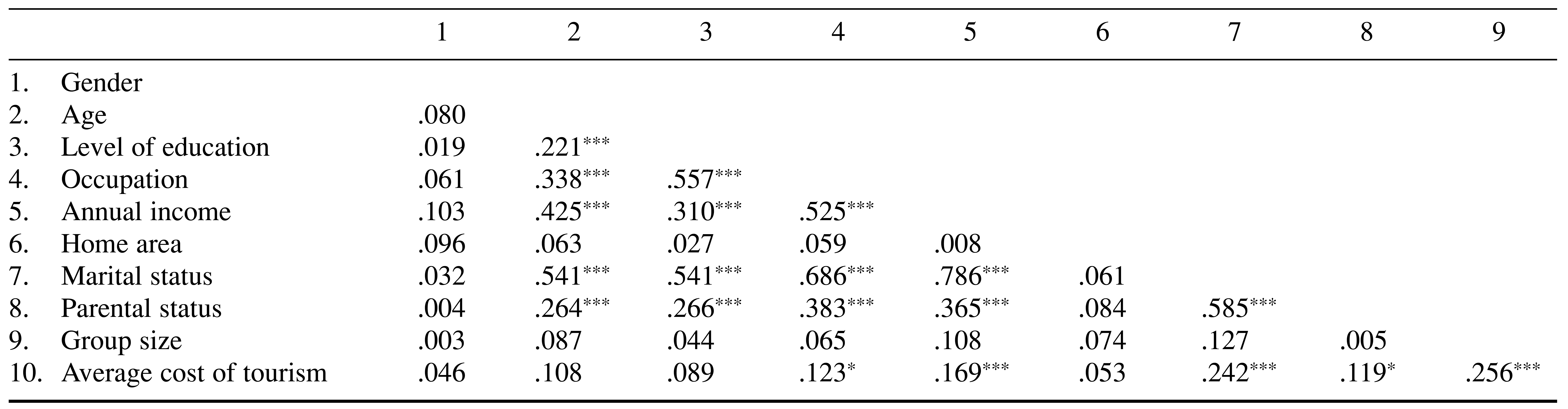
Note. * p < .05, *** p < .001.
Table 4. Results of Model 1
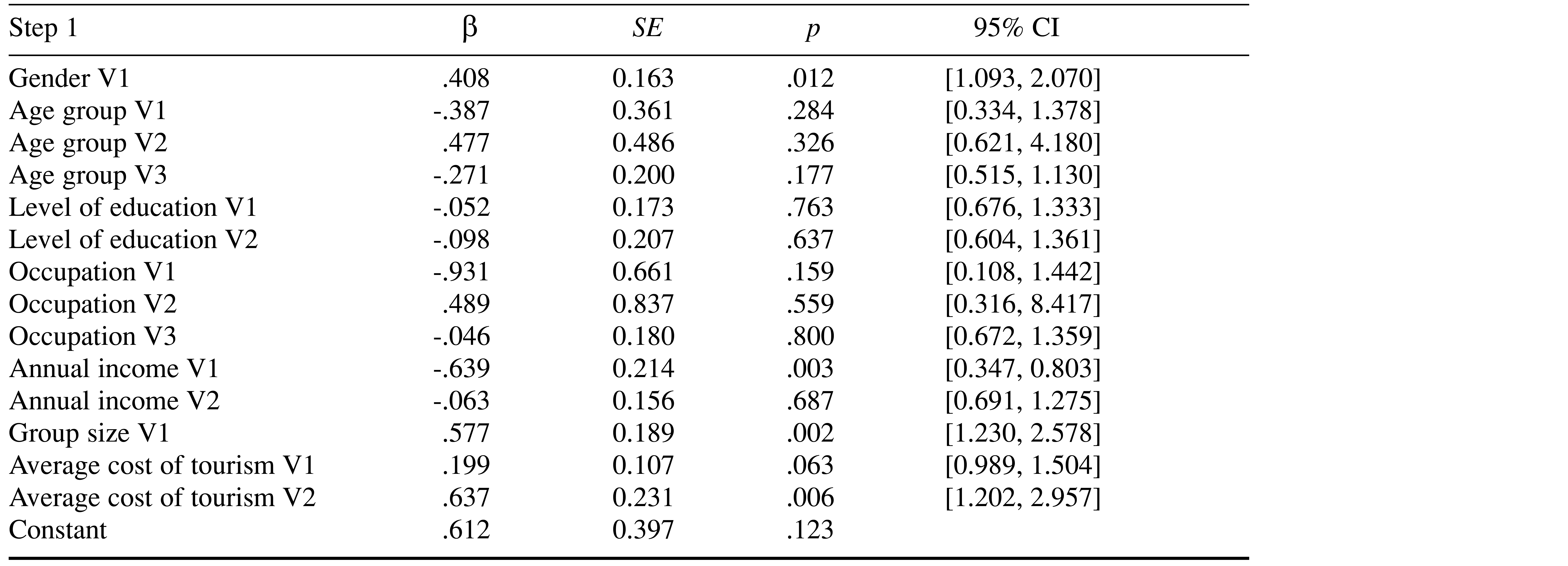
Note. V1 = Vector 1, V2 = Vector 2, V3 = Vector 3, CI = confidence interval.
Table 5. Results of Model 2
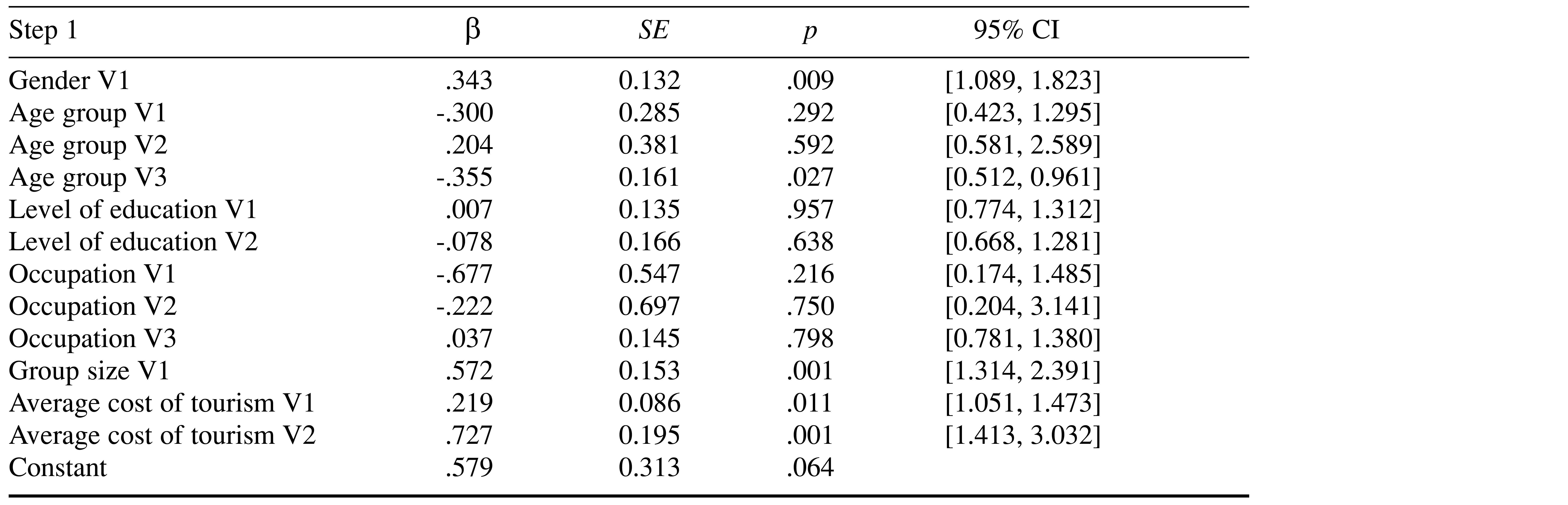
Note. V1 = Vector 1, V2 = Vector 2, V3 = Vector 3, CI = confidence interval.
Table 6. Results of Model 3
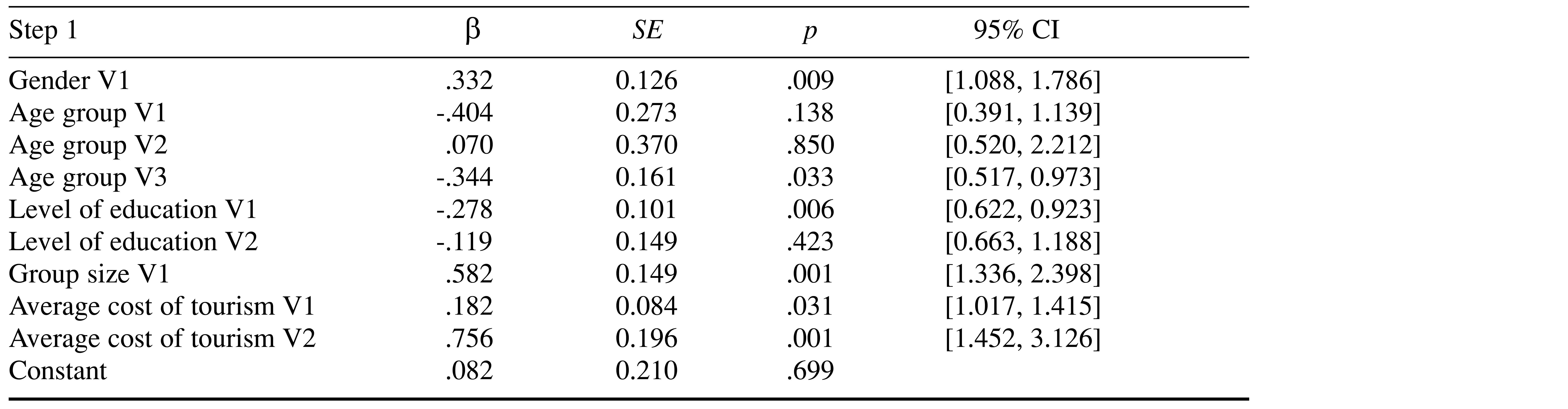
Note. V1 = Vector 1, V2 = Vector 2, V3 = Vector 3, CI = confidence interval.
Table 7. Results of Model 4

Note. V1 = Vector 1, V2 = Vector 2, CI = confidence interval.
This study was supported by Niding Traveling
whose staff members provided real data about key opinion leaders and traveling information.
The author also extends gratitude to Professor Pei-Luen Patrick Rau for his support and guidance.
Rui Kang, Department of Industrial Engineering, Tsinghua University, No. 1, Zhongguancun East Road, Haidian, Beijing 100084, People’s Republic of China. Email: [email protected]