Crowding perception predicts subway passengers’ behavioral decision making during COVID-19 via risk awareness and safety perception
Main Article Content
We studied the prediction mechanism of the effects of the crowding perception, risk awareness, and safety perception of subway passengers on their behavioral decision making in the context of COVID-19 as a public health safety crisis. We conducted a survey of 305 subway passengers in Zhejiang Province, China, and used structural equation modeling for data analysis. The results show that the crowding perception of the passengers did not directly predict their behavioral decision making. Rather, risk awareness and safety perception played a chain mediating role between the crowding perception and behavioral decision making of passengers. We further explored the theoretical and practical implications of our findings.
The coronavirus disease 2019 (COVID-19) pandemic is a major global public health security crisis. Li et al. (2021) showed that maintaining social distancing is an important measure to reduce the risk of transmission of the virus. Underground railways (subways) are an important public transportation system where crowding is common. Thus, it is necessary to prevent subway passengers from becoming tired of maintaining social distance (MacIntyre et al., 2021), and to ensure that they are making proactive behavioral decisions related to avoiding crowding, including choosing to board the carriage that is least crowded, avoiding crowded platforms, keeping as much distance as possible from other passengers, and, with the approval of their employer, working on staggered shifts.
The safety behavior of public transport passengers has received attention from scholars. For example, X. Wang et al. (2020) used the theory of planned behavior (Ajzen, 1991) to explain the formation mechanism of safety behavior of public transport passengers, which helps to safeguard transport security and reduce risks (Stanojević et al., 2020). X. Wang et al. analyzed the role of elements such as passengers’ behavioral attitude, safety awareness, and safety perception. As a planned and predictive thinking process, behavioral decision making directly controls individuals’ actions or actual behaviors (Imani et al., 2021), so it is necessary to study the prediction mechanism of subway passengers’ behavioral decision making. However, in the context of the COVID-19 pandemic, few empirical studies have been conducted in which elements have been considered, such as subway passenger’ perception of crowded environments, cognition of the environmental security, and psychological safety perception, to analyze their decisions regarding safety behaviors, such as avoiding crowding. To bridge the gap in the literature, we integrated theoretical insights from different psychological theories to explore the predictive factors of passengers’ behavioral decision making around avoiding crowding on the subway in the context of the COVID-19 pandemic.
Most studies have considered individual external factors involving the state of the objective environment, and internal factors involving the individual’s awareness and perception. For example, Witting et al. (2021) suggested that the main factors predicting ski tourists’ safety behavior decisions to carry avalanche safety equipment can be grouped into two categories: weather factors external to the individual (bad weather motivating them to carry avalanche safety equipment), and individual skiers’ internal safety awareness and behavioral habits. In a study of accident causation, Fu et al. (2019) showed that the safety behavior of individuals is predicted by the chain transmission of external safety culture, safety management systems, and individual internal factors that include safety knowledge, awareness, and psychological state. Furthermore, Y. Huang et al. (2021) noted that in the context of the COVID-19 pandemic and according to the process of chain transmission, adding advertisements with self-directed content (external environmental feature) can arouse fear in people with Machiavellian personality characteristics and thus guide their behavior. Therefore, we speculated that individual safety behavior decisions may involve external environmental influences and internal psychological factors.
Several related theories have been used to investigate the mechanisms of predicting individual behavior. Bandura (1978) stated that in reciprocal determinism, human behavior is related to the interaction between environmental factors and personal factors (i.e., cognition and emotion). During the COVID-19 pandemic, the generation of subway passengers’ safety behavioral decisions may be predicted by both environmental and personal factors; the former may involve crowding inside the subway, and the latter may involve subway passengers’ risk awareness and safety perception. In the stimulus–response theory of the school of behaviorism in social psychology, it is asserted that most human behavior results from a connection formed by certain behavioral responses after the body encounters a particular stimulus (Skinner, 1935). Therefore, we speculated that the behavioral decision of subway passengers to avoid crowding may be a result of their reaction to the stimulus of the congested external environment. In the cognitive consistency theory of the school of cognitivism, it is similarly maintained that when individuals receive new information that contradicts their beliefs, opinions, or attitudes, they will spontaneously adjust their original views to make the new information consistent with the old (Milne, 1976). When perceiving congestion, during the COVID-19 pandemic subway passengers may adjust their behavioral decisions to avoid crowding as much as possible if they realize that the situation is not conducive to preventing spread of the pandemic. If people have a high level of crisis awareness and remain vigilant to external risks, they are more likely to perform risk-averse behavioral responses and protect themselves from threats and harm (W. Huang et al., 2019; Surry, 1969). This situation may occur in the response of subway passengers to crowding in the context of the COVID-19 pandemic. If they feel that the crowded subway environment is unsafe, they may make corresponding decisions to avoid crowds and reduce the potential risk of being infected.
On the basis of the above analysis of related studies and theories, we argued that there may be correlations among environmental stimuli, and individuals’ awareness, perception, and behavior. Thus, we speculated that passengers’ perception of external environmental stimuli, as well as their internal personal awareness and perception, can be considered when studying the prediction of safety behavioral decisions of subway passengers. As a common phenomenon in subway transportation, congestion may weaken passengers’ willingness to choose the subway as a travel mode and may motivate passengers to make behavioral decisions to avoid congestion (Chowdhury & Ceder, 2016). Subway passengers can make corresponding behavioral decisions to avoid crowding, so we took subway passengers’ crowding perception into consideration. Crowding perception is defined as the degree of restriction of body movement felt by passengers during subway rides (Kim et al., 2016). Differing from describing the density of objective and available space, it is a mental state generated by people based on their external spatial environment, which is also a subjective experience perception formed by the integration of factors, such as the degree of space restriction and social interaction (Stokols, 1972). Kim et al. (2016) regarded spatial crowding as the degree to which being in a crowd restricts individuals’ physical movement, reflecting the individuals’ perceived density of close crowds. In our research, crowding perception reflects subway passengers’ feelings about the density of crowds close to them.
According to the cognitivist theory discussed previously, individual awareness and perception could affect behavioral decisions (Bandura, 1978). Specifically, in a study involving public risks, Vicente et al. (2014) showed that the public’s risk awareness and perception of security risks would impact on their risk decisions and behavior. Therefore, we took passengers’ risk awareness and safety perception into consideration. Risk awareness can be interpreted as an individual’s theoretical analysis of the relevant risk environment, referring to their judgment of external risks (Pagneux et al., 2011) and threats; it is generated by their own attitudes, skills, knowledge, and other elements. Safety perception refers to passengers’ sense of certainty and ability to control the subway environment, reflecting individuals’ sense of external risk (Chowdhury & Ceder, 2016). Different from risk awareness, which is based on certain theoretical knowledge of risk and the rational thinking process (Pagneux et al., 2011), safety perception reflects individuals’ direct feeling of the controllability of the external risk environment, which may be more perceptual than is risk awareness (Chowdhury & Ceder, 2016). In addition, in our study behavioral decision making refers to the behavioral choices of subway passengers regarding congestion conditions and, thus, their consideration of whether to take measures to avoid crowding.
Theoretical Basis and Hypotheses
Crowding Perception Prediction on Behavioral Decision Making
In the context of crises, predicting humans’ behavioral decision making requires considering their perception of the external environment (Liu et al., 2019). When faced with a crowded external environment, people who feel restricted in their movement will be motivated to make behavioral decisions to avoid the crowds. For example, in the context of shopping, people may choose to avoid stores with high crowd densities or restricted activity spaces, and turn to other stores (Mehta, 2013; Stokols, 1976). In a study on commuter travel Verhoeff (2014) noted that commuters may choose a longer route to avoid crowding. Furthermore, in task-based actions rather than recreational actions, perceived crowding may negatively affect individuals’ emotions, causing them to reject the crowded environment, which drives them to respond to this perception with their behavioral decisions (Hui & Bateson, 1991). Therefore, we speculated that for most passengers, taking the subway is a task of commuting to reach a destination, such as the workplace, rather than a hedonic activity. Thus, the crowded environment of the subway may prompt commuters to make decisions to avoid crowding. Previous researchers (Hui & Bateson, 1991; Mehta, 2013; Stokols, 1976; Verhoeff, 2014) have shown that individuals’ decisions to avoid crowding are related to their crowding perception. Hence, we proposed the following hypothesis:
Hypothesis 1: Subway passengers’ crowding perception will prompt them to make behavioral decisions to avoid crowding while riding the subway.
Mediating Role of Risk Awareness
Risk awareness may affect individuals’ behavioral decisions when faced with risky situations (Stoop, 1997). When identifying new situations or objects, individuals will form cognitions based on information from their previous experiences and newly acquired knowledge, which affects their behavioral decision making (Tversky & Kahneman, 1974). When studying the behavioral decision making of subway passengers in the context of a public health safety crisis, it is necessary to understand their risk awareness.
According to cognitive map theory, in a specific environment an individual’s cognition of the external environment comes from the interactions among motivation by environmental factors, personal experiences, media coverage, scientific reports, and individuals’ cognition of the general properties of the environment that can be obtained perceptually. That is, under the condition of a specific environmental perception individuals mobilize their cognition of the environment’s general properties to determine their action (Tolman, 1948). When subway passengers perceive crowding, they may consciously judge the risk level of this crowded environment based on their own experience and knowledge and, further, may make corresponding behavioral decisions driven by this risk awareness. Bargh et al. (1992) suggested that stimuli from the external environment can inspire individuals’ evaluations of that environment. Therefore, it can be speculated that in the context of the COVID-19 pandemic, when subway passengers are stimulated by a crowded environment, they may be driven to judge the risk of such an environment, further leading them to realize that there are risks of infection in this environment. Cambon de Lavalette et al. (2009) found that pedestrians’ subjective cognitions under specific situational constraints could affect their behavioral decision making. When they recognized a behavioral code that needed to be followed, they would make behavioral decisions that were appropriate for that code. In the context of the COVID-19 pandemic, when subway passengers are aware of the risks of crowded environments and believe that they should avoid those environments, they may make various behavioral decisions to do so.
These previous studies have explored how individuals’ behavioral decisions are based on certain environmental perceptions that are driven by their awareness. Nevertheless, there are few studies in which this prediction mechanism has been placed in the context of the COVID-19 pandemic to explore the behavioral decisions of subway passengers based on their crowding perceptions.
We believed that when subway passengers perceived a crowded environment, they might incorporate their own past experiences and cognition to generate instant judgment of the safety of the travel environment, which would further prompt them to make behavioral decisions consistent with this cognition. For example, when passengers feel crowded, this perception of crowding may lead them to be aware of this risky environment. Driven by this risk awareness, they may make various behavioral decisions related to avoid both crowding and the risk. Therefore, we proposed the following hypothesis:
Hypothesis 2: Subway passengers’ risk awareness will play a mediating role between their crowding perception and behavioral decision making.
Mediating Role of Safety Perception
In the context of the COVID-19 pandemic, the public’s perception of social distancing may become more sensitive, which may predict their behavioral decisions. For example, in a study by D. Wang et al. (2021) conducted with 593 North American consumers and 591 Australian consumers during the COVID-19 pandemic, the results showed that North American consumers were more sensitive than Australians were to social distancing in restaurants, so that restaurants with dense crowding may have made them feel unsafe, thus weakening their intention to eat in very crowded restaurants. Furthermore, Yin et al. (2020) suggested that under the condition of perceived risk, crowding perception can make a destination less attractive to tourists. We therefore speculated that in the COVID-19 pandemic, crowding perception could weaken subway passengers’ safety perception and, in turn, cause them to make behavioral decisions to avoid crowding.
The safety perception of passengers is an important entry point for predicting their behavioral decision-making tendencies (Legrain et al., 2015). When subway passengers sense that the crowds around their bodies are too dense, they may feel unsafe and become alert to the need to take evasive action to avoid the crowding (Tirachini et al., 2017). Additionally, when passengers perceive the external environment is crowded, this will not only make them feel unsafe, but also will predict their behavioral decision making related to avoiding crowds, with their anxiety as an impetus (Márquez et al., 2019). Moreover, Cox et al. (2006) showed that when railway passengers felt that the travel environment was too crowded, their safety perception was reduced. This emotional change would make them inclined to take practical actions to avoid risks. Therefore, we speculated that in the context of the COVID-19 pandemic, subway passengers would feel less physically safe based on the crowded environment they perceive and, thus, would make behavioral decisions to avoid crowding. The following hypothesis was formed:
Hypothesis 3: Subway passengers’ safety perception will play a mediating role between their crowding perception and behavioral decision making.
Chain Mediation Effects Between Crowding Perception and Behavioral Decision Making
In the ABC emotion theory framework Ellis (1991) demonstrated the interrelationship of the activating event, belief, and behavioral outcome. This theory suggests that the activating event causes individuals to develop attitudes and emotions about the event, resulting in a belief that predicts the individuals’ behavior. Therefore, we speculated that in the context of the COVID-19 pandemic, subway passengers’ perception of crowding as a precipitating event may ultimately predict their behavioral decisions by causing them to form a perception that the situation is risky, resulting in related emotions.
Individuals’ awareness of risk and safety perception may impact on their behavioral decisions in specific risk environments. In a study involving air travel security that was based on emotion theory, Morosan (2017) noted that concerns about privacy breach risks and individual safety perceptions significantly predicted air travelers’ intention to disclose personal information. Morosan also found that both positive and negative emotional attitudes to privacy disclosure risk affected their security perception. Therefore, we speculated that in a crowded environment, subway passengers’ risk awareness in regard to crowding during the COVID-19 pandemic may affect their safety perception, and further affect their behavioral decisions on crowding.
Moreover, Shi et al. (2003) demonstrated that during the severe acute respiratory syndrome (SARS) epidemic period, individuals would have stronger risk awareness after receiving negative information about SARS, thus affecting their safety perception. This indicates that in the context of a public health security crisis, individuals’ safety perception may be related to their awareness of external risks.
To sum up, we anticipated that subway passengers would be aware of the risk of this situation under the impetus of crowding perception, and this kind of risk awareness would further affect their safety perception, motivating their behavioral decisions. Thus, we proposed the following hypothesis:
Hypothesis 4: Subway passengers’ risk awareness and safety perception will play a chain mediating role between their crowding perception and behavioral decision making.
We constructed a hypothetical model predicting the effect of subway passengers’ crowding perception on their behavioral decision making (see Figure 1).
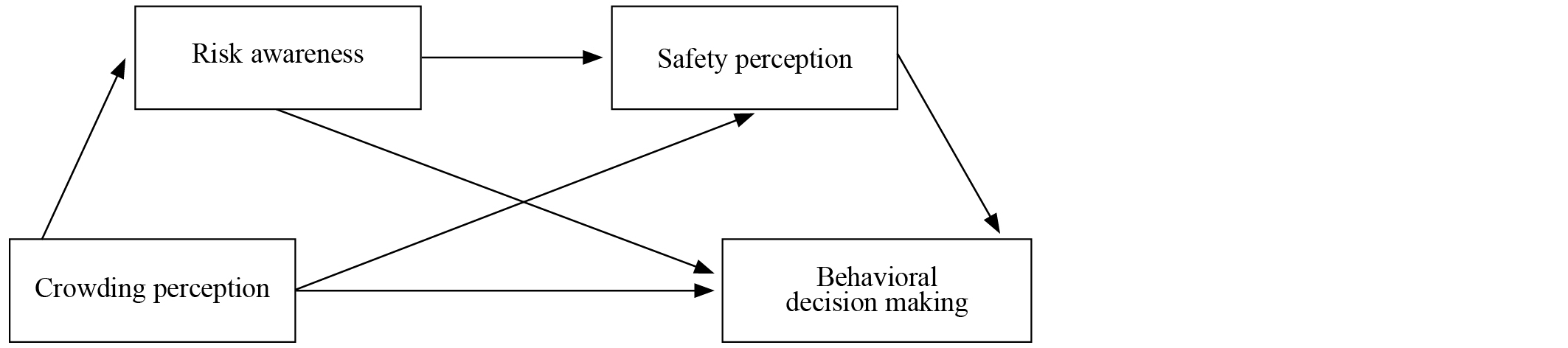
Figure 1. Theoretical Model
Method
Participants and Procedure
Ethical approval for the study was obtained from the Academic Committee of Shanghai Institute of Technology. We collected data for the study in an online survey distributed through WJX (https://www.wjx.cn/). Participants were from Hangzhou and Ningbo, the cities that have subways in Zhejiang Province, China. The survey was conducted from February 28 to March 15, 2020. At the beginning of the survey, all participants were informed of the purpose of the research. We also preset a condition: “Have you ever taken the subway in Hangzhou or Ningbo during the COVID-19 pandemic?” The survey did not include participants’ personal confidential information (e.g., name, home address, workplace).
After a 16-day distribution cycle, we had received 537 responses, of which 305 were valid (i.e., the respondents had taken the subway in Hangzhou or Ningbo during the COVID-19 pandemic). Among the 305 valid responses, 146 (47.87%) were from women and 159 (52.13%) were from men. With regard to age, 1 (0.33%) participant was under 18 years old, 70 (22.95%) were aged 18–25 years, 100 (32.79%) were aged 26–30 years, 92 (30.16%) were aged 31–40 years, 29 (9.51%) were aged 41–50 years, 11 (3.61%) were aged 51–60 years, and 2 (0.66%) were aged over 60 years. With regard to educational level, 58 (19.02%) participants had graduated from high school or had a lower educational qualification, 106 (34.75%) had a college degree, 117 (38.36%) had a bachelor’s degree, and 24 (7.87%) had a master’s degree or postgraduate qualification.
Measures
The measurements were adapted from existing scales. All items except demographic variables were rated on a 5-point Likert scale ranging from 1 (strongly disagree) to 5 (strongly agree). The two authors, who both have many years of experience studying and using English, were responsible for all translations of the three measures used in the study that were developed in English, which were a crowding perception scale developed by Kim et al. (2016), a safety perception scale developed by Sam et al. (2019), and a behavioral decision-making scale developed by Rice and Winter (2019).
Mousheng Song translated the original items into Chinese, and further revised these according to the specific research background. Then, after discussion with Yang Cao, these items were further refined to make them colloquial and easier for the respondents to understand, thus forming the preliminary version of the Chinese measures. Both authors then proofread all the items to check whether there was any misunderstanding of the original meaning and unclear expression, and then compiled them into a unified survey for distribution. Finally, both authors translated the Chinese items back into English.
Crowding Perception
Combined with a scene of subway passengers taking the subway, we measured subway passengers’ crowding perception with the following items from Kim et al. (2016): “I felt suffocated in the subway,” “I felt cramped in the subway,” and “Moving around in the subway was inconvenient.” A higher score indicates a higher level of crowding perception. Cronbach’s alpha coefficient in this study was .85 and the average variance extraction (AVE) was .85.
Risk Awareness
We used items from Chen et al. (2017) to measure subway passengers’ risk awareness. For our study, we revised the original items in the Chinese language to fit the specific research background and research objects: “During the COVID-19 pandemic, I think there is a risk of public health security when riding on a crowded subway”; “During the COVID-19 pandemic, I think avoiding congestion can reduce the risk of public health security”; and “I think the subway has the risk of spreading the pandemic.” A higher score indicates greater awareness of risk in subway travel. Cronbach’s alpha coefficient in this study was .92, and the AVE was .79.
Safety Perception
We used items from Sam et al. (2019) to measure subway passengers’ safety perception: “The passenger capacity of the subway made me feel at ease,” “The antipandemic environment in the subway made me felt reassured,” “The distance between the others and me in the subway made me feel reassured,” and “I was reassured by the amount of congestion in my carriage.” Higher scores indicate a stronger sense of safety. Cronbach’s alpha coefficient in this study was .95 and the AVE was .83.
Behavioral Decision Making
We used items from Rice and Winter (2019) to measure subway passengers’ behavioral decision making related to avoiding congestion: “I don’t mind taking the crowded subway, that is to say, when I encounter a crowded subway train, I won’t wait for another”; “When I find that there are too many people in the subway station, I wouldn’t give up the subway travel mode”; “After experiencing a crowded subway trip, I will still choose the subway to travel in the future”; and “In the subway station, I won’t choose to avoid the crowded areas.” Lower scores indicate a greater likelihood of making behavioral decisions to avoid congestion. Cronbach’s alpha coefficient in this study was .95 and the AVE was .82.
Results
Measurement Model
We performed a confirmatory factor analysis before using structural equation modeling to assess whether the theoretical model was reasonable. The results show that the model had a good fit to the data, chi square/degrees of freedom = 2.04, p < .01, normed fit index = .97, goodness-of-fit index = .94, adjusted goodness-of-fit index = .90, comparative fit index = .99, root mean square error of approximation = .06. All factor loadings exceeded .70. These results indicate the adequate discriminant and convergent validity of the model.
Descriptive Statistics and Correlation Analysis
The correlation coefficients between the variables and the results of descriptive statistical analysis are shown in Table 1. Crowding perception was significantly and positively correlated with safety perception. Further, crowding perception and risk awareness were significantly and negatively correlated with safety perception and behavioral decision making, respectively, whereas safety perception was significantly and positively correlated with behavioral decision making.
Table 1. Descriptive Statistical Results and Correlations for the Variables

Note. ** p < .01.
Hypothesis Testing
We assessed the mediation effects of the model using PROCESS Model 6 in SPSS 23.0 (Hayes, 2013), with 5,000 bootstrapped resamples and 95% confidence intervals (CIs). We found that the direct effect of crowding perception → behavioral decision making was not significant, b = −0.06, p = .09, t(305) = 1.73, 95% CI [−0.01, 0.13], so it did not support our prediction (Hypothesis 1) that subway passengers' crowding perception would prompt them to make behavioral decisions to avoid crowding while riding the subway. Table 2 provides the results of the indirect effects analysis. As shown in Table 2, the 95% CI of the indirect effect of crowding perception → risk awareness → behavioral decision making relationship did not contain zero, indicating that the mediating effect was significant, thus supporting our prediction that subway passengers’ risk awareness would mediate the relationship between crowding perception and behavioral decision making. Therefore, Hypothesis 2 was supported. The 95% CI of the indirect effect of crowding perception → safety perception → behavioral decision making did not contain zero, indicating that the mediating effect was significant, thus supporting the prediction that safety perception would mediate the relationship between subway passengers’ crowding perception and behavioral decision making. Therefore, Hypothesis 3 was supported. The 95% CI of the indirect effect of crowding perception → risk awareness → safety perception → behavioral decision making did not contain zero, indicating that the mediating effect was significant, thus supporting the prediction that safety perception and risk awareness would mediate the relationship between subway passengers’ crowding perception and behavioral decision making. Therefore, Hypothesis 4 was supported. The 95% CI of the total indirect effect did not contain zero, so the total indirect effect was verified.
Table 2. Mediating Effects Test

Note. Boot = bootstrapped; CI = confidence interval; LL = lower limit; UL = upper limit.
The results of the mediating effects test suggest that according to their perceived high level of crowding, subway passengers’ behavioral decisions to avoid congestion can be motivated by stronger risk awareness and weaker safety perception.
Discussion
Theoretical Implications
We paid attention to the relationship of subway passengers’ crowding perception, risk awareness, safety perception, and behavioral decision making in the context of the COVID-19 pandemic. We further explored the prediction mechanisms for subway passengers’ safety behavioral decision making related to avoiding crowding and provided empirical evidence for these mechanisms to add to the literature on the prediction mechanism of subway passengers’ safety behavioral decision making during the COVID-19 pandemic.
Our findings indicate that subway passengers’ crowding perceptions can predict their behavioral decision making related to avoiding crowding through their risk awareness and safety perception. Differing from the findings of Mehta (2013), we found that subway passengers’ crowding perception did not directly predict their behavioral decision making. The mediating role of both risk awareness and safety perception helped explain the mechanism of crowding perception on passengers’ behavioral decision making, which verifies the role of these factors in the prediction mechanism of behavioral decision making (Cambon de Lavalette et al., 2009; Márquez et al., 2019).
Practical Implications
In the context of safety management, managers are eager to know how to persuade the public to make behavioral decisions conducive to maintaining a safe atmosphere. Recently, researchers have been exploring which factors affect individuals’ safety behavioral decisions or are the formation mechanisms of individuals’ safety behavioral decisions (Xia et al., 2020; Zhang et al., 2021). However, in the context of the COVID-19 pandemic, methods to prompt subway passengers to make behavioral decisions to avoid crowding, which is conducive to maintaining public health safety, have received less scholarly attention.
Our findings may provide practical implications for safe transportation in subways during the COVID-19 pandemic and in public policy formulation in response to the public health security crisis. We demonstrated that subway passengers’ crowding perception can predict their behavioral decision making through the mediating roles of risk awareness and safety perception. Enhancing passengers’ risk awareness and vigilance may be achieved by strengthening safety education, which can improve passengers’ safety knowledge level, correct their attitude and increase their safety awareness and, thus, their vigilance in regard to keeping themselves safe (Chang & Liao, 2009). To achieve the effect of alleviating subway crowding and maintaining public health security of the public when they are traveling on the subway, various forms of publicity, education, and psychological intervention are needed to increase passengers’ vigilance with regard to avoiding crowding, for example, improving the content and forms of publicity on maintaining social distance in subway stations and trains; using social media and other methods to reinforce the education of subway passengers to maintain social distances; traveling at nonpeak hours, and staggering the times for staff of businesses to start and finish work so that not all members are on the subway at the same time, making it possible for passengers to realize that avoiding crowding is essential for maintaining their own safety, and prompting them to make active decisions to avoid crowding.
Limitations and Future Research Directions
There are several limitations to our study. First, we did not consider the influence of cultural differences on the behavioral decision making of subway passengers. Regarding the impact of cultural differences on antipandemic measures, MacIntyre et al. (2021) found that people from different countries have different responses to the implementation of antipandemic measures. Therefore, the role of cultural differences could be considered in future related research. Second, our study had a correlational rather than experimental design, so causality cannot be inferred. To conduct a comprehensive investigation of the influence mechanism of subway passengers’ behavior decisions, further experimental studies are needed in the future, under highly controlled experimental conditions (e.g., prime one group with perception of crowdedness vs. another group with perception of empty space, or neutral group). Moreover, our study had a purely cross-sectional design, so we cannot be confident of the best sequence of the variables measured. Therefore, researchers could conduct longitudinal studies to provide more conclusive results. It may also be significant for researchers to conduct multiple cross-sectional studies at different time nodes.
References
Ajzen, I. (1991). The theory of planned behavior. Organizational Behavior and Human Decision Processes, 50(2), 179–211.
https://doi.org/10.1016/0749-5978(91)90020-T
Bandura, A. (1978). The self system in reciprocal determinism. American Psychologist, 33(4), 344–358.
https://doi.org/10.1037/0003-066X.33.4.344
Bargh, J. A., Chaiken, S., Govender, R., & Pratto, F. (1992). The generality of the automatic attitude activation effect. Journal of Personality and Social Psychology, 62(6), 893–912.
https://doi.org/10.1037/0022-3514.62.6.893
Cambon de Lavalette, B., Tijus, C., Poitrenaud, S., Leproux, C., Bergeron, J., & Thouez, J.-P. (2009). Pedestrian crossing decision-making: A situational and behavioral approach. Safety Science, 47(9), 1248–1253.
https://doi.org/10.1016/j.ssci.2009.03.016
Chang, Y.-H., & Liao, M.-Y. (2009). The effect of aviation safety education on passenger cabin safety awareness. Safety Science, 47(10), 1337–1345.
https://doi.org/10.1016/j.ssci.2009.02.001
Chen, Y., Wang, Y., Nie, Q., Zheng, J., & Ding, C. (2017). Modeling and an analysis of safety perception of online car-hailing passengers based on a structural equation model. Journal of Transport Information and Safety, 35(6), 85–92.
Chowdhury, S., & Ceder, A. (2016). Users’ willingness to ride an integrated public-transport service: A literature review. Transport Policy, 48, 183–195.
https://doi.org/10.1016/j.tranpol.2016.03.007
Cox, T., Houdmont, J., & Griffiths, A. (2006). Rail passenger crowding, stress, health and safety in Britain. Transportation Research Part A: Policy and Practice, 40(3), 244–258.
https://doi.org/10.1016/j.tra.2005.07.001
Ellis, A. (1991). The revised ABC’s of rational-emotive therapy (RET). Journal of Rational-Emotive and Cognitive-Behavior Therapy, 9, 139–172.
https://doi.org/10.1007/BF01061227
Fu, G., Zhao, Z., Hao, C., & Wu, Q. (2019). The accident path of coal mine gas explosion based on 24 model: A case study of the Ruizhiyuan gas explosion accident. Processes, 7(2), Article 73.
https://doi.org/10.3390/pr7020073
Hayes, A. F. (2013). Introduction to mediation, moderation, and conditional process analysis: A regression-based approach. Guilford Press.
Huang, W., Shuai, B., Zuo, B., Xu, Y., & Antwi, E. (2019). A systematic railway dangerous goods transportation system risk analysis approach: The 24 model. Journal of Loss Prevention in the Process Industries, 61, 94–103.
https://doi.org/10.1016/j.jlp.2019.05.021
Huang, Y., Yang, S., & Dai, J. M. (2021). Self- versus other-directed outcomes, Machiavellianism, and hypothetical distance in COVID-19 antipandemic messages. Social Behavior and Personality: An international journal, 49(3), Article e10109.
https://doi.org/10.2224/sbp.10109
Hui, M. K., & Bateson, J. E. G. (1991). Perceived control and the effects of crowding and consumer choice on the service experience. Journal of Consumer Research, 18(2), 174–184.
https://doi.org/10.1086/209250
Imani, E., Harati, A., Pourreza, H., & Goudarzi, M. M. (2021). Brain-behavior relationships in the perceptual decision-making process through cognitive processing stages. Neuropsychologia, 155, Article 107821.
https://doi.org/10.1016/j.neuropsychologia.2021.107821
Kim, D., Lee, C.-K., & Sirgy, M. J. (2016). Examining the differential impact of human crowding versus spatial crowding on visitor satisfaction at a festival. Journal of Travel & Tourism Marketing, 33(3), 293–312.
https://doi.org/10.1080/10548408.2015.1024914
Legrain, A., Eluru, N., & El-Geneidy, A. M. (2015). Am stressed, must travel: The relationship between mode choice and commuting stress. Transportation Research Part F: Traffic Psychology and Behaviour, 34, 141–151.
https://doi.org/10.1016/j.trf.2015.08.001
Li, K. K. F., Jarvis, S. A., & Minhas, F. (2021). Elementary effects analysis of factors controlling COVID-19 infections in computational simulation reveals the importance of social distancing and mask usage. Computers in Biology and Medicine, 134, Article 104369.
https://doi.org/10.1016/j.compbiomed.2021.104369
Liu, Y., Ouyang, Z., & Cheng, P. (2019). Predicting consumers’ adoption of electric vehicles during the city smog crisis: An application of the protective action decision model. Journal of Environmental Psychology, 64, 30–38.
https://doi.org/10.1016/j.jenvp.2019.04.013
MacIntyre, C. R., Nguyen, P.-Y., Chughtai, A. A., Trent, M., Gerber, B., Steinhofel, K., & Seale, H. (2021). Mask use, risk mitigation behaviours and pandemic fatigue during the COVID-19 pandemic in five cities in Australia, the UK and USA: A cross-sectional survey. International Journal of Infectious Diseases, 106, 199–207.
https://doi.org/10.1016/j.ijid.2021.03.056
Márquez, L., Alfonso A. J. V., & Poveda, J. C. (2019). In-vehicle crowding: Integrating tangible attributes, attitudes, and perceptions in a choice context between BRT and metro. Transportation Research Part A: Policy and Practice, 130, 452–465.
https://doi.org/10.1016/j.tra.2019.09.061
Mehta, R. (2013). Understanding perceived retail crowding: A critical review and research agenda. Journal of Retailing and Consumer Services, 20(6), 642–649.
https://doi.org/10.1016/j.jretconser.2013.06.002
Milne, R. A. (1976). A graphic approach to Rosenberg’s Affective-Cognitive Consistency Theory. Human Relations, 29(3), 273–285.
https://doi.org/10.1177/001872677602900306
Morosan, C. (2017). Information disclosure to biometric E-gates: The roles of perceived security, benefits, and emotions. Journal of Travel Research, 57(5), 644–657.
https://doi.org/10.1177/0047287517711256
Pagneux, E., Gísladóttir, G., & Jónsdóttir, S. (2011). Public perception of flood hazard and flood risk in Iceland: A case study in a watershed prone to ice-jam floods. Natural Hazards, 58(1), 269–287.
https://doi.org/10.1007/s11069-010-9665-8
Rice, S., & Winter, S. R. (2019). Do gender and age affect willingness to ride in driverless vehicles: If so, then why? Technology in Society, 58, Article 101145.
https://doi.org/10.1016/j.techsoc.2019.101145
Sam, E. F., Brijs, K., Daniels, S., Brijs, T., & Wets, G. (2019). Construction and validation of a public bus passenger safety scale. Transportation Research Part F: Traffic Psychology and Behaviour, 66, 47–62.
https://doi.org/10.1016/j.trf.2019.08.017
Shi, K., Lu, J., Fan, H., Jia, J., Song, Z., Li, W., … Hu, W. (2003). Rationality of 17 cities’ public perception of SARS and predictive model of psychological behavior. Chinese Science Bulletin, 48, 1297–1303.
https://doi.org/10.1007/BF03184166
Skinner, B. F. (1935). The generic nature of the concepts of stimulus and response. The Journal of General Psychology, 12(1), 40–65.
https://doi.org/10.1080/00221309.1935.9920087
Stanojević, D., Stanojević, P., Jovanović, D., & Lipovac, K. (2020). Impact of riders’ lifestyle on their risky behavior and road traffic accident risk. Journal of Transportation Safety & Security, 12(3), 400–418.
https://doi.org/10.1080/19439962.2018.1490367
Stokols, D. (1972). On the distinction between density and crowding: Some implications for future research. Psychological Review, 79(3), 275–277.
https://doi.org/10.1037/h0032706
Stokols, D. (1976). The experience of crowding in primary and secondary environments. Environment and Behavior, 8(1), 49–86.
https://doi.org/10.1177/001391657600800104
Stoop, J. A. (1997). Risk awareness and decision making in shipping. IFAC Proceedings Volumes, 30(8), 793–797.
https://doi.org/10.1016/S1474-6670(17)43919-X
Surry, J. (1969). Industrial accident research: A human engineering approach. Journal of Occupational Medicine, 11(9), 492–493. https://bit.ly/3pnc5Rx
Tirachini, A., Hurtubia, R., Dekker, T., & Daziano, R. A. (2017). Estimation of crowding discomfort in public transport: Results from Santiago de Chile. Transportation Research Part A: Policy and Practice, 103, 311–326.
https://doi.org/10.1016/j.tra.2017.06.008
Tolman, E. C. (1948). Cognitive maps in rats and men. Psychological Review, 55(4), 189–208.
https://doi.org/10.1037/h0061626
Tversky, A., & Kahneman, D. (1974). Judgment under uncertainty: Heuristics and biases: Biases in judgments reveal some heuristics of thinking under uncertainty. Science, 185(4157), 1124–1131.
https://doi.org/10.1126/science.185.4157.1124
Verhoeff, L. (2014). Choice and experience of the movement route. Transportation Research Procedia, 2, 675–680.
https://doi.org/10.1016/j.trpro.2014.09.073
Vicente, R., Ferreira, T. M., Maio, R., & Koch, H. (2014). Awareness, perception and communication of earthquake risk in Portugal: Public survey. Procedia Economics & Finance, 18, 271–278.
https://doi.org/10.1016/S2212-5671(14)00940-X
Wang, D., Yao, J., & Martin, B. A. S. (2021). The effects of crowdedness and safety measures on restaurant patronage choices and perceptions in the COVID-19 pandemic. International Journal of Hospitality Management, 95, Article 102910.
https://doi.org/10.1016/j.ijhm.2021.102910
Wang, X., Yuen, K. F., Shi, W., & Ma, F. (2020). The determinants of passengers’ safety behaviour on public transport. Journal of Transport & Health, 18, Article 100905.
https://doi.org/10.1016/j.jth.2020.100905
Witting, M., Filimon, S., & Kevork, S. (2021). Carry along or not? Decision-making on carrying standard avalanche safety gear among ski tourers in a German touring region. Safety Science, 143(5), Article 105406.
https://doi.org/10.1016/j.ssci.2021.105406
Xia, N., Xie, Q., Griffin, M. A., Ye, G., & Yuan, J. (2020). Antecedents of safety behavior in construction: A literature review and an integrated conceptual framework. Accident Analysis & Prevention, 148, Article 105834.
https://doi.org/10.1016/j.aap.2020.105834
Yin, J., Cheng, Y., Bi, Y., & Ni, Y. (2020). Tourists perceived crowding and destination attractiveness: The moderating effects of perceived risk and experience quality. Journal of Destination Marketing & Management, 18, Article 100489.
https://doi.org/10.1016/j.jdmm.2020.100489
Zhang, J., Xie, C., & Morrison, A. M. (2021). The effect of corporate social responsibility on hotel employee safety behavior during COVID-19: The moderation of belief restoration and negative emotions. Journal of Hospitality and Tourism Management, 46, 233–243.
https://doi.org/10.1016/j.jhtm.2020.12.011
Ajzen, I. (1991). The theory of planned behavior. Organizational Behavior and Human Decision Processes, 50(2), 179–211.
https://doi.org/10.1016/0749-5978(91)90020-T
Bandura, A. (1978). The self system in reciprocal determinism. American Psychologist, 33(4), 344–358.
https://doi.org/10.1037/0003-066X.33.4.344
Bargh, J. A., Chaiken, S., Govender, R., & Pratto, F. (1992). The generality of the automatic attitude activation effect. Journal of Personality and Social Psychology, 62(6), 893–912.
https://doi.org/10.1037/0022-3514.62.6.893
Cambon de Lavalette, B., Tijus, C., Poitrenaud, S., Leproux, C., Bergeron, J., & Thouez, J.-P. (2009). Pedestrian crossing decision-making: A situational and behavioral approach. Safety Science, 47(9), 1248–1253.
https://doi.org/10.1016/j.ssci.2009.03.016
Chang, Y.-H., & Liao, M.-Y. (2009). The effect of aviation safety education on passenger cabin safety awareness. Safety Science, 47(10), 1337–1345.
https://doi.org/10.1016/j.ssci.2009.02.001
Chen, Y., Wang, Y., Nie, Q., Zheng, J., & Ding, C. (2017). Modeling and an analysis of safety perception of online car-hailing passengers based on a structural equation model. Journal of Transport Information and Safety, 35(6), 85–92.
Chowdhury, S., & Ceder, A. (2016). Users’ willingness to ride an integrated public-transport service: A literature review. Transport Policy, 48, 183–195.
https://doi.org/10.1016/j.tranpol.2016.03.007
Cox, T., Houdmont, J., & Griffiths, A. (2006). Rail passenger crowding, stress, health and safety in Britain. Transportation Research Part A: Policy and Practice, 40(3), 244–258.
https://doi.org/10.1016/j.tra.2005.07.001
Ellis, A. (1991). The revised ABC’s of rational-emotive therapy (RET). Journal of Rational-Emotive and Cognitive-Behavior Therapy, 9, 139–172.
https://doi.org/10.1007/BF01061227
Fu, G., Zhao, Z., Hao, C., & Wu, Q. (2019). The accident path of coal mine gas explosion based on 24 model: A case study of the Ruizhiyuan gas explosion accident. Processes, 7(2), Article 73.
https://doi.org/10.3390/pr7020073
Hayes, A. F. (2013). Introduction to mediation, moderation, and conditional process analysis: A regression-based approach. Guilford Press.
Huang, W., Shuai, B., Zuo, B., Xu, Y., & Antwi, E. (2019). A systematic railway dangerous goods transportation system risk analysis approach: The 24 model. Journal of Loss Prevention in the Process Industries, 61, 94–103.
https://doi.org/10.1016/j.jlp.2019.05.021
Huang, Y., Yang, S., & Dai, J. M. (2021). Self- versus other-directed outcomes, Machiavellianism, and hypothetical distance in COVID-19 antipandemic messages. Social Behavior and Personality: An international journal, 49(3), Article e10109.
https://doi.org/10.2224/sbp.10109
Hui, M. K., & Bateson, J. E. G. (1991). Perceived control and the effects of crowding and consumer choice on the service experience. Journal of Consumer Research, 18(2), 174–184.
https://doi.org/10.1086/209250
Imani, E., Harati, A., Pourreza, H., & Goudarzi, M. M. (2021). Brain-behavior relationships in the perceptual decision-making process through cognitive processing stages. Neuropsychologia, 155, Article 107821.
https://doi.org/10.1016/j.neuropsychologia.2021.107821
Kim, D., Lee, C.-K., & Sirgy, M. J. (2016). Examining the differential impact of human crowding versus spatial crowding on visitor satisfaction at a festival. Journal of Travel & Tourism Marketing, 33(3), 293–312.
https://doi.org/10.1080/10548408.2015.1024914
Legrain, A., Eluru, N., & El-Geneidy, A. M. (2015). Am stressed, must travel: The relationship between mode choice and commuting stress. Transportation Research Part F: Traffic Psychology and Behaviour, 34, 141–151.
https://doi.org/10.1016/j.trf.2015.08.001
Li, K. K. F., Jarvis, S. A., & Minhas, F. (2021). Elementary effects analysis of factors controlling COVID-19 infections in computational simulation reveals the importance of social distancing and mask usage. Computers in Biology and Medicine, 134, Article 104369.
https://doi.org/10.1016/j.compbiomed.2021.104369
Liu, Y., Ouyang, Z., & Cheng, P. (2019). Predicting consumers’ adoption of electric vehicles during the city smog crisis: An application of the protective action decision model. Journal of Environmental Psychology, 64, 30–38.
https://doi.org/10.1016/j.jenvp.2019.04.013
MacIntyre, C. R., Nguyen, P.-Y., Chughtai, A. A., Trent, M., Gerber, B., Steinhofel, K., & Seale, H. (2021). Mask use, risk mitigation behaviours and pandemic fatigue during the COVID-19 pandemic in five cities in Australia, the UK and USA: A cross-sectional survey. International Journal of Infectious Diseases, 106, 199–207.
https://doi.org/10.1016/j.ijid.2021.03.056
Márquez, L., Alfonso A. J. V., & Poveda, J. C. (2019). In-vehicle crowding: Integrating tangible attributes, attitudes, and perceptions in a choice context between BRT and metro. Transportation Research Part A: Policy and Practice, 130, 452–465.
https://doi.org/10.1016/j.tra.2019.09.061
Mehta, R. (2013). Understanding perceived retail crowding: A critical review and research agenda. Journal of Retailing and Consumer Services, 20(6), 642–649.
https://doi.org/10.1016/j.jretconser.2013.06.002
Milne, R. A. (1976). A graphic approach to Rosenberg’s Affective-Cognitive Consistency Theory. Human Relations, 29(3), 273–285.
https://doi.org/10.1177/001872677602900306
Morosan, C. (2017). Information disclosure to biometric E-gates: The roles of perceived security, benefits, and emotions. Journal of Travel Research, 57(5), 644–657.
https://doi.org/10.1177/0047287517711256
Pagneux, E., Gísladóttir, G., & Jónsdóttir, S. (2011). Public perception of flood hazard and flood risk in Iceland: A case study in a watershed prone to ice-jam floods. Natural Hazards, 58(1), 269–287.
https://doi.org/10.1007/s11069-010-9665-8
Rice, S., & Winter, S. R. (2019). Do gender and age affect willingness to ride in driverless vehicles: If so, then why? Technology in Society, 58, Article 101145.
https://doi.org/10.1016/j.techsoc.2019.101145
Sam, E. F., Brijs, K., Daniels, S., Brijs, T., & Wets, G. (2019). Construction and validation of a public bus passenger safety scale. Transportation Research Part F: Traffic Psychology and Behaviour, 66, 47–62.
https://doi.org/10.1016/j.trf.2019.08.017
Shi, K., Lu, J., Fan, H., Jia, J., Song, Z., Li, W., … Hu, W. (2003). Rationality of 17 cities’ public perception of SARS and predictive model of psychological behavior. Chinese Science Bulletin, 48, 1297–1303.
https://doi.org/10.1007/BF03184166
Skinner, B. F. (1935). The generic nature of the concepts of stimulus and response. The Journal of General Psychology, 12(1), 40–65.
https://doi.org/10.1080/00221309.1935.9920087
Stanojević, D., Stanojević, P., Jovanović, D., & Lipovac, K. (2020). Impact of riders’ lifestyle on their risky behavior and road traffic accident risk. Journal of Transportation Safety & Security, 12(3), 400–418.
https://doi.org/10.1080/19439962.2018.1490367
Stokols, D. (1972). On the distinction between density and crowding: Some implications for future research. Psychological Review, 79(3), 275–277.
https://doi.org/10.1037/h0032706
Stokols, D. (1976). The experience of crowding in primary and secondary environments. Environment and Behavior, 8(1), 49–86.
https://doi.org/10.1177/001391657600800104
Stoop, J. A. (1997). Risk awareness and decision making in shipping. IFAC Proceedings Volumes, 30(8), 793–797.
https://doi.org/10.1016/S1474-6670(17)43919-X
Surry, J. (1969). Industrial accident research: A human engineering approach. Journal of Occupational Medicine, 11(9), 492–493. https://bit.ly/3pnc5Rx
Tirachini, A., Hurtubia, R., Dekker, T., & Daziano, R. A. (2017). Estimation of crowding discomfort in public transport: Results from Santiago de Chile. Transportation Research Part A: Policy and Practice, 103, 311–326.
https://doi.org/10.1016/j.tra.2017.06.008
Tolman, E. C. (1948). Cognitive maps in rats and men. Psychological Review, 55(4), 189–208.
https://doi.org/10.1037/h0061626
Tversky, A., & Kahneman, D. (1974). Judgment under uncertainty: Heuristics and biases: Biases in judgments reveal some heuristics of thinking under uncertainty. Science, 185(4157), 1124–1131.
https://doi.org/10.1126/science.185.4157.1124
Verhoeff, L. (2014). Choice and experience of the movement route. Transportation Research Procedia, 2, 675–680.
https://doi.org/10.1016/j.trpro.2014.09.073
Vicente, R., Ferreira, T. M., Maio, R., & Koch, H. (2014). Awareness, perception and communication of earthquake risk in Portugal: Public survey. Procedia Economics & Finance, 18, 271–278.
https://doi.org/10.1016/S2212-5671(14)00940-X
Wang, D., Yao, J., & Martin, B. A. S. (2021). The effects of crowdedness and safety measures on restaurant patronage choices and perceptions in the COVID-19 pandemic. International Journal of Hospitality Management, 95, Article 102910.
https://doi.org/10.1016/j.ijhm.2021.102910
Wang, X., Yuen, K. F., Shi, W., & Ma, F. (2020). The determinants of passengers’ safety behaviour on public transport. Journal of Transport & Health, 18, Article 100905.
https://doi.org/10.1016/j.jth.2020.100905
Witting, M., Filimon, S., & Kevork, S. (2021). Carry along or not? Decision-making on carrying standard avalanche safety gear among ski tourers in a German touring region. Safety Science, 143(5), Article 105406.
https://doi.org/10.1016/j.ssci.2021.105406
Xia, N., Xie, Q., Griffin, M. A., Ye, G., & Yuan, J. (2020). Antecedents of safety behavior in construction: A literature review and an integrated conceptual framework. Accident Analysis & Prevention, 148, Article 105834.
https://doi.org/10.1016/j.aap.2020.105834
Yin, J., Cheng, Y., Bi, Y., & Ni, Y. (2020). Tourists perceived crowding and destination attractiveness: The moderating effects of perceived risk and experience quality. Journal of Destination Marketing & Management, 18, Article 100489.
https://doi.org/10.1016/j.jdmm.2020.100489
Zhang, J., Xie, C., & Morrison, A. M. (2021). The effect of corporate social responsibility on hotel employee safety behavior during COVID-19: The moderation of belief restoration and negative emotions. Journal of Hospitality and Tourism Management, 46, 233–243.
https://doi.org/10.1016/j.jhtm.2020.12.011
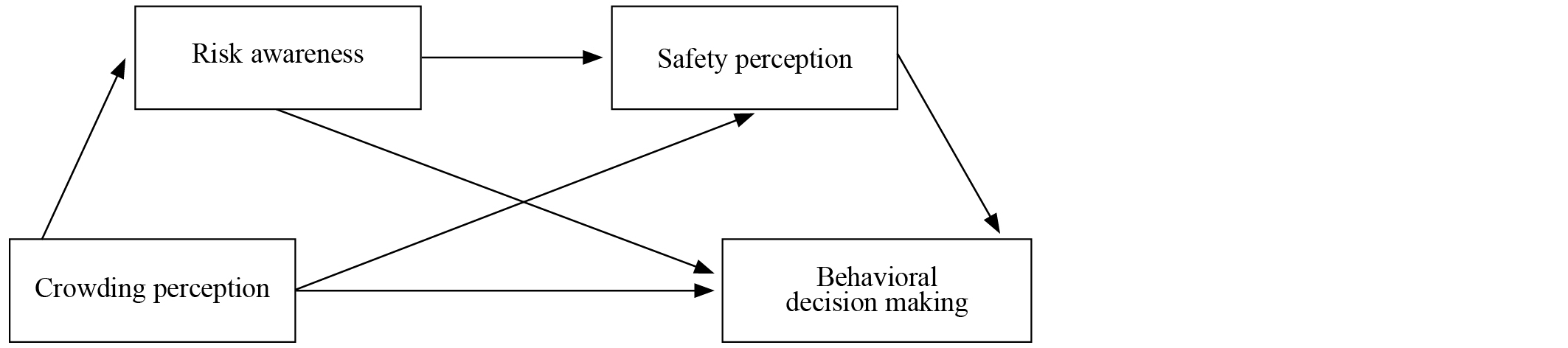
Figure 1. Theoretical Model
Table 1. Descriptive Statistical Results and Correlations for the Variables

Note. ** p < .01.
Table 2. Mediating Effects Test

Note. Boot = bootstrapped; CI = confidence interval; LL = lower limit; UL = upper limit.
This research was supported by the research project of The Research Institution of Beautiful China and Ecological Civilization of Shanghai Institute of Technology in 2020.
Mousheng Song, School of Economics and Management, Shanghai Institute of Technology, No. 100 Haiquan Road, Shanghai, People’s Republic of China, 201418. Email: [email protected]